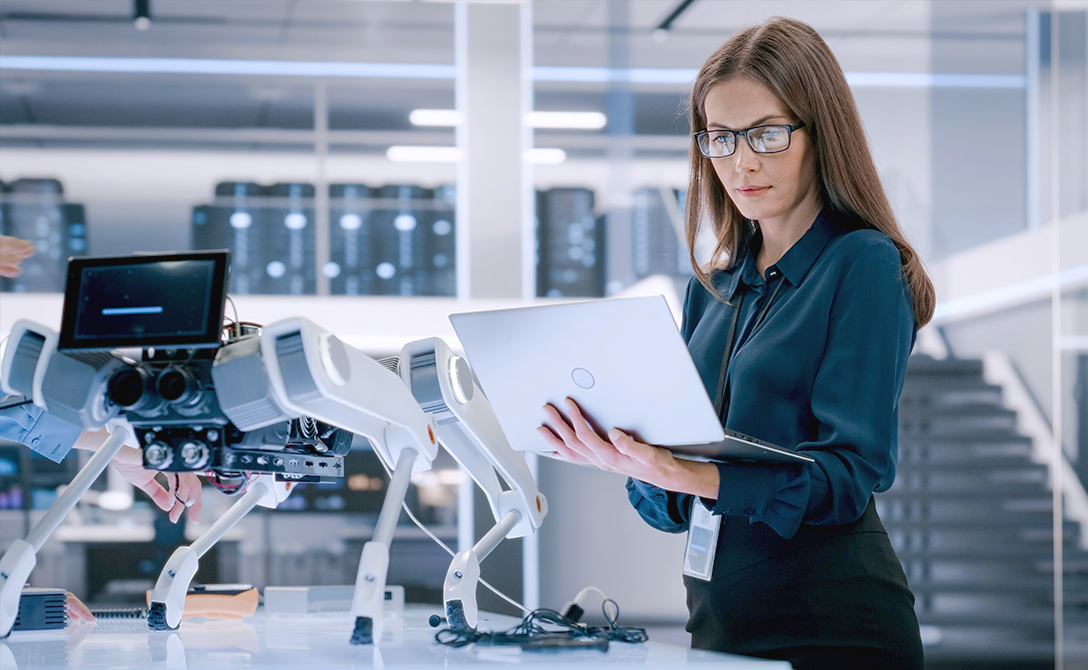
The recent past: From naive curiosity about transformer networks to a sublime plunge into the metamorphosis of LLMs
In 2017 the paper ‘Attention is all you need’ highlighted the advent of transformer networks. The world was then introduced to the Family of BERT models when Google introduced BERT: Pre-training of Deep Bidirectional Transformers for Language Understanding, where they took transfer learning to the next level. And the rest is a domino effect of competitors trying to dominate the Large Language Model (LLM) market, which provides promising output with a small task-specific dataset.
This development also included the collation of task-specific datasets in Natural Language Processing (NLP). SQuAD 1.0/2.0, TriviaQA, GraphQuestions, and MS MARCO are all well-known examples for:
- Question Answering, NER datasets like MalwareTextDB, re3d, Assembly, DataTurks (not functional anymore but was previously a good open source crowdsourcing NER labeling websites), etc.;
- Sentence Classification datasets on Kaggle were very famous (;
- Text Generation datasets like WikiQA (which includes specific models for this task - BART, GPT, and other GAN-based approaches).
The creativity
Researchers were more creative with transformer models. They even tried different approaches to optimizing the LLM, fine-tuning on a GPU using FNet: Fourier Transform techniques for replacing Self-Attention (a time-consuming method that was earlier used in language models).
To further explain:
Fine-tuning is an approach to transfer learning in which the weights of a pre-trained model are trained on new data. Fine-tuning can be done on a subset of the layers of a neural network or the entire network. (In general, we fine-tune the last few layers for LLM and make it work for a specific task).
Self-attention, sometimes called intra-attention, is an attention mechanism relating different positions of a single sequence to compute a sequence representation.
