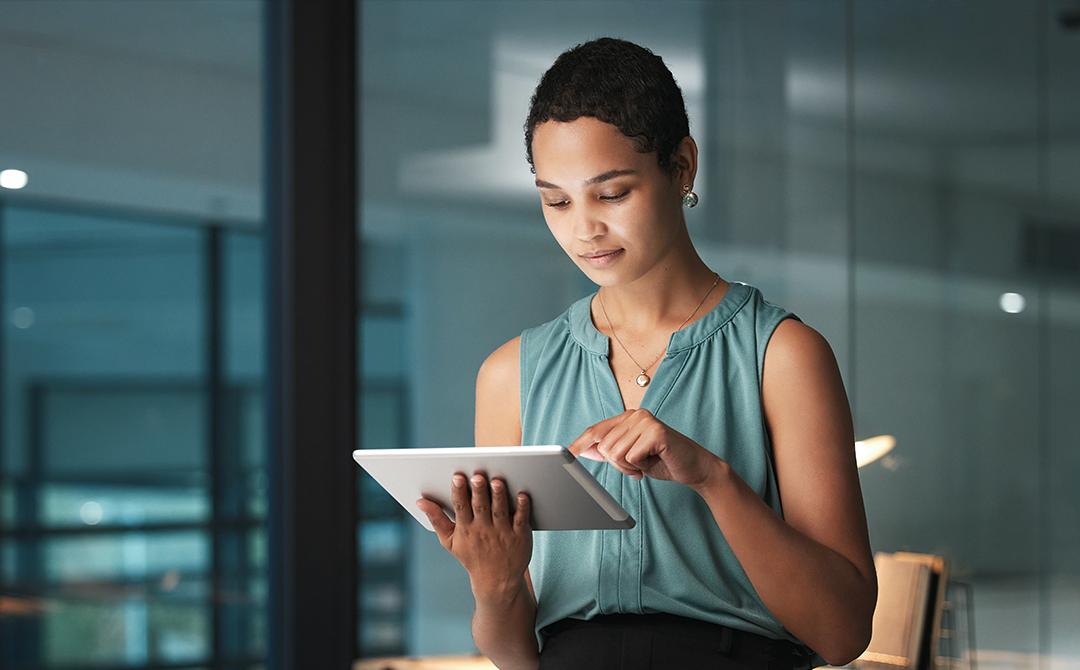
Databricks is all about comprehensive data governance, from data to AI toolsets, which has attracted data scientists to develop models using its platforms. On the other hand, Databricks is slowly catching up with the flexibility that Snowflake has provided its users since the beginning with features like instant startup and zero table management. While performance comparison has always been an ongoing debate, Databricks now presents a new argument, suggesting that its total cost of ownership is cheaper than CDW1 (Cloud Datawarehouse 1) without explicitly naming Snowflake.
Snowflake is all about bringing apps closer to the data because they have long enjoyed the superior performance of distributed data processing with instant startup and zero table management. Traditionally, Snowflake is just one of the data sources. However, through its partnership with Nvidia, Snowpark, and the introduction of Snowpark ML, it wants to close the AI gap with Databricks.
In the Databricks vs. Snowflake comparison, it is important to pay attention to the following:
- Is Databricks, with its open platform, setting standards beyond the Spark community?
- Will Snowflake’s AI capabilities see uptake due to their partnership announcements?
AI short-term threads mentioned by both Satya Nadella (CEO, Microsoft) and Eric Schmidt (Ex CEO, Google)
With tons of product updates, it is easy to lose track of the important things. If two very influential CEOs talked about the same thing without consensus, it merits paying close attention and listening again. While designing AI systems, we must prioritize the following:
- Preventing misinformation/disinformation
- Avoiding cyberattacks/bio threats that would impact thousands of lives
- AI guardrails – ensuring AI aligns with human values
Data Governance
Databricks, first of all, emphasize the importance of Unity Catalog (UC), as all their innovations are built on top of UC. Unity Catalog does not cost extra to upgrade, and successful implementation means a strong foundation for centralized data governance. However, organizations cannot upgrade UC at the press of a button. They must plan carefully to reap its full benefits, potentially needing to rearchitect their existing environments. But the advantage of UC, including centralized governance, lineage, and data sharing, simplifying the management and discovery process of data for organizations.
