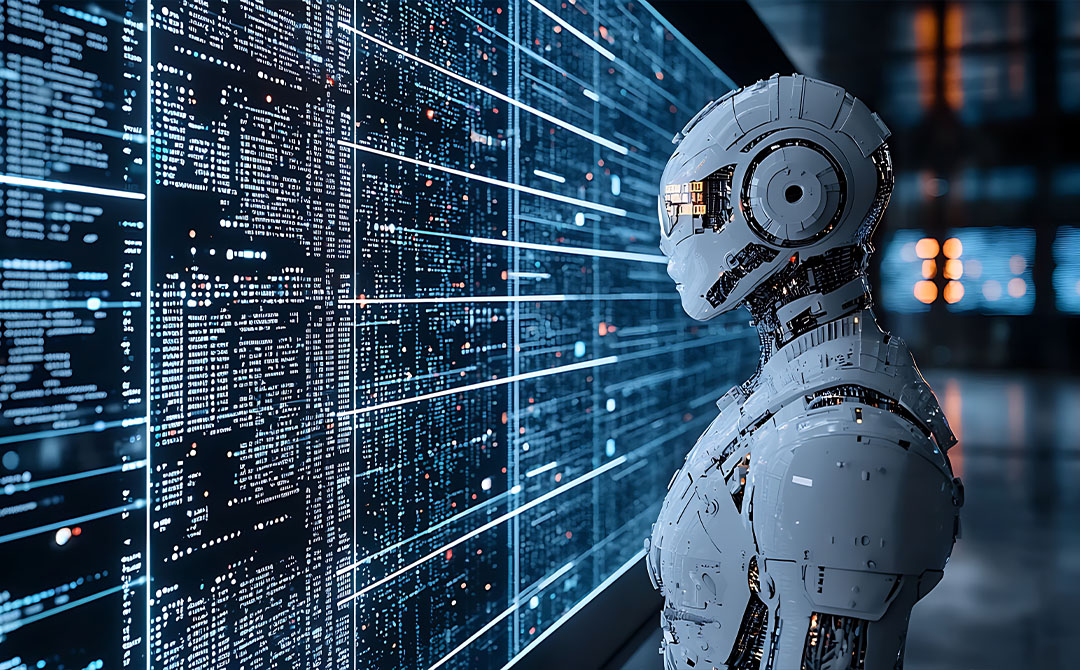
As AI becomes mainstream, the demand for data science and machine learning has surged, driven by an explosion in data, advances in AI research, and increased investments in AI technology. Tools like OpenAI’s ChatGPT and Anthropic’s Perplexity AI have further accelerated adoption, showcasing the potential of AI in transforming business innovation and challenging traditional search capabilities.
The terms “data science” and “machine learning” are often used interchangeably; however, they differ in essence. This article explores data science vs machine learning, highlighting their differences, similarities, and key applications.
What is Data Science?
Data science refers to the extraction of insights from an organization's internal and external data. It includes data collection, data cleaning and preprocessing, exploratory data analysis (EDA), model building and statistical analysis, data visualization and reporting, and finally, deployment and maintenance.
Data science integrates various disciplines, including statistics, computer science, and data engineering, to clean raw data, unify and harmonize it, and extract actionable insights.
What is Machine Learning?
Machine learning is a subset of AI that focuses on developing systems capable of self-learning and improving continuously without being programmed explicitly. It uses statistical models and algorithms to read patterns, identify them, and make decisions or predictions based on them.
Key components of machine learning include algorithms, training data, and model evaluations.
Is Machine Learning Part of Data Science?
Although the two terms may be used interchangeably, machine learning and data science are distinct fields with their own applications and focus areas.
Machine learning is considered a subset of data science, which encompasses a number of tasks, from data collection to visualization. Data scientists rely on various techniques, such as statistical modeling and business analytics, to make data-driven decisions.
Machine learning is a technique designed for data analysis and prediction. However, the capability to continuously learn and reduce or eliminate the need for explicit human intervention distinguishes machine learning from data science.