Self-attention was replaced by FNet, a layer-normalized ResNet architecture with multiple layers, each consisting of a Fourier mixing sublayer followed by a feed-forward sublayer. The Google team replaced the self-attention sublayer of each transformer encoder layer with a Fourier Transform sublayer. They applied 1D Fourier Transforms along both the sequence and hidden dimensions.
The result is a complex number that can be written as a real number multiplied by the imaginary unit (the number “i” in mathematics, which enables solving equations that do not have real number solutions). Only the result’s real number is kept, eliminating the need to modify the (nonlinear) feed-forward sublayers or output layers to handle complex numbers.
The result?
92% Accuracy, 7 Times Faster on GPUs
Another creative example is Vision Transformers (ViT). Transformers lack the inductive biases of Convolutional Neural Networks (CNNs); the transformer is, by design, permutation invariant. It cannot process grid-structured data, and we need sequences. Conversion of a non-sequential spatial signal to a sequence is required to implement ViT, which gives us the boost from transfer learning. The following steps are used to finetune a ViT:
- Split an image into patches
- Flatten the patches
- Produce lower-dimensional linear embeddings from the flattened patches
- Add positional embeddings
- Feed the sequence as an input to a standard transformer encoder
- Pretrain the model with image labels (fully supervised on a huge dataset)
- Finetune on the downstream dataset for image classification
Interest in replicating the human behavior
The human-like behavior of an Artificial Intelligence (AI) model has been a desired outcome for a long time. Before the AI winter, it was just a concept; after that, things started to become a reality slowly with transfer learning--from CNNs to LSTM/GRU, to Attention Mechanism, to transformer network and KG/GNN/beyond, and with the respective datasets like ImageNet/ImageNette, NLP datasets for QA/Sentence Classification/NER/Text Generation.
In addition to model architectures, research around how a neural network's simplest units could behave more human-like launched. For example,
- Oscillating Activation Functions like the Growing Cosine Unit and Family of Oscillating Activation Functions helped solve the XOR problem with a single neuron in image datasets, which boosted the performance and reduced the network size for any given architecture on standardized image datasets.
- Alternate Loss Functions, a frequently unappreciated hyperparameter in ANN models, can be tuned to significantly improve performance. Better performance can be achieved with the same dataset by using alternate loss functions that penalize training errors more harshly than cross-entropy loss. This paper introduces new loss functions that outperform cross-entropy on many computer vision and NLP tasks.
Interest in building an AI-powered Chatbot using RASA continues to grow; meanwhile, ChatGPT has gained millions of followers in just a few months.
By December 4, 2022, ChatGPT had over one million users. It reached over 100 million users in January 2023, making it the fastest-growing consumer application. CNBC noted on December 15, 2022, that the service "still goes down from time to time", and the free service is throttled.
ChatGPT and its use-cases
ChatGPT is a large language model developed by OpenAI that uses deep learning techniques to generate human-like language responses. At its core, ChatGPT is a neural network trained on a massive amount of text data, enabling it to understand language and coherent responses to user input. One of the key features of ChatGPT is its ability to generate contextually relevant and semantically accurate responses. This is achieved through unsupervised learning, where the model is trained on a large corpus of text without explicit guidance on what constitutes correct or incorrect responses.
The training data for ChatGPT consists of a diverse range of sources, including books, articles, and websites. The model learns from this data using a transformer network technique, which allows it to capture the long-term dependencies and complex relationships between words in a sentence. One of the benefits of using a transformer-based architecture like ChatGPT is that it allows for parallel processing, making it possible to train models that can handle large amounts of data efficiently. This is important because the data required to train large language models like ChatGPT is immense, with billions of text samples needed to achieve state-of-the-art performance.
In addition to its impressive language generation capabilities, ChatGPT has several other features that make it a powerful tool for natural language processing tasks. For example, it can be fine-tuned for tasks such as sentiment analysis, question answering, and summarization, making it a versatile tool for various applications. To fine-tune ChatGPT for specific tasks, additional training data is used to retrain the model on the particular task at hand. This process helps the model learn the nuances of the task and enables it to produce more accurate and relevant responses. One of the most impressive aspects of ChatGPT is its ability to understand and generate natural language responses that are virtually indistinguishable from those of a human. This has significant implications for various industries, from customer service and support to education and entertainment.
