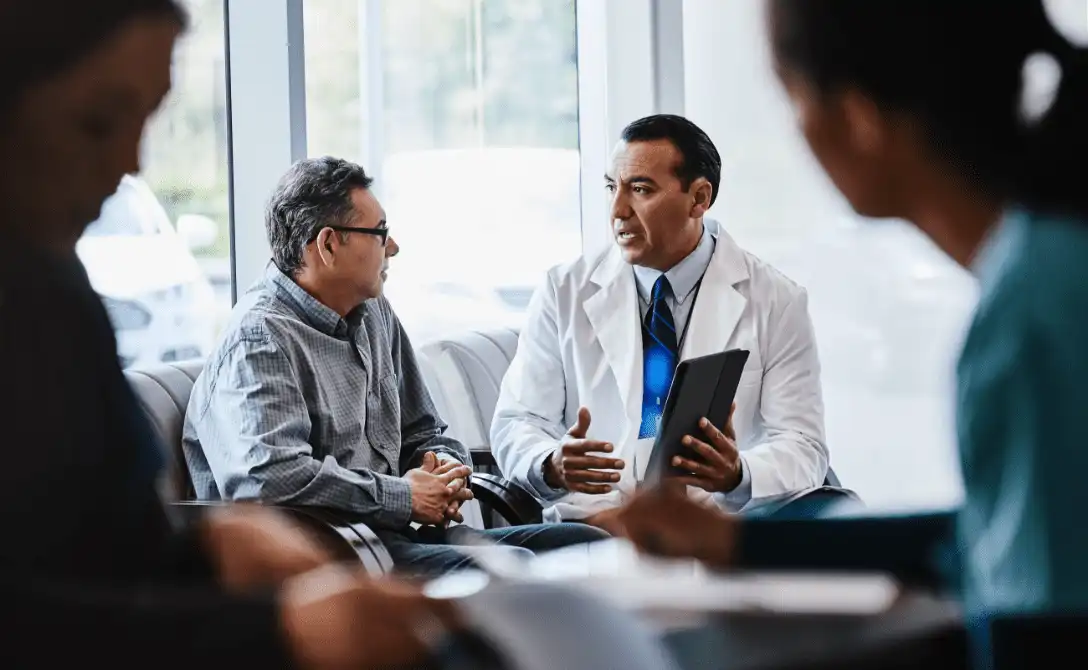
Shub Bhowmick is the CEO and co-founder of Tredence Inc. focused on solving the last mile problem in analytics.
The pandemic has been an unpleasant awakening for the entire healthcare industry. The health crisis has put immense pressure on the health system and has also exposed devastating disparities across race, ethnicity, gender, age, sexual orientation and socio-economic factors. As Dr. Martin Luther King, Jr. said in 1966, “Of all the forms of inequality, injustice in health care is the most shocking and inhumane.”
To add to this issue, healthcare disparity costs the economy an estimated $309 billion annually as recently as 2012. Also, the life expectancy difference is a whopping 10 to 15 years between privileged and vulnerable populations.
The Untapped Power Of Data-Driven Healthcare
For years now, big data and analytics have contributed significantly to improving patient outcomes and enabling value-based care. According to IDC, approximately 30% of the world’s data is being generated by the healthcare industry. By 2025, the compound annual growth rate (CAGR) of data for healthcare will reach 36%. Data has played a pivotal part in helping healthcare providers (HCPs) mount efficient defenses and prepare effective responses against the Covid-19 pandemic.
Data is ultimately the key to bridging the disparity gaps, but leveraging data comes with a disclaimer when it comes to healthcare. There’s often an inherent bias in sourced data that gets reinforced in downstream analytics, severely amplifying the existing disparity.