For example, PayPal and Stripe use machine learning models, including Random Forest and Gradient Boosting, to automatically detect fraudulent transactions. Analysts train these models on large volumes of data to identify patterns, such as geographic trends and anomalous spending behaviors (Source: PayPal, Stripe).
Netflix's Machine Learning Platform (MLP), powered by Metaflow, integrates tools and infrastructure to support diverse ML/AI use cases across data, compute, orchestration, and deployment layers, enabling scalable and production-ready ML workflows. Key integrations include Fast Data for efficient data handling, Titus for scalable compute, Maestro for orchestration, and Metaflow Hosting for API-based deployments, all designed to empower teams to build, deploy, and maintain complex ML systems seamlessly. (Source: Netflix).
The focus on data as the foundational element is a key similarity between data science and machine learning. The reliance on data extends beyond the input level to encompass the entire ecosystem.
Both data science and machine learning focus on data processing. Organizations often encounter unstructured data across various sources and formats, including APIs, sensors, CRMs, POS, emails, and transcripts. Duplicity and missing values, often leading to incorrect insights, are common issues with unstructured data.
Data science is a broader discipline, with a key focus on extracting insights from voluminous raw data, while machine learning is a tool within this broad discipline. Both disciplines rely significantly on ‘data as the foundational model.
Data Science vs Machine Learning: Key Differences
Data science is all about solving problems with the help of data. For example, “Why did sales drop last quarter?” Or “What type of customers are likely to buy this product?” Data science is concerned with the “what” and “why.”
Machine learning, on the other hand, focuses on the how. It enables data-driven solutions by identifying patterns, building predictive models, and automating decision-making processes. For instance, machine learning answers how to forecast customer churn, how to optimize pricing to boost sales, or how to classify customers based on their behavior.
Netflix, the streaming giant, relies significantly on data science to understand the nuances and subtleties of its audience. It analyzes vast amounts of user data—what shows people watch, how long they watch, and when they usually drop off.
Through data science, Netflix may discover that users in urban centers tend to watch shows with shorter episode durations or customers in the suburbs are more likely to binge-watch. This information gives Netflix insights into what future content it should acquire or how it should promote its shows.
Once these insights are derived, machine learning automates the process. Netflix’s recommendation engine uses collaborative filtering and neural networks to eliminate the need for constant human intervention in acting on insights. These techniques automatically suggest shows based on a user's viewing history.
Data Science vs Machine Learning in a Nutshell
Aspect |
Data Science |
Machine Learning |
Focus |
Understanding why users in urban areas prefer shorter-duration episodes. |
Automating the recommendation system to show users shorter episodes in urban areas. |
Objective |
Translating insights into a business strategy. |
Ensuring the system executes recommendations without human intervention every time. |
Nature |
Analytical and strategic. |
Automated and operational. |
Example Context |
Analyzing user preferences to derive actionable insights. |
Using algorithms to recommend shorter episodes at the right time. |
The key difference between data science and machine learning lies in their roles: data science focuses on understanding "what" and "why" through analysis and insights. In contrast, machine learning operationalizes these insights by automating predictions and actions.
Together, they complement each other, with data science driving strategic decisions and machine learning ensuring seamless execution at scale.
Applications of Data Science
Many end-users and industries leverage data science owing to its transformative impact on decision-making.
Let us take a look at how data science is being used across key sectors:
Healthcare and Lifesciences
Traditionally, the healthcare sector has been sluggish in accepting ‘digital transformation.’ However, the rise of AI has encouraged the healthcare and life sciences industry to explore areas that could benefit from this innovation.
In a survey conducted in Q1 2024, 70 percent of respondents mentioned they had already implemented AI capabilities or were in the process of doing so (Source: McKinsey).
Here are some key areas where the healthcare and life sciences industry is applying data science:
1. Disease Diagnosis and Risk Analysis
Data science enables healthcare professionals to analyze vast patient datasets, helping diagnose diseases at earlier stages.
