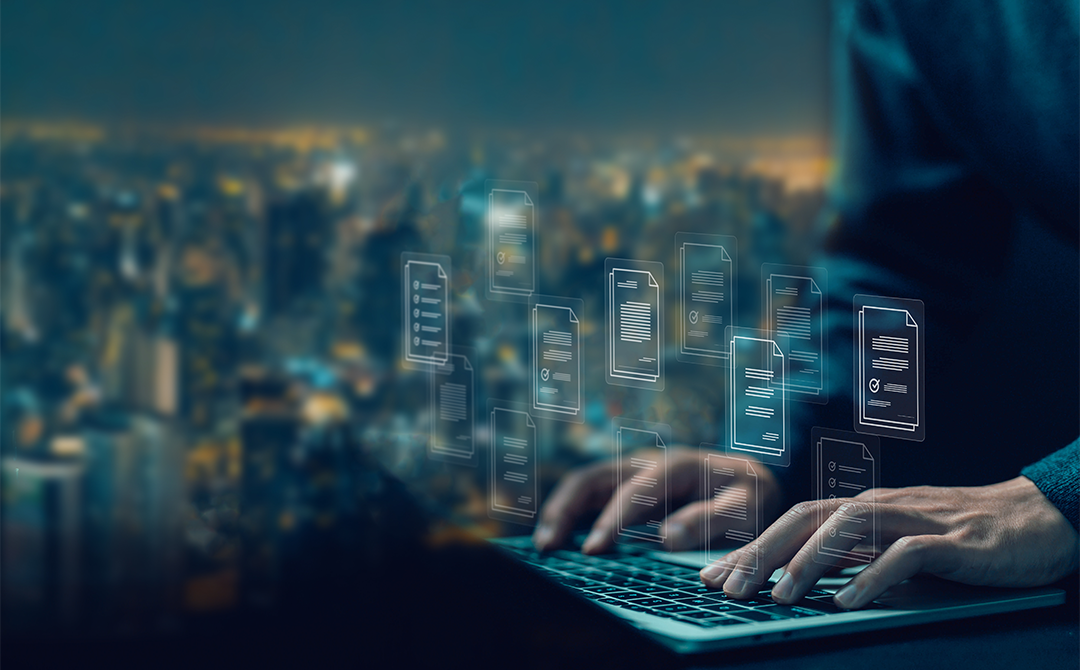
Modern organizations are inundated with diverse and complex data, making data management a formidable task. From inventory and sales data to vendor contracts and social media sentiment, every piece of information must be in a single repository, stored securely, and ready for daily activities, regular reporting, and advanced analytics, including AI. The evolution of data architectures - from data pools to data warehouses and data lakes, and now the data lakehouse – traces the journey of technologists as they race to create a robust, unified data platform that can help enterprises draw the maximum benefit from data that is growing in volume and variety.
Source: BCG
This post delves into the advantages of deploying a data lakehouse over data lakes and data warehouses for future-proof data management as data volumes explode.
The Data Management Journey
Data Warehouses
Data Warehouses have served the business intelligence requirements of enterprises for over three decades. A data warehouse gathers raw data from multiple sources and stores it in a single, central repository. It uses the ETL (Extract, Transform, and Load) process to load the data to its destination. It stores only structured data that is cleansed, standardized, and tailored for specific purposes across daily tasks and advanced analytics. But, warehouses often have an on-premises component.
Consequently, organizations have to expend money and effort to increase storage as data grows. Since warehouses restrict data structures, they cannot support comprehensive AI/ML efforts. This constellation of reasons makes the architecture increasingly unviable today.
Data Lakes
The escalating data storage needs and the advent of live data streaming mandated a new data storage architecture for enterprises - the data lake. A data lake, typically hosted in the cloud, accommodates massive data of all types, including audio, visual, and text, with no schema enforcement. It is cost-effective, scales easily, and is ideal for AI initiatives. However, the lack of rigorous data structures hampers transaction query engines and establishing relationships between data sets. This makes it challenging for data engineers to navigate the repository, and its efficiency decreases with increasing data workloads. In fact, the relatively relaxed data governance may lead to the lake becoming a "swamp, " obscuring valuable insights.
Organizations began to depend on warehouses and lakes to overcome the challenge of deriving value from every kind of data while also powering high-powered analytics and AI engines. This means double the spends– likely even more.
The Data Lakehouse – The Need of the Hour
Leading ecosystem players, cognizant of enterprises' daily struggles, responded with an innovation that simplifies the complexities of modern data management.
