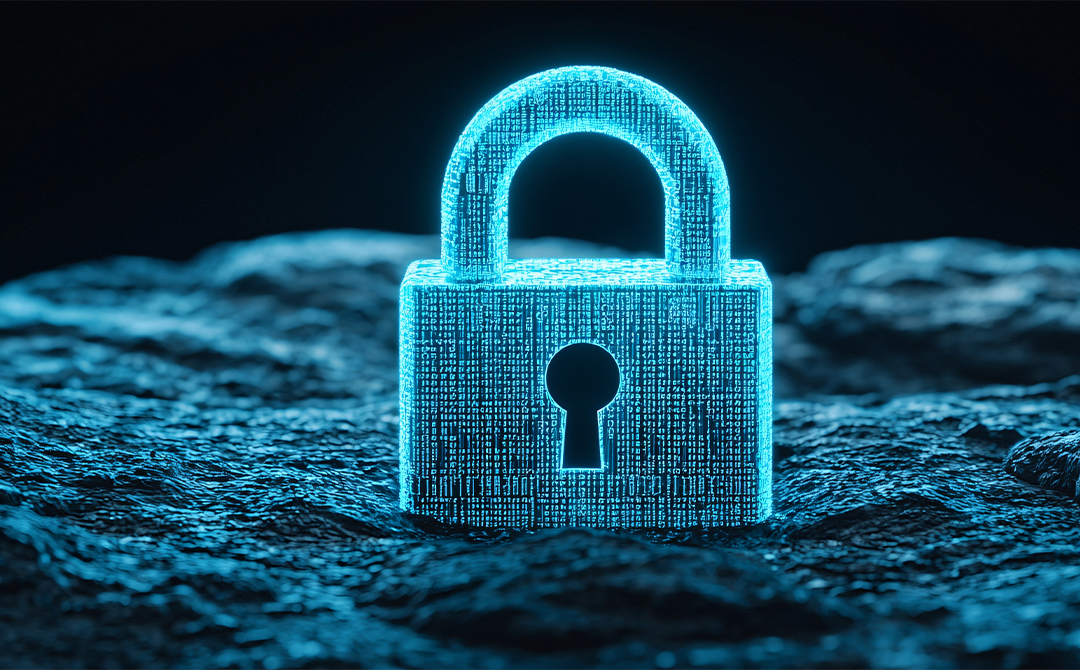
Introduction
Large Language Models are contributing a great deal to business operations today. Be it software development or market analysis, customized LLMs have shown notable capabilities since the public debut of foundational models such as ChatGPT and Gemini a couple of years ago.
But there is also a complex side. Organizations need to be keenly aware of how the adoption of these models that collect, process, and retain large volumes of diverse and sensitive data expands the risk surface.
Say, a financial firm's customer service LLM reveals anonymized transaction patterns during routine inquiries. Such exposure could trigger hefty regulatory penalties under SEC regulations and FINRA, with potential fines in the millions. If you think LLM risk management in 2025 is just about protecting raw data, the stakes are far higher.
This article introduces the risks, breaks down what matters for your security team, and outlines actionable strategies to protect your data.
LLM risk management involves systematically mitigating potential risks while deploying large language models. This includes frameworks for identifying, prioritizing, and minimizing hallucinations, data privacy violations, and data and system security risks, to ensure the ethical and secure use of LLMs in business.
What Are Some Risks Of LLMs?
Nearly seven in ten organizations use large language models, an O’Reilly report has found. These systems are trained on billions of data points to create human-like responses and output. They are powerful but have vulnerabilities too.
Amir Feizpour, CEO of AI Science, told Forbes, "They are as unbiased and objective as their designers." He also points out that these models are "based on statistics, not causal reasoning."
So, companies need to address the risks that come with LLM adoption. Here are the most pressing ones that demand attention.
Information privacy risk: Organizations routinely share client names, project details, and internal documents with their LLMs. Research shows that these models keep exposing sensitive data even after companies try to remove it. Clearly, this can injure company reputation and trigger privacy law violations.
Training data security: Since LLMs store all the pieces of information fed into them, including the not-so-important ones, this can lead to trouble with training data. Research shows competitors can reconstruct training data through systematic querying. This means your product roadmaps, pricing strategies, and customer insights could leak through simple LLM interactions.
Misinformation: LLMs produce convincing but fabricated responses (aka hallucinations) because they learn from patterns rather than logic. A Stanford study says one in six responses from LLMs used in the legal space are hallucinations. The implications of this in sectors like healthcare and education are very worrying.