Lakehouse IQ
Lakehouse IQ is an offering to understand the ins and outs of an organization using LLM. Lakehouse IQ leverages all the internal signals happening around the Databricks platform to understand an organization, including but not limited to queries, lineage, dashboards, notebooks, and documentation defined within the Unity Catalog. The context of the organization is what Databricks said is the differentiator, like being able to understand the jargon used in the organization.
Without additional information, it is hard to understand what is under the hood of Lakehouse IQ. They didn’t say what model they are using for this LLM—whether it involves fine-tuning GPT-4 or if they have something more powerful than GPT-4. The amount of work it takes to reach an acceptable level for most users remains unknown. But one thing for sure is that Databricks wants people to use all of their toolsets to take advantage of the Lakehouse IQ behind. There is still a challenge because not all organizations are ready to migrate end-to-end data governance to Databricks.
Despite the uncertainty, it is still a good opportunity for partners because enterprises have been struggling with a single source of truth for a long time. With Databricks’ focus on bringing everything under one umbrella while ensuring its openness in Delta format and the Spark engine. If Lakehouse IQ can prove invaluable as a free add-on, it will save time and money for organizations trying to train their own model. On the other hand, there also doesn’t exist a single tool that has integrated both data and its governance. The generosity of Databricks will definitely help organizations. Remember, Microsoft’s Purview is both a separate and a premium tool.
Query federation
This capability is not a breakthrough per se. SQL Server’s linked server has long allowed users to access other RMDB using ODBC/JDBC drivers and recognize it as part of the system.
I have written an article on how to use Spark to accelerate data movement. As we can see from the documentation, it is still using ODBC/JDBC. However, with Databricks’ Photon engine, it will accelerate the write-into-Parquet. There is some magic in Photon but not in query federation. The whole point of having query federation is, once again, data governance. Right now, despite we can move the data from the source system with Spark JDBC and photon without query federation, the lineage is often missed and is not standard across teams. So once again, it will be beneficial for data governance, audit, and upstream troubleshooting.
Uniform
In my previous post, I also discussed about the format war that nobody was talking about. But Databricks secretly worked on Uniform, to create a framework to integrate Delta, Iceberg, Hundi, and beyond. On the other hand, Snowflake continues to enhance its support on Iceberg called Snowflake Iceberg. This is yet another important data governance effort by Databricks, where they want to unify all data into one place by offering support from the DMBS level and data format level.
LLM
Mosaic ML
As people use LLM like ChatGPT daily, few realize that we cannot train an LLM from scratch by spinning up a data science virtual machine or even on compute cluster. So, what are the options for organizations that want to train a model with their data?
According to a Weights and Biases whitepaper, there are three options:
- Use the API of a commercial LLM, e.g., GPT-3 (OpenAI, 2020), Cohere APIs, AI21 J-1.
- Use an existing open-sourced LLM, e.g., GPT-J (EleutherAI, 2021), GPT-NeoX (EleutherAI, 2022), Galactica (Meta AI), UL2 (Google, 2022), OPT (Meta AI, 2022), BLOOM (BigScience, 2022), Megatron-LM (NVIDIA, 2021), CodeGen (Salesforce, 2022).
- Pre-train an LLM by yourself or with consultants: You can either manage your own training or hire LLM consultants & platforms. For example, Mosaic ML provides training services focusing on LLMs.
Companies will likely use a mixture of #2 and #3 as their solutions, as most organizations are not willing to send their data outside their VPN for LLM or risk leaking their confidential information. But on the other hand, they also don’t have the skills required to train an LLM, not to mention setting up the infrastructure. So they will hire consultants to employ tools like Mosaic ML to train an LLM. We can look at it from the cost and tooling perspective.
Cost
Despite it is said that Mosaic ML is capable of training an LLM at a fraction of the cost, Nvidia also made similar announcements that they are capable of training one LLM at a similar amount of cost. While we don’t know if they are talking apple to apple, but less than half a million dollars is what they are targeting. In other words, Mosaic ML might just be taking advantage of what Nvidia GPU has to offer and optimized it with a unique infrastructure.