IBM Watson Health uses data science to analyze millions of medical histories and journal articles to help doctors diagnose serious diseases such as cancer (Source: The New York Times).
This includes predictive analytics, which helps identify patients at risk of serious disorders at an early stage, giving healthcare providers valuable time to implement medical intervention.
2. Epidemiology and Public Health
Management of public health data is a challenge for organizations. According to the World Economic Forum, 97 percent of hospital data is unused (Source: World Economic Forum).
This unused data is a cause for concern, especially during epidemics and pandemics. In these situations, governments must quickly estimate and predict how many people will require urgent care or hospitalization. Based on these predictions, temporary or makeshift hospitals can be set up quickly to accommodate the patient surge.
Data science-enabled models helped governments understand epidemiology during the COVID-19 pandemic.
Johns Hopkins University created real-time dashboards with data science tools for governments and healthcare organizations during the pandemic. Using global databases, researchers identified hotspot areas and optimized resource allocation to minimize the effects of the pandemic (Source: John Hopkins Center for Communications Program).
3. Resource Management and Operational Efficiency
Hospitals and health facilities use data science to optimize resource distribution and simplify operations. Predictive analytics tools help predict patient admission rates, assisting hospitals in better managing staff, equipment, and bed capacity.
Cleveland Clinic uses data-driven models to predict which patients are more likely to be readmitted. Based on robust data analytics methodologies, this analysis considers several factors, including socio-economic conditions, patient demographics, and disease categories (Source: Cleveland Clinic).
4. Drug Discovery and Development
Data science accelerates drug discovery and clinical trials in the pharmaceutical industry. Novartis and Pfizer extensively use data-driven approaches to discover potential drug candidates and assess their efficiency.
Pfizer leveraged data analytics to accelerate the production of the COVID-19 vaccine. It integrated vast amounts of data from clinical trials, thus saving time and cost for getting the new drug to the market in a time of urgency (Source: Pfizer).
5. Customized Patient Care and Medication Adherence
Data science allows patient care to be customized by examining health records, genetic data, and lifestyle. Adhering to medications is a key challenge for healthcare providers, as missed doses not only delay recovery but can prove to be life-threatening in certain cases.
Data science enables healthcare providers to develop effective customized patient protocols by analyzing genetic, lifestyle, and environmental data.
For instance, Oncora Medical, a digital health company, uses data science extensively in its patient care platform, providing oncologists with crucial data in formulating personalized treatment plans (Source: Oncora Digital).
Finance
The finance industry has been one of the early adopters of data science. By April 2023, 85 percent of banks had already adopted some form of AI in their operations (Source: New York Institute of Technology).
Fraud detection and prevention is one of the most discussed use cases, but let us also explore other applications:
1. Fraud Detection and Prevention
Banks and financial institutions increasingly rely on data science to flag suspicious and fraudulent activities. The global fraud detection and prevention market will likely reach $252 billion by 2032, expanding at a CAGR of 24.3% (Source: Allied Market Research).
JPMorgan Chase uses data science tools to monitor millions of transactions in real time. These models alert human reviewers to potential fraud based on anomalies such as the use of different IPs or sudden changes in location.
Predictive analytics models are built on data science, and past events are analyzed to predict future risks and ensure better cybersecurity (Source: American Banker).
2. Credit Scoring and Risk Management
Data science enables financial institutions to establish creditworthiness and mitigate risk. By analyzing datasets related to income, spending habits, and loan histories, banks and financial institutions can build accurate credit scores for individuals or businesses.
FICO, one of the leading credit scoring companies, uses advanced data science models to determine lending risks. As with most large organizations, FICO manages vast amounts of data.
This data is not always in structured form, which further amplifies the data management challenge. FICO uses advanced analytical models, including, but not limited to, neural networks, random forests, and stochastic gradient boosting, to make accurate lending decisions (Source: FICO).
Other investment firms use risk analysis algorithms to predict market volatility, which helps portfolio managers make data-driven decisions to protect investments.
