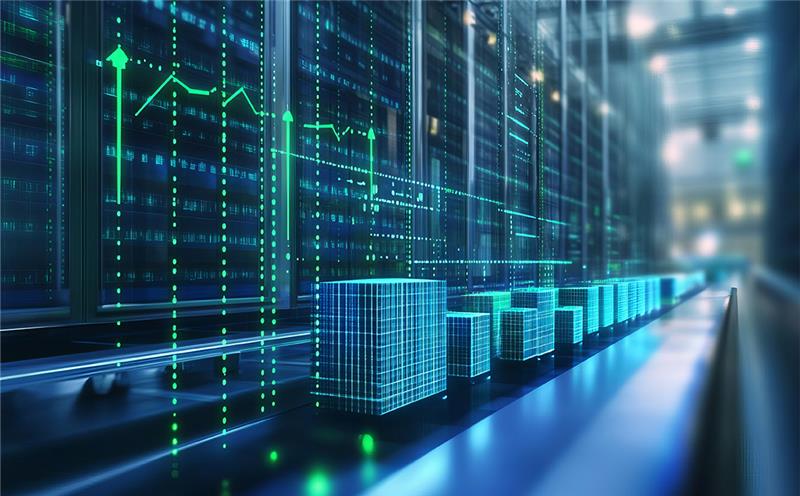
Businesses face ongoing pressure to anticipate demand, manage inventory, and adapt to supply chain disruptions. By using advanced AI-driven forecasting, they can transform raw data into powerful insights, ensuring agility, cost efficiency, and alignment with evolving market demands.
As a critical part of an effective supply chain, forecasting empowers businesses to navigate uncertainty with precision and purpose.
What is Supply Chain Forecasting?
Supply chain forecasting is the process of analyzing data to anticipate demand, supply, or pricing. In supply chain forecasting, various algorithms pull data from suppliers and clients to project product prices. This helps businesses make informed decisions about inventory and pricing strategies.
The credit for developing supply chain forecasting is given to Dr. Jay Wright Forrester, an engineer and systems scientist from the US. He developed the system for understanding complex supply chains and how various factors impact these.
79% of companies with efficient supply chains experience higher-than-average revenue growth. Furthermore, 81% of supply chain professionals believe analytics is vital in reducing costs. Despite these obvious advantages, 63% of companies do not use any technology to monitor their supply chain performance. (Source: Michigan Tech).
Supply chain forecasting algorithms improve prediction accuracy by factoring in external elements such as weather or disruptions, enabling proactive adaptation and optimization of supply chains.
However, the converse is also true–inaccuracy in supply chain forecasting can exacerbate the bullwhip effect. In this phenomenon, small changes in consumer demand have a massive impact on the supply chain. The bullwhip effect induces errors and inaccuracies in supply chain forecasting, which can lead to an increase in cost by 10% to 20% (SpringerNature).
Innovation in supply chain forecasting, particularly machine learning-based forecasting, can potentially mitigate costly errors. A study by McKinsey suggests that AI-driven supply chain forecasting can lead to a 20 percent to 50 percent reduction in errors.
Why is Forecasting Important in Supply Chain Management?
Supply chain management is widely recognized as crucial for an organization's effective and smooth operation. Let's explore its detailed usage and impact on daily and critical operations.
Making strategic decisions
Forecasting is the foundation for informed decision-making in any organization. Using historical data, competitive analysis, and demand projections, you can strategize across multiple facets, including operations, finances, and inventory management.
For instance, Walmart, a global retail giant, is often considered a benchmark for using supply chain management effectively. It analyzes historical sales data, regional demand signals, and weather patterns to anticipate even minute changes in consumer demand and preference. Before Hurricane Katrina, its systems predicted a surge in demand for bottled water, flashlights, and other essential supplies.
Walmart pre-stocked this crucial inventory to ensure customers did not face ‘out of stock’ issues during a calamitous time. Its implementation of Just-in-Time (JIT) inventory forecasting model has also been key to helping the retail giant in boosting its savings, as per Panmore Institute.
Ensuring agility and adaptability
Accurate supply chain forecasting provides the flexibility needed to adapt quickly, helping you respond to sudden shifts in demand or supply chain interruptions. This agility ensures customer expectations are met, inventory levels stay optimal, and operations run smoothly, all while minimizing costs and maximizing efficiency.