Infrastructure
On top of their open-sourced models, MosaicML is also a platform. According to a case study from Oracle, MosaicML’s Training Runtime uses the following major components to train state-of-the-art LLM:
- Composer training library: Tested, high-performance, scalable training framework
- StreamingDataset library: Fast, efficient data loading from any cloud object storage
- Training Docker images: All necessary software packaged as Docker images
Just to imagine the number of hours required to set up the above infrastructure and maintain it. It will easily cost organizations a lot of money to hire and retain talents to train these models significantly. However, readily available infrastructure will make it easier for organizations to train and deploy their models.
While the Snowflake vs. Nvidia partnership might be a similar offering or even be potentially even more powerful, MosaicML offers a more flexible architecture and is also battle tested.
Reference: https://www.mosaicml.com/blog/build-ai-models-on-any-cloud-in-your-secure-environment
LLM Ops
The importance of https://www.tredence.com/MLOps-101ML Ops in traditional machine learning is undeniable. It is even more important in LLM because the time and cost can be much more than training a churn model. On top of model management, cost management will also become standard in LLM Ops.
Lakehouse AI illustrates the AI platform that Databricks is building on top of the Unity Catalog.
Reference: https://www.databricks.com/blog/lakehouse-ai
MLflow 2.5
The latest MLflow version is yet to be released in early July 2023, but there are two main new features:
1) AI Gateway : LLMs will only make sense if we wrap it up in an application like ChatGPT; this way, it would be more user-friendly than expecting the average user to navigate Python within a Databricks notebook. Do note that LLM is available in two formats: Commercial (SaaS) and Open Source. And the newly proposed AI Gateway aims to capitalize on these effective LLM integrations and is guided by the following: 1)
- Credentials management – As the commercial LLM options grow, organizations will be required to manage API keys securely so it is not exposed to the general public.
- Query routes – It provides an abstraction of the interaction with the LLM. Different LLMs will have different access points to generate answers for the questions; by having an abstraction not only it will make it easier for users to test various models, but it will also make application development easier.
- Security – Limiting how often each user or application can query a given route will facilitate cost optimization and prevent unauthorized access to sensitive data. For example, if an LLM is trained on HR data for authorized HR use, we don’t want to expose it outside of HR.
2) Prompt tools: Also known as MLflow evaluation, the new function mlflow.evaluate() provides a way to evaluate the performance of an ML model, including but not limited to LLM. In particular, the question-answer model type provides a default evaluation type as an exact match; in other words, it requires a specific answer to be known beforehand.
Additionally, there is a no-code interface to compare the results of the prompts from different models. While this is a very nice debugging tool, it is by no means an acceptable way to evaluate an LLM.
According to the whitepaper from Weights and Biases, it suggested ten different ways to evaluate an LLM as well as suggesting an open source framework EleutherAI to do a systematic evaluation. The MLflow evaluation is only scratching the surface. Similar to the AI gateway, Databricks is leaving developers to implement their solutions via a standardized interface.
Auto ML
1) Curated models: Curated models in Databricks represent two things. First, they picked some state-of-the-art yet truly free-for-commercial-use models. They go from quality-optimized with up to 40 billion parameters to some large models with 7 billion parameters to some smaller models for performance purposes. The first one, Falcon 40B, is on the top of the hugging face Open LLM leaderboard. Secondly, Databricks would fine-tune the models and pre-load them on the platform. That’d save the serving cost. For example, Falcon 40B is 80 gigabytes in size.
2) Auto Fine-tuning: Foundation models are already developed with 90% to 95% of the general knowledge. If we want the LLM to understand specific instructions, we need to fine-tune it with internal datasets. There are generally three types of tuning: prompting, instruction tuning, and reinforcement learning through human feedback (RLHF). It is unsure which method Databricks supports at this moment. It is worth noting that Databricks’ traditional AutoML does not allow choosing which model to train, nor would it train on the full dataset when a huge one is given. As such, if we know for sure that some models will train faster, there is no way for data scientists to ask AutoML to tune one particular model, so it can also be wasting compute. We will see if these restrictions are lifted in the AutoML for LLM.
Monitoring
Lakehouse monitoring is a monitoring solution built on top of the Unity Catalog. Once again, it relies on the dependencies in Unity Catalog to automatically build relevant dashboards. The monitoring capabilities span from data quality to data drift to model monitoring. It is by no means comprehensive but its ability to capture insights and automatically set up dashboards has already provided vast improvements for many organizations that don’t have the expertise to set up a solution. It also comes with automatic alerts and custom metrics for advanced users. It greatly simplifies the time to insights for the entire AI pipeline.