As an AI language model, ChatGPT also has the potential to revolutionize the field of natural language processing, making it possible to automate tasks that humans previously performed. This could lead to significant productivity gains and cost savings for businesses across various industries. However, like any AI technology, ChatGPT also has its limitations; for example, it may struggle to understand sarcasm or humor and generate biased or inappropriate responses in certain contexts. Additionally, the ethical implications of using AI language models like ChatGPT are still being debated, with concerns around privacy, data security, and algorithmic bias.
The Next Level? GPT 4.0 and Multimodal Learning
ChatGPT is correct about its future!
Various new NLP techniques like multimodal learning are the future, and we are already observing that in GPT 4.0.
A fun fact: Google has already made models like Palm-e, Calm, LamDA, and, recently, Bard to compete with OpenAI’s language modeling capabilities. Are we observing a similar 2018 era that happened with the creation of BERT?
Answer to the questions and the way forward.
The culprit is already known, leading many industries to incorporate ChatGPT through transfer learning and fine-tuning!
Let’s boil it down to basics in a few simple steps and examples:
- Clarity on business requirements - categorize file names into the department, file type, and use case categories. Each category has a list of items under which it can be placed.
- Clarity on minimal task-specific data - nearly 10k records should suffice as some files could have a similar context, and we need a balanced dataset to train for a text classification task (in this case, it is a sentence classification task).
- Clarity on Fine-tuning Process:
- The labeled data creation for the desired text classification (manually by your team, or could use data labeling services like CloudFactory or Mechanical Turk by Amazon)
- Fine-tuning ChatGPT or relevant LLM model - Every model has a different pipeline. Exploring Huggingface, blogs, and demos from Analytics Vidhya could help.
- Clarity on infrastructure to use and deploy the solution: The services that provide you with a good virtual machine (VM) with low cost and efficient graphics processing unit (GPU) are your go-to cloud-service providers. Databricks could be explored for multi-purpose GPU instances, providing the Azure environment to develop an end-to-end solution.
ChatGPT will be used not only in customer service, education, and entertainment but also in marketing to generate messages on the spot and effectively have a playbook for marketers to boost their reach in spot campaigns since they don’t have to spend much time brainstorming the idea, and ChatGPT prompting could be the next upcoming skillset in the job market.
It is helpful to understand the underlying algorithms to comprehend the new world that will come with GPT 4.0, which is multimodal. To put things in perspective for consultants, deeper concept understanding is also required when clients become smarter with next-generation leaders in place.
In simple terms, GPT-4 is multimodal, meaning it can generate content from image and text prompts. "GPT-4 can solve difficult problems with greater accuracy, thanks to its broader general knowledge and problem-solving abilities," according to the OpenAI website.
An effective campaign strategy design could depend on how well the marketer understands the underlying functionality of GPT 4.0 in the image, text, voice, video, and more, making it essential for marketers to have a good research background in machine learning to compete in the new AI-driven market.
At the same time, governance around text and image generation (even image generation using artists’ open-source image data for services like Midjourney, Jaspor, Fotor, Zapier, etc., which use GAN, stable diffusion, and enhanced models) is being debated.
Humans are indeed making a replica of themselves today, and it is essential to understand the basics of technology, at a minimum, to adapt to the new world and its requirements. ChatGPT and GPT 4.0 are impressive AI language models that have the potential to transform the way we interact with machines and automate a wide range of natural language processing tasks in a variety of applications across industries. Furthermore, its ability to generate human-like language responses and understand complex language structures make it a powerful tool for many applications, but its limitations and ethical implications must be carefully considered and addressed.
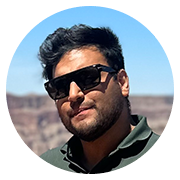
AUTHOR - FOLLOW
Shubham Bharadwaj
Associate Manager, Data and AI
Topic Tags