For example, IKEA, a global furniture retailer, suffered disruptions to its supply chain during the COVID-19 pandemic. With the help of its effective supply chain forecasting, IKEA responded to the crisis by diversifying its wood supply sources and increasing production from regions less affected by the pandemic. Implementing a holistic operational strategy, deeply rooted in analytics and forecasting, helped IKEA maintain its sales during the pandemic.
Optimizing costs
Supply chain forecasting allows you to balance stock levels efficiently, preventing overstocking or stockouts. Accurate predictions reduce carrying costs, minimize wastage, and optimize storage.
For instance, Dell’s build-to-order (BTO) model is an apt example of the tangible impact of accurate supply chain forecasting and management on an organization's profit and loss. Dell has largely implemented its BTO system rather than manufacturing personal computers without accurately estimating demand changes.
It uses predictive analytics to assess demand across customer segments. By using this analysis, Dell pre-orders processors, displays, memory units, and other components. Estimates suggest that real-time forecasting within the supply chain has led to a 30 percent reduction in Dell's inventory costs.
Types of Supply Chain Forecasting Methods
Both quantitative and qualitative methods offer unique strengths in supply chain forecasting. Quantitative approaches provide data-driven accuracy for established products, while qualitative methods allow for insightful predictions in new or evolving markets.
By using these forecasting techniques in the supply chain, you can develop a robust forecasting strategy that adapts to both historical patterns and emerging trends.
Quantitative Forecasting
Quantitative forecasting uses historical data to predict future sales. This method assumes that demand will follow a pattern of past trends and calculates forecasts based on established formulas.
The quantitative forecasting method is effective when growth is consistent; however, it may overlook significant factors such as emerging market trends, competitive shifts or black swan events (COVID or global wars).
Widely used supply chain quantitative forecasting methods include:
- Exponential smoothing: This method assigns weights to historical data, giving more importance to recent data to predict trends. Ideal for short-term predictions, exponential smoothing offers a data-driven approach without extensive analysis.
- Moving average: This method relies on calculating averages over a set period, smoothing out fluctuations. While effective for steady demand, it often fails to capture seasonal or market-driven changes.
- Regression analysis: This method examines the relationship between variables (e.g., seasonality and sales) to make predictions. It is fast and relatively simple and is widely used for initial forecasts.
- Adaptive smoothing: This method captures data variations across time, enabling refined predictions. It relies on automation for real-time data updating, supporting more accurate forecasting.
- Lifecycle modeling: This method predicts a product's progression through market adoption stages, enabling businesses to effectively manage inventory and tailor marketing efforts throughout its lifecycle.
Qualitative Forecasting
This forecasting method is primarily used for new product lines, especially when historical data is limited. It helps businesses make informed predictions in such cases, ensuring more accurate planning.
Widely used qualitative forecasting methods are:
- Market research: This method analyzes demand potential for profitability through surveys, competitive analysis, and interviews.
- Delphi: This method gathers expert opinions to predict long-term trends. Experts are consulted individually, and their insights are analyzed collectively to identify key patterns.
- Historical analysis: This method uses sales history or competitor performance to forecast demand for similar products.
- Panel consensus: This method involves a collaborative approach, where team members at all levels contribute to discussions and collectively develop forecasts.
Data Sources for Supply Chain Forecasting
Effective supply chain forecasting relies on various data sources that provide the insights needed to predict demand, optimize inventory, and streamline operations.
Internal data sources
Internal sources include historical sales data, production records, and customer feedback. Analyzing past performance enables you to anticipate future needs. These records offer valuable insights into demand cycles, peak periods, and overall performance, enhancing forecasts' accuracy.
Historical sales data is one of the most valuable internal data sources for any organization. It captures consumer buying patterns and gives a clear view of best-performing and weak demand categories. Apart from historical sales data, other key internal sources of data include,
- Production records: These records provide information on capacities, lead times, efficiency levels, etc.
- Customer feedback: Provides a qualitative dimension to supply chain forecasting through surveys, support
- Inventory movement data: This data helps an organization understand the supply-demand equation, which is how quickly items are getting sold and which ones are getting overstocked.
External Data Sources
External sources, including competitor analysis, economic indicators, and social media trends, are equally crucial. They provide additional context for more accurate forecasting.