A data lakehouse is a data management platform that combines the most productive capabilities of data lakes and data warehouses so enterprises have a cost-effective, modern, analytics-compatible unified data platform. |
To provide modern organizations with much-desired agile, optimal-cost data storage, a data lakehouse leverages the cloud to store data of all types, including structured, semi-structured, and unstructured. It also has a robust governance layer and is amenable to hosting friendly interfaces. It supports programming languages like Python and high-performance SQL. Unlike its predecessor architectures, it effortlessly decouples storage and compute, giving you more effortless scalability. Hence, it can support BAU data processing and facilitate advanced data analytics, including AI/ML workloads and gen AI, giving you far better ROI.
Source: Databricks
More than 9000 organizations - including over 50% of the Fortune 500 use the pioneering Databricks Data Lakehouse platform to unify their data, analytics, and AI. |
Why are more and more enterprises moving to the data lakehouse?
It offers ACID compliance for database transactions. ACID is a robust framework that ensures the trustworthiness and integrity of data at any scale –a non-negotiable as enterprises digitize rapidly.
Atomicity: All operations on data are carried out as a single task. This ensures operations are either performed completely or left untouched, avoiding data loss or corruption.
Consistency: Maintains the data consistently from when the transaction begins until it ends.
Isolation: Ongoing transactions are invisible to others until completed. Allows concurrent transactions to be serialized.
Durability: Transaction-induced changes remain unchanged even in case of system failures. Ensures data consistency even as multiple users read and write data simultaneously.
While data warehouses are ACID compliant, they do not provide the flexibility of data structures modern analytics needs. Meanwhile, data lakes are not ACID compliant.
Data Lakehouse Vs. Data Lake Vs. Data Warehouse – A Round-up
Data Lakehouse | Data Warehouse | Data Lake |
On the cloud | Often on-prem | Mostly on the cloud |
Accommodates structured, semi-structured, and unstructured data | Accommodates structured data | Accommodates structured, semi-structured, and unstructured data |
Mid ETL needs | High ETL needs | Low ETL needs |
Robust governance layer and ACID compliant | Robust governance layer and ACID compliant | Weak governance |
Decouples storage and compute unlocking speed and scalability | Does not decouple store and compute | Does not decouple store and compute |
Can underpin daily activities, advanced analytics including AI/ML and gen AI at scale | Can underpin daily activities, advanced analytics, and some AI/ML | Can support AI/ML |
The Business Benefits of a Data Lakehouse
Here is how the blend of ACID compliance, flexibility, and simplicity helps enterprises use data, analytics, and AI to beat the competition
Simplified architecture optimizes data management and powers analytics. : A data lakehouse offers a simplified single data repository that can underpin the entire data management lifecycle. This eliminates the need for data migration, duplication, and transfers, which drive down ETL needs. The cloud ensures the fastest resolution of sync issues, reducing the formation of complex data pipelines. You can implement governance more easily, and connect many tools directly to the lakehouse to support ML, AI, data analytics, and data science workloads from the same repository to support diverse use cases throughout your organization.
Greater agility: The lower ETL requirements than a warehouse, the greater structure than a lake, and the capability to support diverse data offered by a data lakehouse will empower the company to respond emphatically to real-time shifts. Consider a pharma company that has to ramp up an entire production line and distribution for a specific medication during a global health crisis. From supercharging supplier networks to monitoring global body websites to understand where infection rates are soaring and where medication is needed the most, a lakehouse will help consolidate information and insights rapidly.
Databricks' Data Lakehouse is central to the Shell.ai Platform, which helps Shell use its complete historic data set to run 10,000+ inventory simulations across all its parts and facilities. Shell's inventory prediction models now run in a few hours rather than days, significantly improving stocking practices and driving significant savings annually. Source: Databricks |
Unlocking collaborative intelligence: With all the data now in one place and accessible to technical and business users through conventional BI tools and advanced analytics and AI/ML workflows, teams will no longer work in siloes. Every innovation or improvement will start from the collective knowledge of the entire organization. For instance, data scientists, sales, and supply chain teams can come together to create a global inventory strategy much faster than if the former had to toggle between a lake and a warehouse to prepare reports for the latter two.
Take Charge: Streamline your Data, Analytics, and AI with a Unified Platform
Convert a two or multi-tier architecture into a one-stop, single-tier, easily scalable data lakehouse architecture solution for end-to-end data quality management and analytics success. Technological advancement will seamlessly augment the existing capabilities of the lakehouse in the long run, making your business future-proof with data management and analytics that are at the bleeding edge.
Partner with elite data management and last-mile analytics and AI provider to unlock the full potential of your data.
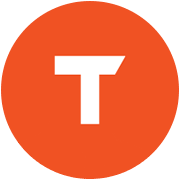
AUTHOR - FOLLOW
Editorial Team
Tredence
Topic Tags