LLM Risks In Terms Of Cybersecurity
As a new technology that interacts closely with humans and operates across ecosystems, LLMs open some very critical cyber security gaps that your teams cannot miss in the rush to deployment. Let’s look at the risks one by one.
Prompt injection: It’s a case where attackers can manipulate input prompts to gain unauthorized access to sensitive data. When you skip input validation (a common blunder among most organizations), data becomes vulnerable. The consequences? Data infiltration, unauthorized actions, and even social engineering attacks such as phishing.
Insecure plugins: When third-party plugins are used, they can connect your LLM to other tools or data sources. And if there’s no proper testing done, the plugins become the entry point for attackers. All it takes is one weak link to corrupt your system.
Denial of service: This is when LLMs are overwhelmed by a flood of requests. When organizations fail to establish user limits or authentication, the system delivers poor service quality or becomes completely unresponsive.
Excessive agency: Sometimes, organizations let LLMs have too much autonomy. This leads to unauthorized actions and data exposure. You need clear boundaries on what your LLM can and cannot do.
Supply chain attacks: They do not target your LLM directly but the underlying services and components that power it. Attackers can easily exploit these unsecured frontiers to breach your main system.
Poor output handling: Most security teams also overlook output handling. The resultant vulnerabilities in LLMs if criminals lay their hands on these outputs are cross-site scripting, remote code execution or cross-site request forgery.
Why Is Data Security Important In LLMs?
Data security is mandatory for any organization to its operations in the age of AI and LLMs. Recent studies show data breaches now cost organizations $4.88 million on average — a 10% increase from previous years. While this is not directly attributable to the new technologies yet, their rising use of structured and unstructured data from diverse sources will only increase the risk.
Specific to LLM risk management, securing data becomes important for a few more reasons.
- LLM data security gaps affect your legal standing, too. Under GDPR and CCPA, organizations face hefty fines for exposing personal data. A single security oversight can trigger violations across multiple regulations — HIPAA for healthcare data, SOC 2 for service organizations, and industry-specific standards for your business.
- Customer trust takes years to build but vanishes instantly after a breach. 66% of US consumers would not engage with companies that mishandle their data, and you can expect a direct impact on revenue, partnerships, and market position.
Source: LLMs in Crosshairs: Why Security Can't Wait - Venture Highway
How To Improve Risk Management For LLMs?
True, LLMs come with security risks. But integrating them into your business in one form or another is non-negotiable today. The following sections present an actionable plan to protect your systems while still getting the most value from your LLMs.
LLM Risk Management Assessment Prioritization
Kick off your planning with a systematic assessment of threat potential.
Begin by identifying the risks to critical assets like client data, intellectual property, and sensitive business plans.
Next, monitor how teams interact with LLMs and track third-party vendor access to spot vulnerabilities.
Conduct regular audits as new features and business needs can create unexpected security gaps.
Remember that risks often cascade - a single data leak can trigger customer losses, legal issues, and reputational damage simultaneously.
Source: LLMs in Crosshairs: Why Security Can't Wait - Venture Highway
LLM Risk Management Strategies
Once you’ve assessed your areas and priorities for LLM risk management, go ahead and implement a strategy that comprises the following. Remember, your strategy must be tailored to your organization’s unique risk factors and operations.
Read your vendors’ license agreements carefully: Ask specific questions like — Are they using subcontractors? Where do they store your data? What level of system access do they need — read, write, or admin?
Use VPNs and private networks for external connections: Public networks leave your LLM traffic exposed. A dedicated VPN keeps your data safe when teams work remotely or connect to outside services.
Set up clear data rules for your teams: Create allowlists of approved data types and content. Add validation steps that spot risk elements before they reach the LLM to stop malicious code and sensitive data from entering your system.
Define clear steps for mitigating hallucinations: Here is a 9-step approach that has been tested in live situations. As a part of their hallucination mitigation, many firms also use Retrieval Augmented Generation (RAG) in conjunction with other techniques.
Build strong access controls: Not everyone needs full access to LLM tools. Give Role-Based Access Controls (RBAC) and monitor usage patterns to flag unusual activity early.
Add security layers to your LLM setup: Use encryption for data transfers, require two-factor authentication, and regularly test your security measures. Keep detailed logs of who uses the system and how.