3. Algorithmic Trading
Data science is changing the status quo in traditional stock market trading through algorithmic trading systems. These advanced systems scan vast volumes of historical and real-time market data to find patterns in executing trades at the best prices.
QuantConnect’s trading algorithms are based on data decisions in trading instead of human evaluations and recommendations. Data science-enabled models are particularly efficient in high-frequency trading, which requires rapid decision-making owing to micro-movements in stock prices (Source: QuantConnect).
4. Customer Segmentation and Personalization
Data science enables financial institutions to segment customers based on behavior, preference, and demographics. Precise and targeted customer segmentation allows organizations to offer customized services to every customer.
American Express uses targeted customer segmentation to offer credit card recommendations and rewards. The bank analyzes spending patterns through advanced data science models to predict customer needs for loans, insurance, or investment plans (Source: American Express).
Retail and E-commerce
The retail and e-commerce industry relies significantly on data science in its daily operations. As per Accenture, generative AI has the potential to impact 50 percent of all working hours across retail over the next five years (Source: Accenture).
From sentiment analysis to pricing optimization, let's explore how data science is becoming the foundation for AI-driven transformation in retail.
1. Demand Forecasting and Inventory Management
Data science and data modernization enable retailers to forecast demand and manage their inventory effectively. For instance, Walmart’s proprietary data analytics platform processes millions of customer transactions daily.
At the onset of Hurricane Sandy, Walmart noticed that people were stocking up on flashlights and strawberry Pop-Tarts. As a result, the company prepared for future storms by replenishing these items in the affected areas.
This precise demand forecasting technique enables companies such as Walmart to increase stocks at the right time and decrease overstocking during periods of muted demand (Source: Walmart).
2. Recommendation and Customer Engagement
Amazon's recommendation system is one of the best examples of effective data science usage in retail. The global e-commerce giant has successfully delivered the right recommendations to its customers. It analyzes over 100 million profiles through collaborative filtering and item-based algorithms.
If a customer looks at headphones, Amazon recommends other accessories, such as headphone cases or chargers. This targeted approach is responsible for approximately 35% of all sales on Amazon (Source: Amazon Science).
Similarly, Starbucks’ rewards app sends personal offers based on purchase history and preferences to drive customer engagement and loyalty. The company has 17 million users on its mobile app, with access to their preferences such as favorite drink, usual times of ordering, and spending patterns.
By analyzing this information, Starbucks can create personalized promotional campaigns and rewards for its customers to increase the probability of additional sales (Source: Harvard.edu).
3. Pricing Optimization
Data science enables retailers to achieve optimal pricing by implementing a dynamic pricing system that replaces the traditional static pricing model.
Uber uses dynamic pricing, which measures parameters such as trip demand, weather, and traffic, in real time. It raises prices during the peak season, especially during rains, to maintain the balance between supply and demand. This pricing mechanism is also being adopted by retailers. (Source: Uber Blog).
Manufacturing
Data science has become a cornerstone of innovation in the manufacturing industry, providing tools and methodologies to optimize production, improve product quality, and enhance operational efficiency.
Below are detailed use cases of data science in the manufacturing sector:
1. Predictive Maintenance
Sudden equipment failures, which stall projects, have been hampering the manufacturing sector. As per a study by Senseye, large manufacturing companies lose an average of 27 hours per month in downtime (Source: Siemens).
Advances in data science and AI are emerging as pragmatic solutions to predict downtimes, helping manufacturers adopt a pre-emptive rather than reactive approach.
Predix, General Electric's (GE) IIoT platform, collects data from sensors installed on machinery to monitor variables such as vibration, temperature, and pressure. By identifying key patterns, GE accurately predicts the right maintenance time.
This data-driven predictive model has helped GE's Aviation division reduce its maintenance costs by 10 percent to 20 percent (Source: GE Aerospace).
2. Quality Control
Data science enables the manufacturing sector to enhance quality control, leading to fewer product recalls and a stronger brand reputation. Boeing employs predictive analytics to monitor and improve its manufacturing processes.