Examining external data can help identify market shifts, adjust pricing strategies, and respond to competitors’ actions.
Advanced AI and ML Tools
Advanced AI and ML tools incorporate vast datasets, from weather forecasts to global trade dynamics, offering real-time insights.
These tools also analyze patterns in purchasing behavior or social media sentiment, providing a comprehensive outlook that enhances agility and responsiveness in the supply chain.
According to a recent survey, 20% of supply chain leaders have already implemented AI and machine learning-based forecasting methods, while another 60% plan to do so soon. (Source: McKinsey)
A combined supply chain forecasting approach uses internal and external data sources to capture a full spectrum of market dynamics. This integration allows you to make data-informed decisions, adapt swiftly to changes, and maintain a competitive edge through precise and responsive forecasting.
Benefits of Supply Chain Forecasting
Supply chain forecasting can significantly enhance business performance by improving decision-making and operational efficiency.
Supply chain forecasting offers the following benefits:
Cost reduction
Accurate supply chain forecasting reduces costs by aligning inventory with actual demand. Overstocking often leads to high costs, tying up capital, while understocking results in lost sales and dissatisfied customers. Forecasting helps balance these factors, helping you achieve cost efficiency.
Procter & Gamble (P&G) uses collaborative forecasting and advanced analytics to optimize inventory levels across its supply chain. By sharing data with retail partners, P&G reduced safety stock requirements by 15 percent, saving an estimated $300 million annually. This was achieved by aligning replenishment schedules with real-time demand data, reducing overproduction and waste.
Improved customer satisfaction
Customer satisfaction depends on various factors; however, the unavailability of desired products or frequent 'out of stock' issues can also impact it. Accurate supply chain management ensures you manage your inventory based on customer desires and expectations.
For instance, Amazon’s success in e-commerce is partly attributed to its highly advanced demand forecasting capabilities. Amazon predicts demand down to the zip code level by integrating machine learning with consumer purchase patterns.
During the COVID-19 pandemic, Amazon could pre-position essential items such as sanitizers and face masks in high-demand areas, achieving 98 percent on-time delivery rates even during peak demand periods.
Efficient resource allocation
Efficient resource allocation is a key advantage of investing in supply chain management. It helps you optimize labor, production schedules, raw material procurement, and logistics.
Coca-Cola HBC, a partner of The Coca-Cola Company, has undertaken key initiatives to improve its supply chain with the help of advanced capacity planning and demand forecasting. These efforts have been crucial in aligning production and distribution with consumer preferences, helping Coca-Cola HBC systematically determine how to allocate resources to various projects.
Enhanced decision-making
Supply chain forecasting isn’t only a tool for operational efficiency–it helps executives make better decisions.
A compelling case study from Hamilton, Ontario, shows how sound supply chain analytics can help, even in highly critical environments such as healthcare.
The challenge for healthcare organizations was clear: maintaining sufficient red blood cells (RBC) inventory to meet critical patient needs while minimizing waste from unused units. Unlike e-commerce, this was not just a supply-demand issue but also a matter of citizens' health.
Traditional inventory management methods were not entirely successful in helping the organization achieve this. To address this, researchers developed a hybrid forecasting model that combined clinical predictors with real-time data analytics to anticipate short-term demand more accurately.
The impact was transformative. By implementing this forecasting model, the healthcare system reduced inventory levels by 40 percent without compromising availability.
Simultaneously, order frequency decreased by 60 percent, and wastage due to expired inventory dropped to near-zero levels. These outcomes were not incidental; they directly resulted from actionable insights provided by the forecasting model, which guided daily inventory and ordering decisions.
This example illustrates that supply chain forecasting goes beyond predicting future scenarios when integrated into decision-making processes.
Supply chain risk mitigation
The global semiconductor shortage after COVID-19 taught us the importance of supply chain risk mitigation. Although most companies were impacted by the semiconductor shortage, some were able to mitigate its risks better. Dell Technologies showed how good forecasting can help an organization manage its operations during raw material shortages.
Dell wasn’t just reacting to the shortage; it was anticipating it. Through predictive analytics and digital modeling, Dell mapped out scenarios well in advance, preparing for possible supply disruptions.
Dell was guided by data, which allowed it to prioritize high-margin products, such as enterprise solutions, and precisely manage customer expectations.