Set up a governance framework: This is essential to ensure LLM risk management is a standardized, ongoing organizational practice. Industry bodies like the non-profit Open Worldwide Application Security Project (OWASP) provide guidelines.
Train your teams: Show them how to use LLMs safely, what risks to watch for, and when to report problems. Make security part of their daily routine, not a nice-to-have.
Stay alert for new threats: Technology changes fast, creating new security gaps. Run regular audits, keep your security team updated on LLM developments and adjust your protection measures accordingly. Pay attention to retraining models regularly based on your learnings.
Consider the DREAD Framework as a foundation for your strategy: It is a risk assessment model developed for assessing cybersecurity threats that can be adapted to evaluate risks associated with LLMs.
Source: Threat Modelling and Risk Analysis for Large Language Model (LLM)-Powered Applications |
The most effective way to make LLM risk management strategies work is to have multiple security techniques working together. The following Large Language Model risk management techniques target specific issues, so you can use a combination.
Data minimization: Store only what your LLM needs for accurate results. Remove unnecessary data during training, fine-tuning, retrieval-augmented generation (RAG), and user interactions.
Adversarial training: Test your LLM against real attack scenarios during development. Feed it modified inputs that mimic actual security threats.
Model monitoring: Security vulnerabilities often start small and grow over time. Track your LLM's performance daily to catch compliance issues, biases, or security problems early.
Federated learning: Instead of storing all training data in one place, spread it across different computers. Your LLM still learns from all the data, but hackers can't access everything at once.
Reinforcement Learning from Human Feedback (RLHF): Most LLMs learn automatically based on set rules. RLHF adds human reviewers who check if responses align with your values and requirements. This cuts down on harmful outputs and biases during the training phase.
Differential privacy: Add noise to your training data in such a way that your LLM can identify patterns but not violate privacy norms. This sanitizes sensitive information that might have slipped through your first round of cleaning.
Examples Of LLM Risk Management
1. How a major provider, Amazon, is helping companies enhance their LLM risk management:
Amazon Bedrock recently added guardrails that let organizations control AI interactions. The system spots and blocks harmful content categories. The security features include:
- Automated Reasoning to help prevent factual errors from hallucinations
- Blocking of up to 85% more undesirable and harmful content
- Filtering out over 75% hallucinated responses from models for Retrieval Augmented Generation (RAG) and summarization use cases.
2. How a leading FMCG is responsibly and ethically transitioning into the new technology
The EU AI Act, the first comprehensive AI regulation, came into force on 1 August 2024.
By then, Unilever had robust risk management fully integrated across the organization. With over 500 AI systems globally, ranging from AI-driven R&D to generative AI-powered consumer experiences, the conglomerate has a Responsible AI Framework that governs the development, deployment, and usage of AI. Proof of concept projects go through an assurance process at an early stage. For regulatory compliance, potential new projects are assessed by a cross-functional team, including external partners.
Conclusion
A solid LLM risk management plan starts with understanding your specific threats — from data leaks to prompt injections. Companies like Amazon and Unilever show us it's possible to use AI safely while staying competitive.
“Tredence has a unique generative AI policy to ensure robust security. This includes setting up a generative AI working group, a risk framework system, and generative AI security control system.” - Chinmaya Jena, Senior Director, Tredence |
Success comes down to picking the right strategy, training your team well, and staying alert to new risks. Most importantly, treat risk management as an ongoing process. Your approach must evolve as LLM technology grows. Work with a data science and analytics firm to deploy LLMOps capabilities to standardize this all-important process.
FAQs
1. What are the key risks associated with LLMs?
LLMs can fabricate answers, deliver biased outputs and violate copyright issues while generating content all of which put your brand reputation at stake. They can compromise private data and cause security breaches through prompt injection attacks.
2. How can I mitigate the risk of prompt injection attacks?
You can create layers of defense against prompt injection in a few ways.
- Validate inputs to block malicious prompts.
- Filter and encode all user inputs before they reach your models.
- Keep your systems current with regular model updates and fine-tuning focused on security.
- Track every interaction with your LLMs — unusual patterns often signal potential attacks.
3. What is a zero-trust approach in LLM risk management?
LLM risk management with zero-trust means treating every input as potentially harmful — from your team or external users. The system verifies each request before granting access, limits user permissions to only what's necessary, and keeps monitoring interactions with the model.
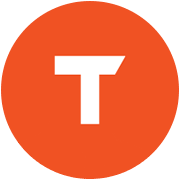
AUTHOR - FOLLOW
Editorial Team
Tredence