By analyzing historical production data and real-time sensor readings, Boeing can identify patterns that may indicate potential defects or quality issues before they occur (Source: Quality Magazine).
3. Supply Chain Optimization
Supply chain optimization is one of the most impactful data science applications in manufacturing. It enables companies to enhance efficiency, reduce costs, and respond dynamically to market changes.
By leveraging advanced analytics and machine learning, manufacturers can gain actionable insights into every aspect of their supply chain, from procurement to delivery.
Toyota uses data analytics to predict demand and optimize its inventory and logistics supply chain. The carmaker procures raw materials based on cues from its predictive analytics model, which considers historical sales data, market trends, and supplier performance.
During the 2021 semiconductor shortage, Toyota's data-driven supply chain model could better navigate supply chain production disruptions than its competitors, owing to its robust predictive insights (Source: Toyota Europe).
The adoption of data science as a discipline has still not reached its full potential in several industries. However, more industries are likely to adopt data science in the near future, given the rising availability of a skilled workforce and real-world examples demonstrating its impact on revenues and operations.
Applications of Machine Learning
The adoption of machine learning is rising in various industries, owing to advancements in the development of new models and decreases in initial starting costs. The machine learning market is projected to expand at a 34.8% CAGR by the end of 2030 (Source: Grand View Research).
Let us take a look at some of the applications of machine learning:
Healthcare & Lifesciences
Machine learning has quickly gained prominence in the healthcare and life sciences sector. Its adoption positively impacts operations and patient care.
Machine learning has transformed the healthcare industry in every sense, from managing and extracting insights from vast electronic health records (EHRs) to recommending personalized care based on patient data.
Let us explore some prominent applications of machine learning in the healthcare and life sciences industry:
1. Advanced Medical Imaging
Machine learning is driving innovation in medical imaging, particularly in detecting complex patterns in images that the human eye may miss. Machine learning models, especially convolutional neural networks (CNNs), are designed to analyze complex datasets, enabling early and accurate detection of diseases such as cancer and neurological disorders.
Zebra Medical Vision, an AI imaging startup, uses machine learning algorithms to analyze CT scans, X-rays, and MRIs, detecting conditions such as osteoporosis, liver disease, and early-stage breast cancer.
Unlike traditional medical imaging procedures, Zebra's AI processes thousands of images in seconds and prioritizes crucial cases for radiologists. This reduces human oversights and accelerates diagnoses (Source: Harvard Digital Innovation and Transformation).
2. Clinical Documentation
Maintaining accurate clinical documentation consumes a significant amount of time in the daily schedules of healthcare providers. Automation using natural language processing (NLP) has emerged as a practical solution to this long-standing challenge, enabling automated transcription and analysis of clinical notes.
Dragon Medical One, a machine learning-based voice recognition software developed by Nuance Communications, converts spoken medical notes into structured text.
The system understands medical jargon and integrates seamlessly with electronic health records, reducing documentation time by over 50 percent. These applications enhance operational efficiency while minimizing documentation errors (Source: Nuance).
3. Patient Risk Stratification
Machine learning can stratify at-risk patients, reducing emergency room (ER) visits and providing timely interventions for chronic conditions such as diabetes, hypertension, and heart diseases. Advanced machine learning models consider patient history, real-time vitals, and lifestyle factors to predict future health outcomes.
Omada Health uses machine learning to scan data from wearables and patient records. This enables the organization to identify people at higher risk of developing type 2 diabetes in later stages of life. Based on these insights, Omada Health designs customized digital health plans that mitigate the risk of developing type 2 diabetes (Source: Omada Health).
Similarly, Kaiser Permanente uses machine learning models to predict the risk of a heart attack while scanning EHRs. It then immediately recommends preventive care for those at risk. This real-time risk stratification is unique, as it is dynamic and continuously learns and updates predictions based on new data (Source: Kaiser Permanente).
Finance
Machine learning transforms how financial institutions manage risk, detect fraud, and optimize investment strategies.
By analyzing vast datasets, machine learning algorithms can uncover patterns, predict market trends, and enable real-time decision-making, thus enhancing operational efficiency and customer experience.