Snapshot profile – It is designed for basic quality metrics for any table at a snapshot in time. While Delta Live Tables (DLT) comes with basic expectations, the snapshot profile is similar to the data profiling function in dbutils for exploratory data analysis over time.
Time series profile – This isn’t talking about a time series forecast but rather determining data drift occurrence given a time stamp column. The drift analysis metrics include:
- Chi square test
- Tv distance
- I infinity distance
- Js distance
- Numeric
- Ks test
- PSI
- Wasserstein distance
Inference profile – This is designed to measure classification and regression influence results like precision and recall as well as R2 scores. Currently, data scientists need to maintain the codebase to calculate these numbers, and each team will try to use a different library depending on what model they are using. Databricks’ approach is model agnostic and is calculated completely based on an inference table. On top of that, it includes fairness and biases – which is a baby step forward to Responsible AI. The metrics include: predictive parity, predictive equality, equal opportunity, and statistical parity.
Microsoft’s Responsible AI standards
All of the above profilers are capable of detecting PII in the data using AI, sensitive columns will be tags as PII in the catalog.
Below is the vision of the monitoring framework from Databricks, which covers all the way from the Delta Live table to the inferencing table:
Vector search
Every AI organization is announcing a vector search. For example, Microsoft also announced the Bing Index service in Azure AI Studio. This is rather an unusual yet technical naming of the product/feature. Perhaps it is already an easier term to understand than “embedding,” but it is still a hard concept to grasp.
Vector, or embedding, results from some complex “encoding” algorithms from deep learning. These algorithms encode an image or a chunk of text into a multi-dimensional vector in the form of matrix. Documents or webpages will be broken into chunks and stored in a high-performance, low latency database, aka Delta Table, on top of serverless in Databricks backend. When a question is asked, or some text is being searched, a similarity (aka dot product between two vectors) is compared with top k (k can be 10 to 100 depending on the number of results desired) records in the embedding table. Imagine searching on a very large text corpus (e.g., the entire Wikipedia); if the database is not fast enough, it will take an eternity to return any meaningful results. That’s why every AI company highlights this capability as a standalone product, not a simple feature.
Retail Applications
Similarity search can also be used in Retail, where Graph Neural Networks have long been able to learn the embeddings of a user based on their purchase history. The sections on Amazon like “similar item to consider,” “frequently brought together,” and “customers also consider these items” are the results of similarity search based on user or item (product) similarity.
Model Serving
Historically, once a model is developed, it is saved as binary and curated for later use. Databricks has had a model registry for a while and is part of ML Ops. But to use the models easily and abstract the model out from any clusters, model serving is created using serverless; it supports both CPU and GPU, curated models, or MLflow models. It also supports an automatic inference table that automatically writes the prediction output to a delta table. It might sound trivial, but it is an extra step for every model development, and keeping track of where the inference table is located can be a challenge. Now, Unity Catalog can keep track of everything from end to end.
Here’s an end-to-end architecture, not limited to LLM.
Cleanroom
Data cleanroom has been in Snowflake for about a year now. , along with added privacy like encrypting sensitive PII columns.
Databricks did not just create another copycat of data Cleanroom. A unique advantage of Databricks’ approach includes:
- Nothing to hide – Delta sharing is an open source and adheres to open standards. If Databricks is afraid of the cleanroom data getting hacked, they would be strongly against opening the framework to the world.
- Backed by the world’s most trusted partner – Cloudflare is probably one of the most trusted companies in the world on internet security and is the backbone of the Internet. Delta sharing is partnering with Cloudflare to securely distribute its data; vendors can rest assured that the data-sharing experience is safe.
- No replication – With open standard comes open implementation. Delta sharing can share data with different vendors and across regions without replication.
- No vendor lock-in – Due to security and private standards, all data cleanroom solutions must be shared within their platform; whether distributed or not, they must be within the same region and data cloud. Delta sharing is an ecosystem that allows sharing of data to vendors who decide to implement it.
