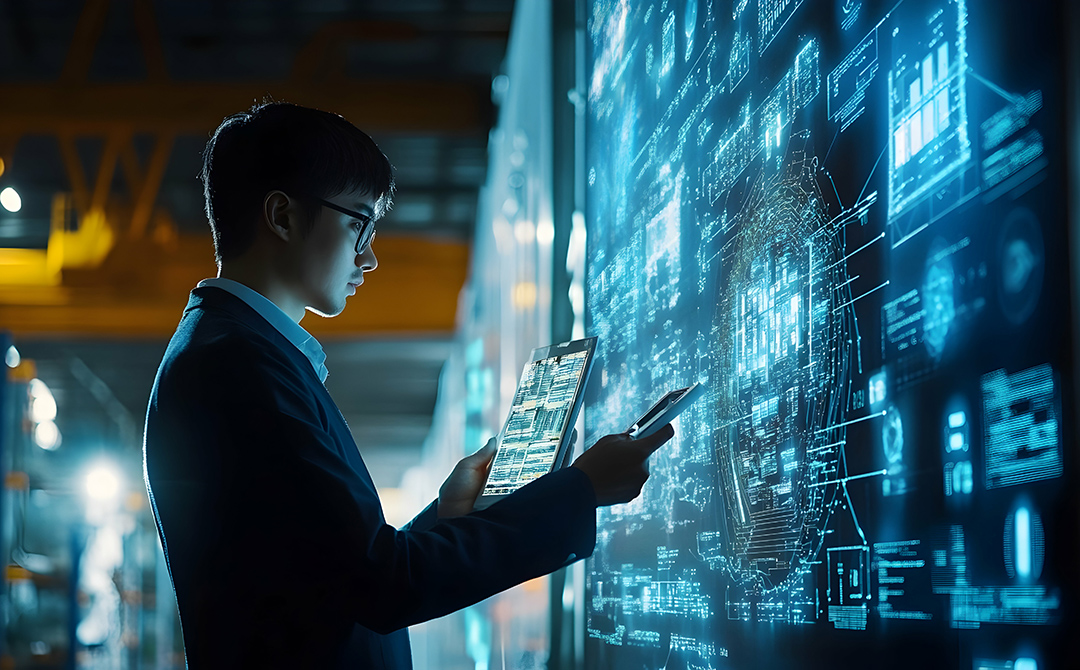
Enterprise leaders are keen on leveraging generative AI to transform their businesses. Since the release of ChatGPT in late 2022, many organizations have started experimenting with large-language model (LLM) capabilities. Common applications include summarizing text, analyzing input, generating content, and answering questions. However, most enterprises are still in the experimentation phase and have not yet fully deployed generative AI in their operations.
Moving Beyond Experimentation
While over 70% of organizations are experimenting with LLMs, only 18.2% have implemented them. This hesitation stems from concerns about data privacy, security, model hallucination, and bias. Yet, the greatest risk is falling behind as other organizations advance their LLM capabilities. Early adopters will gain a significant advantage by deploying and scaling LLMs across various use cases and business functions.
Understanding LLMOps
LLMOps, combining MLOps with specialized processes, is essential for developing, productionizing, and deploying generative AI models at scale. Traditional MLOps processes include designing, testing, deploying, and monitoring models. LLMOps extends these to address unique challenges such as creating scalable infrastructure, protecting data, and leveraging new skills.
Key Components of LLMOps
- Scalable Infrastructure and Architecture: Setting up the necessary infrastructure and cloud layers for training and deploying models.
- Data and Model Protection: Implementing controls to mitigate data privacy risks and protect models from misuse.
- Leveraging New Skills: Involving LLMOps engineers, prompt engineers, and SecMLOps experts to develop and manage models effectively.
Benefits of LLMOps
Implementing LLMOps processes can lead to significant benefits, including:
• Significant cost savings through effective resource provisioning and cost controls.
• Reduced API calls to less than half the amount.
• Faster deployment of new capabilities, accelerating time to market.
• Improved model performance and output quality.
Overcoming Challenges in Generative AI Deployment
Several factors hold enterprises back from fully deploying generative AI models:
Setting Up Data and LLM Processes
Teams need to select suitable foundational models, design architectures, secure data, and develop new skills. Implementing frameworks, storing and securing data, developing, managing, and monitoring models, and tuning them for accuracy is crucial. Teams must also engineer prompts, fine-tune models, and monitor outputs to ensure relevance and quality.
Ensuring Compliance and Security
Addressing risks such as prompt injection, insecure output handling, and model theft by implementing robust frameworks is essential. This includes governance and security guardrails, quality checks, and human review where necessary to mitigate risks identified by OWASP.
Trusting Output
Challenges such as hallucination, bias, data privacy, and security have been well-documented. Implementing frameworks with security and governance measures ensures the quality of outputs and enterprise data protection.
Managing Time and Costs
Avoiding skyrocketing costs by right-sizing LLMs and implementing cost-effective LLMOps processes is vital. For example, training costs for advanced models can be prohibitively high without proper management.
The Roadmap to LLMOps Maturity
1. Create an LLMOps Landing Zone
The first step involves creating an LLMOps landing zone. This includes understanding LLM capabilities and infrastructure by creating a business case for new generative AI capabilities, designing initial prompts, and pre-selecting foundational models. Teams should set up the necessary infrastructure, including storage, serving, and security layers, and test foundational models while logging results to evaluate performance against cost, speed, precision, security, and scalability goals.
2. Create Repeatable LLMOps Processes
Once foundational models are tested, the next step is to develop repeatable LLMOps processes. This involves fine-tuning foundational models to improve outputs and training teams on new processes while establishing a serving architecture. Automating processes by chaining prompts, registering and versioning applications, logging data inputs and outputs, and analyzing and filtering results is crucial. Developing rating mechanisms to validate output quality and perform security and privacy checks ensures the integrity of these processes.
3. Develop Reliable LLMOps Processes
As these processes become repeatable, the focus shifts to developing reliable LLMOps processes. Teams should fine-tune personalized LLMs to specific needs, manage data dependencies and organizational templates, store and log prompts, and test prompt lineage. Implementing transfer learning to reuse models for new problems, setting up pipelines and automated processes to scale model deployment, and standardizing serving processes, including API testing, rating, and reliability checks, are essential. Establishing tradeoff controls to balance performance and cost objectives, and continuously monitoring and analyzing to identify and mitigate risks, will further ensure reliability.
4. Scale LLMOps Capabilities
The final step is to scale LLM capabilities across the organization. This involves developing an organizational repository of fine-tuned LLMs and automating control flow, agents, and tools for training and serving pipelines. Enhancing prompt engineering with vector databases and prompt augmentation, ensuring robust and reliable model outputs through continuous monitoring and automated retuning, and implementing comprehensive governance to maintain data security, privacy, and compliance with regulations are critical. Codifying AI principles to minimize hallucination and bias in model outputs ensures the integrity and trustworthiness of the deployed models.
Conclusion
Enterprises must develop LLMOps capabilities to stay competitive in the generative AI landscape. By moving forward with LLMOps, organizations can accelerate growth, enhance productivity, and achieve new ROI from generative AI. Seize the acceleration advantage and drive business transformation with effective LLMOps implementation
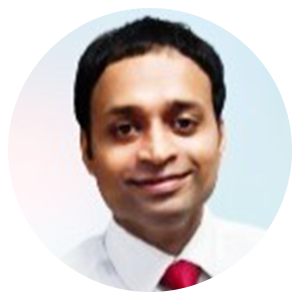
AUTHOR - FOLLOW
Ravindra Patil
Vice President, Data Science, Tredence Inc.