To correct course, HCPs must categorically harness the power of data to improve healthcare multi-dimensionally and ensure they represent the data of diverse populations to realize its full benefits. Here are a few of the data challenges aggravating healthcare disparity:
- Atomistic Data Sourcing and Management. Electronic Health Records (EHR) often leave out critical determinants of patient data such as income, race and ethnicity, leading to a data gap, which intensifies the bias in downstream analytics and decision-making, which can result in an inequitable delivery of care.To eliminate data disparity, it’s critical to have a holistic and standardized data collection strategy that will factor in ethnic and racial indicators of patients. And to further intensify accuracy, large sample sizes of data with self-reported race and ethnicity information are necessary to recognize data disparity among the population.
- Insufficient Standardization For Data Exchange. In a rush to digitalize data, healthcare participants have haphazardly adopted varied data standards for collecting, organizing and encoding clinical data. A wide variety of tools, formats and approaches are devoted to collecting the Social Determinants of Health (SDOH).Even with standard tools, HCPs still go about using their own formats. A common data vocabulary will help HCPs communicate quickly and seamlessly, creating a healthcare constellation supporting patients. Standardization in collecting the SDOH would also promote the interoperability required to bring all the subgroups of the population into the fold of accessible healthcare at the right time and with the right provider.
- Sample Size Limitations. Small samples limit researchers’ ability to measure disparities even among a larger subpopulation. To counter this, sample size, sampling extent and modeling approaches need to be reformed to include oversampling, periodic surveys and ensemble survey modes.
- Interoperability and Collaboration Gaps. HCPs, care management teams and follow-up teams often fail to collaborate productively and provide interdisciplinary training to their data teams.Healthcare participants must create multidisciplinary teams that use a standardized data vocabulary and empower them to exchange clinical data irrespective of their toolset.
The Trifecta Approach: Bridging the Healthcare Disparities Gap With Data Analytics
- Maintaining Data Equity Data equity at the source can help facilitate fairness in healthcare decisions. Reinforcing data equity at the retrieval and collection stages ensures that the machine learning models reflect the diversity of backgrounds and reduce bias in algorithms. A multipronged approach can help create holistic datasets that address the subpopulation markers through measures such as:
- Accounting for local social factors at the ZIP Code/household level so that the AI/ML models are localized, effective and efficient.
- Addressing sample size limitations can be achieved by using national surveys that oversample vulnerable populations, combining similar clinical surveys from various periods and leveraging targeted periodic surveys. These measures can help eliminate the profile gaps for the underserved populations.
- Incentivizing data providers to include diversity markers can promote the collection of race, ethnicity and income data.
- To reduce standard errors, modeling approaches shouldn’t focus only on larger population groups. The biases in insights can sometimes stem directly from ML teams and modeling techniques, not just from data.
- External Data Sources To decode each community’s unique healthcare requirements, healthcare providers need to build comprehensive patient profiles of the target population. HCPs should foster a larger ecosystem with specialists, healthcare agencies, pharma companies, communities, affiliates, technology partners and wearable manufacturers as a critical step to extend the ambit of data beyond EHRs. Factors to consider:
- Race, Ethnicity and Language (REaL data), demographic data, socio-economic clinical data, mediclaim data, data from public health agencies as well as self-reported and patient-generated remote data.
- Data collected by the care management team or member engagement tools (text, email and IVR responses) should be integrated back to the EHR.
- Contextual Machine Learning Models To Spot Disparity Patterns While it’s imperative to drive healthcare decisions with data, HCPs must ensure that the algorithms leading from data to decisions don’t carry bias by:
- Collaborating actively with their analytics partners to co-develop the predictive models driving these decisions.
- Creating a localized severity scoring system with features from proven studies. Research shows that existing scoring systems have built-in disparity due to issues such as outdated or missing data.
It’s time that HCPs focus on setting up holistic data practices and improving them with impartial data collection, analytics, and insights-driven intervention to bridge the healthcare disparity. The right Healthcare Analytics Consulting partner can help eliminate bias from each step of the data and modeling pipeline and help create sustained equity in healthcare.
This article was originally published on Forbes.com
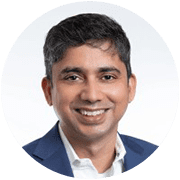
AUTHOR - FOLLOW
Shub Bhowmick
Co-founder and CEO
Topic Tags