Let us discuss certain key applications of machine learning in the finance industry:
1. Market Insights through Sentiment Analysis
Data science aggregates data into trends, while machine learning enables organizations to analyze real-time sentiments by processing information from news articles, earnings calls, analyst briefings, company announcements, and social media posts. This helps financial institutions understand the market perspective and its impact on stock prices.
Bloomberg uses NLP to analyze investor sentiment toward a particular company or stock. Its machine learning models consider the tone, term, frequency, and previous history to predict the future direction of the market (Source: PyQuant Newsletter).
2. Portfolio Optimization
Reinforcement learning (RL) is a machine learning technique that enables an artificial intelligence agent to learn how to make decisions by interacting with its environment. The agent learns through a trial-and-error process, receiving feedback through rewards or punishments based on its actions.
One key advantage of using RL in portfolio optimization is the ability to enhance risk management. RL algorithms can learn to balance potential returns against associated risks by evaluating the outcomes of various investment strategies over time. This capability helps construct portfolios that aim for high returns and minimize potential losses.
3. Chatbots for Financial Advisory and Customer Service
Machine learning-based chatbots offer financial advice, answer customer queries, and enable simple transactions. These chatbots are fully autonomous; however, they can hand off the conversation to a human agent when tasks are complex and require detailed intervention.
Erica, the Bank of America AI assistant, employs machine learning to provide customers with personalized financial information. It uses data analysis to suggest action plans based on spending patterns and helps customers schedule appointments at financial centers.
Erica is available 24/7 to Bank of America customers, assisting them with various interactions previously handled by human agents (Source: AIM Research).
Retail and E-commerce
Machine learning plays a significant role in enhancing customer experiences, improving inventory management, and optimizing pricing strategies in the retail and e-commerce industries.
Machine learning enables personalized recommendations and demand forecasting by leveraging customer behavior and market trends. However, its adoption requires careful attention to data privacy, potential biases, and integration complexities into existing systems.
Let us explore some of the primary applications of machine learning in the retail and e-commerce industry:
1. Visual Search and Image Recognition
When shopping on Amazon, you can search for products by typing keywords into the search box or uploading an image to find similar items. This mode of visual search and image recognition has been made possible by advances in machine learning, especially convolutional neural networks (CNNs).
CNNs enable systems to identify and match products with remarkable accuracy and speed by analyzing the uploaded image's patterns, shapes, and features.
2. Supply Chain Optimization
Retailers can use machine learning models to monitor supply chain parameters, such as inventory and shipment time, to report anomalies during delays or stock shortages. By analyzing historical data with real-time inputs from these models, retailers and e-commerce companies can also predict equipment failures in warehouses or transportation vehicles.
Anomaly detection improves demand forecasting by optimizing inventory levels to account for sudden spikes in demand. Leading retailers such as Target use advanced machine learning-enabled analytics to optimize the timely delivery of goods (Source: Progressive Grocer).
Manufacturing
Machine learning is helping improve efficiency, enhance quality control, and enable predictive maintenance in the manufacturing industry. Machine learning can identify patterns to reduce downtime and optimize resource utilization by analyzing production data and equipment performance.
Here are some of the key applications of machine learning in the manufacturing industry:
1. Adaptive Robots
Robots were used in the manufacturing sector long before AI and machine learning became mainstream. However, most of these robots were static, unable to learn from experience.
With the help of machine learning, manufacturers can now deploy adaptive robots that enhance their efficiency and adjust their behavior to meet specific expectations. For instance, BlueScope Steel, an Australian steel manufacturer, leverages Senseye Predictive Maintenance, powered by machine learning, to monitor critical metrics like pressure, temperature, and vibration across modern and legacy equipment, enabling early detection of issues such as hydraulic leaks and preventing costly unplanned downtime. By combining IoT sensors with AI-driven forecasting, BlueScope has enhanced operational efficiency, improved workplace safety, and reduced maintenance costs through a proactive, data-driven maintenance strategy. (Source: Siemens Blog)
2. Augmented Supply Chain Automation
Machine learning positively impacts supply chain logistics by allowing systems to make real-time, intelligent decisions during disruptions. These disruptions can be as simple as shipment delays and inventory shortages or as complex as sudden surges in demand, which traditional models of supply chains cannot handle efficiently.