Another good example is Cisco Systems. As the pandemic reshaped global logistics, Cisco leveraged AI-driven forecasting to assess demand shifts and realign resources. Real-time analytics became the backbone of its decision-making process.
By reallocating inventory to high-demand areas and adjusting production timelines on the fly, Cisco stayed ahead while competitors waited for supplies to catch up.
Sound anticipation and forecasting can be the difference between companies that can mitigate risks associated with market changes in a balanced way.
Challenges of Supply Chain Forecasting
Effective supply chain forecasting is essential for driving efficiency and meeting customer demand. However, accurate data and sophisticated models are required to be truly effective.
Supply chain forecasting entails the following challenges:
- Data quality and accuracy: Reliable data is essential; inaccurate data leads to poor forecasts.
- Market volatility: Rapid changes in the market, such as sudden demand shifts, can adversely affect forecasts.
- Supply chain complexity: Complex supply networks can increase unpredictability.
Best Practices for Effective Supply Chain Forecasting
Accurate supply chain forecasting is the backbone of efficient operations, helping businesses anticipate demand, reduce costs, and improve customer satisfaction. You can enhance predictability and make data-driven decisions that drive success by implementing proven best practices.
Embrace AI and Machine Learning
Supply chains are complex and ever-evolving. That is why many organizations are turning to machine learning and AI agents to improve their supply chain management.
AI and machine learning make supply chain forecasting smarter, faster, and more reliable. These tools spot patterns you’d never notice—like how a tiny uptick in one product leads to a domino effect across your entire supply chain.
Accenture found that companies using AI-driven forecasting were able to reduce inventory errors by 50%. Imagine the impact on your bottom line, operations, and ability to gain a competitive edge. If you’re not using automation in 2025 and beyond, you miss out on opportunities to streamline your supply chain management and forecasting.
Collaborate Across Departments
Forecasting isn’t just about algorithms and data. It’s about people. And if your sales team, marketing department, supply chain managers, and other stakeholders are working in silos, getting accurate forecasting is a difficult ask.
According to Harvard Business Review, companies that collaborate across departments see a 20% boost in forecast accuracy. Better alignment is key to better forecasts and, eventually, better results. When cross-department unison becomes standard practice, its benefits rub off on many aspects, including the accuracy of analytics and forecasting.
Measure Forecast Accuracy
What gets measured gets improved. That’s why companies like Nestlé place a lot of emphasis on measuring everything. Every forecast, every adjustment, every outcome. The result? They’ve fine-tuned their process so well they’ve boosted supply chain efficiency by 15%.
Tracking accuracy isn't just about finding mistakes—it's about knowing which aspects need improvement and which ones are doing fine.
Conclusion
Effective supply chain forecasting is essential to stay resilient, cost-efficient, and agile in today’s evolving and competitive market.
By combining advanced quantitative and qualitative methods, you can accurately anticipate demand and make proactive, data-driven decisions. Tredence, a leading data & AI company, has wide expertise in providing AI-powered supply chain forecasting management.
Through its customized solutions, including supply chain control tower and smart transportation planning, Tredence helps you simplify complex processes and drive cost optimization across the supply chain network.
Contact Tredence today to explore its advanced supply chain forecasting solutions, which are designed to enhance visibility, optimize costs, and effectively manage evolving risks.
FAQs
- Is forecasting part of supply chain management?
Forecasting is a key component of supply chain management, as it provides the insights needed to make informed decisions regarding inventory, production, and distribution. - What are the three main forecasting roles in supply chain management?
Forecasting in supply chain management has three main roles: demand planning, which anticipates customer needs; inventory management, which helps balance stock levels; and production scheduling, which ensures the effective allocation of resources. - What two data types are used in supply chain forecasting methods?
Forecasting relies on two main data types: quantitative data, which includes historical records and statistical models to predict trends, and qualitative data, gathered from surveys, expert opinions, and market analysis. - What is the best forecasting method in the supply chain?
The best forecasting method depends on business needs, but exponential and adaptive smoothing are often preferred for flexibility and accuracy, especially in dynamic market conditions.
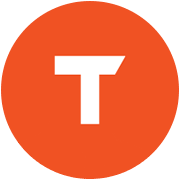
AUTHOR - FOLLOW
Editorial Team
Tredence