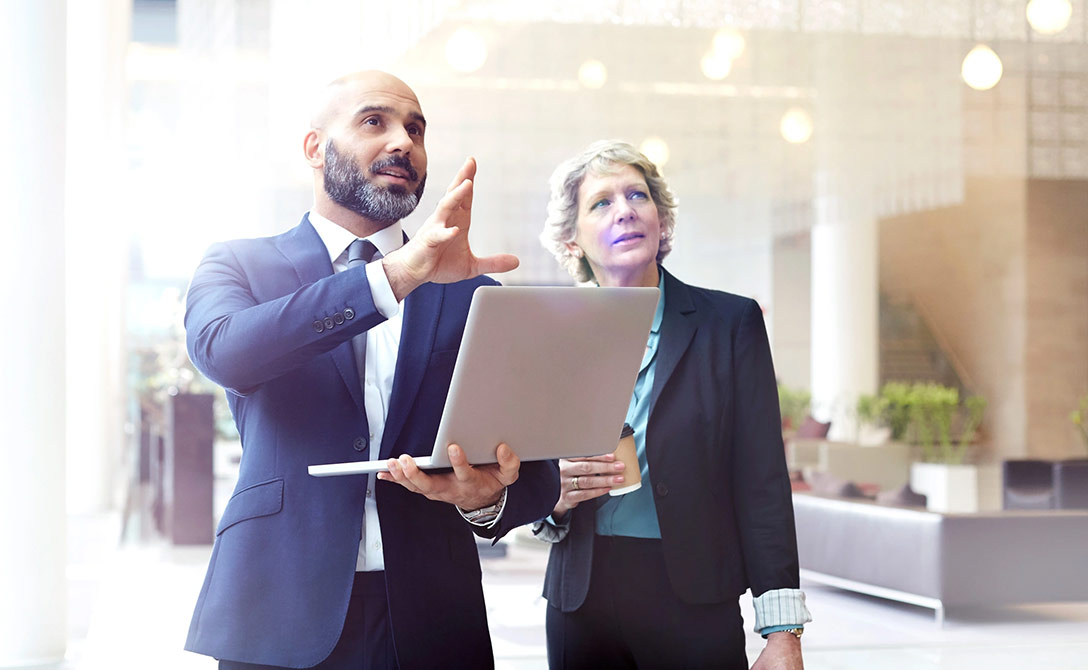
Until 2022, most businesses were complacent about dealing with unexpected events and crises. However, the pandemic upended many normal structures and processes across industries, inviting extreme levels of uncertainty. Supply chain disruptions became too familiar in the form of shortages, businesses shutting down, and goods lying idle at ports. As a result, companies were forced to revolutionize their working models to withstand the crisis caused by COVID-19. Thus, businesses responded by re-assessing their business resilience strategies to remain updated, flexible, and resilient at all times. The most effective way to prepare organizations against uncertainty is to measure this uncertainty.
Further, the healthcare crisis has turned greater attention towards the power of AI and analytics. ML models can determine outcomes based on the patterns and behaviors identified in the past. These models help predict what could happen and even recommend the best choices and options for businesses. An increasing number of companies are now employing predictive analytics to deal with the challenges of an uncertain future. According to a Gartner report, more than 70 percent of organizations will deploy AI models by 2025. Even with an increase in the use of AI/ML models, there is scope to improve how they are deployed and used.
In measuring uncertainty, there is a clear disconnect between prediction models and business outcomes, which makes uncertainty the primary cause. There are three major stumbling blocks to deal with: First, while testing the efficacy of models, companies don't account for both data and model uncertainty. Second, ML models lack the notion of reliability. And third, there is no transparency into the business outcomes based on the model predictions. Tredence, a leading AI and data science solutions provider approached the uncertainty challenge from two different perspectives. Since using a confidence score is a well-known approach to address one of the challenges, the Tredence Uncertainty Quantification Framework (TUQ) extends this in an innovative fashion to address all of these challenges.
Confidence scores
To illustrate the Tredence approach, we take the example of demand forecasting and start with how confidence scores work and their limitations.
Demand forecasting is the process of making predictions based on insights from historical data and other analytical information. Warehouse demand forecasting helps companies determine when, how much, and where they should deliver merchandise to optimize the supply chain and inventory. An important outcome for Tredence clients was to perform labor planning using the output from the demand forecasting models.
Labour planning involves forecasting the demand and supply of the workforce in an organization, helping businesses understand how many employees to hire to meet demand. Using confidence scores from the model helps businesses understand how much confidence the model had in its predictions.
Despite the high explainability of this approach, it is still difficult for clients to determine the threshold of confidence the model should show for them to arrive at decisions. For example, should the client hire their labor when the model's score stands at 60 percent or 90 percent? Tredence noticed that most clients didn't feel secure about their decision-making with this approach; likewise, it does not address data and model uncertainty.
Tredence Uncertainty Quantification (TUQ) framework
To that end, Tredence developed another approach to address the gap in the confidence score system. The company built an uncertainty estimation framework using tools like Temperature Scaling, Monte Carlo Dropout, and Deep Ensembles to quantify uncertainty. For example, if data can be considered in distribution, the model can employ temperature scaling to estimate uncertainty. In the case of transfer learning, the model can use the Monte Carlo dropout or a combination of Monte Carlo dropout and Deep Ensembles.
The model's predictions, along with the confidence scores are then fed into a scenario generator. The model also considers factors like the real-time business impact that a decision might have and the organization's risk tolerance capabilities. For example, if a company is employing the model for labor planning, the model grades the business as risk-taking, risk-neutral or pessimistic.
The scenario generator then creates a range of outputs in the form of dollar impact and presents them in a tabular form. Based on the numerous 'What If' scenarios mentioned in the table, the user can easily judge the economic impact on their business. This allows the business to connect the model predictions to the business outcomes and make informed decisions.
Conclusion
Often, the critical issue in ML models is the reliability and ability to connect the predictions to business outcomes. Uncertainty modeling helps in improving model reliability. The Tredence Uncertainty Quantification framework (encompasses the uncertainty modeling and the ability to convert model predictions into economic impact, inspiring clients to use the model output in driving their business. This is not limited only to the demand forecasting/labor planning problem—it can help retail and CPG domains across a wide range of issues.
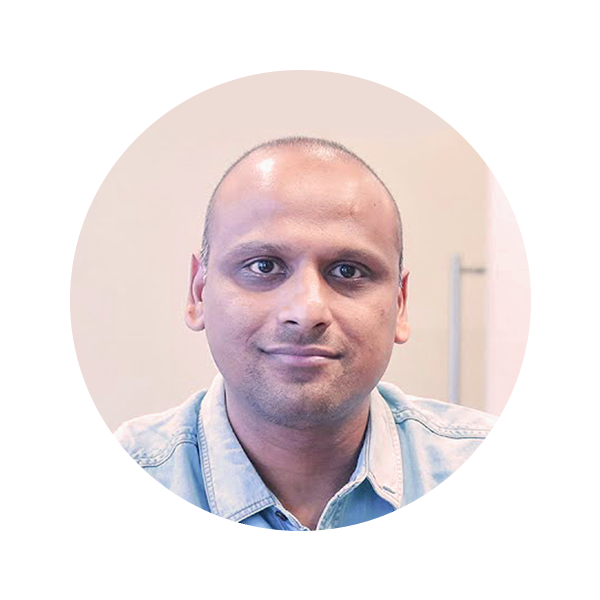
AUTHOR - FOLLOW
Aravind Chandramouli
Head AI COE, Tredence
Topic Tags