DHL uses its Resilience 360 system to identify risks such as natural disasters, political upheavals, or closure of trade routes. Based on these early predictions, DHL adapts quickly by routing shipments through alternative routes, thereby reducing delays and maintaining an agile supply chain (Source: Procurement Magazine).
Machine learning drives innovation across industries by enabling intelligent automation, predictive analytics, and personalized experiences. It optimizes processes, enhances decision-making, and efficiently addresses complex challenges in the healthcare and manufacturing sectors.
Data Science vs. Machine Learning: Which is Better?
Whether data science or machine learning is better is akin to asking which is more critical for a business—vision or action? Both are indispensable and work in tandem to drive progress.
Data science is a comprehensive discipline that encompasses the entire lifecycle of working with data, from collection and cleaning to analysis and visualization, all aimed at informing decision-making. On the other hand, machine learning is a subset of data science that primarily develops models that enable systems to learn and make predictions autonomously.
Data science is a multidisciplinary field that combines statistics, data engineering, and domain expertise to answer complex business questions. A data scientist may predict customer churn, optimize marketing campaigns, or analyze supply chain efficiency.
So, which is better, data science or machine learning? Well, both are equally important. Data science provides a framework for a problem, while machine learning strengthens it by adding predictive and adaptive capacities. Together, these two drive innovation and actionable intelligence for an organization.
Data Science and Machine Learning: Sustained Innovation with Tredence
Advancements in data science, machine learning, and AI mean that the power of data drives unprecedented innovation and opportunity. These disciplines are catalysts for a future defined by smarter decisions, personalized experiences, and sustainable solutions.
From revolutionizing healthcare to optimizing global supply chains, their impact reshapes industries and redefines what is possible.
The possibilities of data science and machine learning seem limitless—a testament to human ingenuity and our ability to harness technology for a brighter, more connected world.
Organizations adopting these technologies are gaining a crucial first-mover advantage. However, thorough planning and execution are required to incorporate these innovations successfully.
Winning enterprises have moved beyond the AI hype and are harnessing its true potential to transform their business operations. At Tredence, we combine deep data engineering, data science and machine learning expertise with extensive domain experience to help the world’s top enterprises modernize their data foundations and turn enterprise data into actionable insights through AI and ML.
Our portfolio includes over 140+ AI/ML accelerators designed to tackle even the most complex data science and machine learning challenges. These accelerators empower the largest and fastest-growing enterprises to unlock unprecedented value from their data. Using our accelerators, clients can accelerate time-to-value by more than 50%—achieving meaningful results in weeks, not months.
Unlike outdated SaaS platforms that require lengthy implementations, Tredence data science and machine learning accelerators are customized to each enterprise's data landscape and unique business requirements. They reduce the total cost of ownership, capitalize on the power of hyperscalers, and safeguard your data—delivering the speed and security necessary to keep pace with today’s demanding consumers. Our approach delivers tangible results, backed by an industry-leading 94% Net Promoter Score across 1,000+ project engagements.
Contact Tredence today and transform your organization with advanced data science and machine learning solutions.
FAQs
1. Data science vs machine learning–what is the primary difference?
Data science focuses on extracting insights and solving problems using data, while machine learning enables systems to learn and make predictions autonomously.
2. Can machine learning exist without data science?
No. Machine learning relies on data science for data preparation, cleaning, and insights to build and train effective models.
3. Which is better for businesses: data science or machine learning?
Both are essential—data science defines the framework and strategy, while machine learning enhances predictions and automates processes.
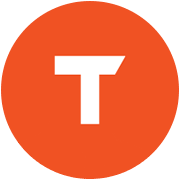
AUTHOR - FOLLOW
Editorial Team
Tredence