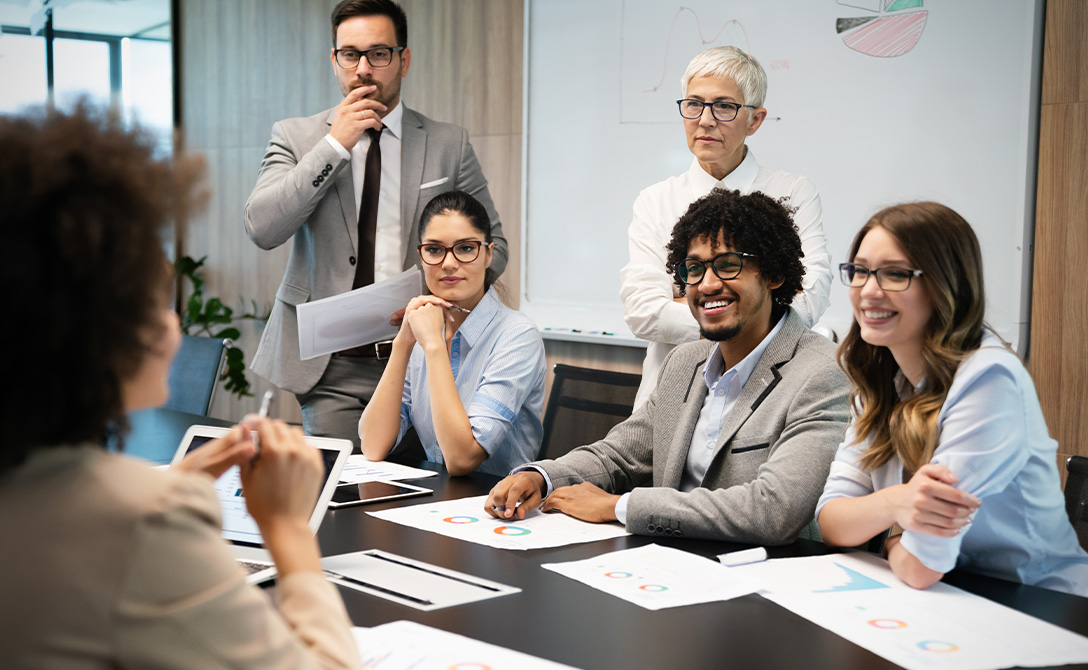
Over the past decade, data-driven business transformation has inspired enterprise leaders to reimagine business models, new revenue streams, customer experiences, operational models, and processes. Extracting actionable insights from business data often helps organizations bridge the last-mile gaps in analytics and drive faster value realization. In fact, it has grown so popular that market research by Global Newswire confirms the data science market is expected to be valued at nearly $133 billion by 2026.
Considering the impact that data analytics will have in the business world, it is crucial to identify the upcoming trends in this space. The following key trends will definitely play a role in shaping the data analytics industry of tomorrow.
Shifting from a project to a product mindset
Today’s forward-thinking clients demand a sustainable, mature product-centric delivery model from their data analytics partners and want them to move from a project mindset to a product mindset. Data science is not limited to just building an accurate algorithm and creating a dashboard around it. Clients today want to go beyond just fancy dashboards; they want the ability to create full-stack applications that can be integrated into an enterprise’s decision-making process. This requires a mature product mindset. Clients today want a custom product shop, not a project shop. Adopting a product mindset will also pave the way for data analytics solutions that increase revenue and have better business outcomes.
Overhauling the revenue model
Enterprises have dramatically shifted revenue models in the wake of the Covid-19 pandemic. Fixed cost models are a thing of the past and data analytics solution providers are expected to have more at stake with a gain-share model or an outcome-based revenue model. Since the success of a data analytics solution primarily lies in measuring tangible outcomes, clients find it prudent to share both profits and risks equally with solution providers. This also prompts data analytics companies to continuously tweak solutions to ensure that the right business outcomes are met.
Adopting Agile methodologies
The Agile way of working is well established in the software development industry. However, Agile methodologies have only recently begun to gain traction in the world of data science. They allow data scientists to optimize outcomes by prioritizing tasks based on preset benchmarks and performance goals while empowering them to iterate, learn new things, and experiment until the desired result is achieved, strengthening the product mindset.
Operationalizing data science
Clients prefer service providers who operationalize and standardize their data science models and move them towards production environments. The answer to operationalization lies in the three ops MLOps, AIOps and DataOps. MLOps or model ops refers to the collaborative process of building, managing, deploying, and constantly monitoring machine learning models to consistently deliver the right inputs to the organization. AIOps or artificial intelligence ops is the implementation of AI to IT operations with the goal of governing IT structures that are hybrid and distributed. It contributes to smarter, quicker operations within an IT framework by managing the large amount of data generated. DataOps is an innovative methodology that seeks to optimize the time of a full-cycle data analytics solution. Its Agile approach to data analytics ensures that data scientists and users work together to create valuable analytical insights.
Last-mile in data science
Conquering the last mile in data science can be an enormous task. After the entire data collection, preparation, exploration, and modeling lifecycle, the last-mile adoption includes operationalization and translating those data models into actionable insights that drive business impact. This information can further be used to bridge the gap and offer cognizant inputs to drive operational changes in the enterprise. To ensure last-mile adoption, data scientists must start with conceptualizing solutions with the end goal in mind to ensure a clear blueprint to detail the kind of insights required to reach the set goal.
Another roadblock to last-mile adoption is the lack of data interoperability. Leveraging the right data and capitalizing on a robust data strategy hones the focus on execution for data scientists.
In conclusion, enterprise innovation has changed — so has its work, methodology, scale, and speed. Data is at the epicenter of this change. Large enterprises are feasting on data, but they’re starved for insights. To drive successful adoption, analytics must be a holistic initiative that solves entire problem spaces. Data analytic strategies are best executed as a long-term vision at scale with incremental short-term wins, with participation from all functions with a stake. These impactful trends will change the shape of data analytics and technology in 2022 and beyond.
Author:
Shashank Dubey, Chief Revenue Officer & Co-founder, Tredence
Topic Tags