- Not just SQL – Delta sharing supports all languages run on top of Spark, aka Python, R, SQL, Java, and Scala. Data providers will have more flexibility in terms of data transformation.
- Unity Catalog support – Delta sharing in Databricks can also take advantage of the added fine-grain control provided by UC.
And Oracle is the latest big name using Delta sharing for data exchange. It also enabled legacy DMBS to a new possibility.
Data marketplace
Backed by Delta sharing, the data marketplace allows users to subscribe to data from trusted providers. However, it is still an immature feature that doesn’t support financial transactions within Databricks. Any contracts and agreements will need to be done outside of the platform before the data can be shared. Nevertheless, the ability to share using any language means providers can now share along with code examples in multiple languages for advanced usage. This can be attractive to advanced users.
English SDK
English SDK was announced in the second day’s keynote and received a lot of interest. The promise of English SDK is to be able to write code in English inside a Databricks notebook. It can generate queries,
It might sound similar to Lakehouse IQ. But under the hood, if we look at the source code from , we know that it is merely depending on an LLM, like GPT-4, to understand the English and generate the query. In other words,
As a developer, this is something we do all the time, but it is a bit manual. The steps are below:
- Create a data set – Yes, it is a requirement, or the SDK won’t work at the moment
- Come up with a question for the data set
- Download the schema and tell LLM the exact columns and schema
- Ask LLM the question and execute the query
While these steps are tedious, we often get good results, as reported by many news outlets. English SDK, as of now, is automating exactly as above. But as the interest grows, we will see how the community will evolve the use cases. It can become very useful if an organization can run a private code-gen LLM that is good enough to handle most of the cases; it will eliminate some repetitive tasks and empower business users at the same time.
However, despite all the promising curated models from Databricks, the SDK team still recommends using GPT-4 after extensive testing.
As of June 2023, our extensive testing suggests that the most effective utilization is with the English SDK and GPT-4.
Date warehousing
Databricks/Spark (as well as the big data community) adopted an open format originally created by Hadoop called Parquet, released in 2013. Parquet’s columnar format (vertically) allows aggregation of data faster than traditional databases (where data is stored at row level, horizontally). But as the demand for data warehousing and ACID transactions increases, Databricks must come up with a new standard yet continue to be able to work with Parquet.
Compared to Snowflake’s closed format, Databricks’ commitment to open source leaves them with little choice but to continue innovating on top of the Parquet format. Similarly, Snowflake is also trying to innovate on top of Iceberg, another format based on Parquet. According to an independent study by Hitachi Solutions, Databricks’ performance exceeds Snowflake’s.
Serverless
Snowflake users have long been enjoying instance startup time (3-4 seconds); Databricks’ is definitely considered as playing catch up in this case. The difference between serverless and deploying clusters is that users will always pay for the monthly VM costs once it is allocated in the Databricks cluster, and all Databricks does it to deploy the runtime on top of the machines and be able to distribute the workload and only when we run the workload, DBU or Databricks Unit, will start coming into the picture as the total cost of ownership.
Serverless, on the other hand, charges per usage only without the VM cost. Databricks had to come up with even more optimized algorithms to manage the servers. Otherwise, the cost would be out of control.
Fortunately, for Databricks, there are more ways to optimize the cost of serverless due to its AI ability. For instance, Model Serving and Delta Sharing can now operate using serverless and integration with reporting tools like Power BI and Tableau. These are all opportunities to utilize the VMs with a return on investment.
As of now, Databricks’ serverless startup time is about 7 seconds, but they aim to cut it down to 3 seconds.
Liquid Clustering
Goodbye partitioning, hello clustering. The AI world talks a lot about similarity because we recognize objects, text, and voices by their familiar shape and form. In terms of data, there is no difference. Clustering has long been a machine-learning technique to group similar data points together. When we group similar data, the need to shuffle data across the network is reduced (aka sorting). Historically, this is done using partitioning and z-ordering.
By using AI, Databricks is now able to allow developers to re-cluster the table with minimal re-writing. The ability to change clustered columns at will is why it is called “Liquid” clustering.
Liquid clustering does not require partitioning anymore and will use AI to handle automatic data grouping.
To manage delta lake upgrades, please refer to this guide by Databricks.
Predictive I/O
Another AI feature by Databricks is to speed up read and update operations in a delta table using the Photon engine. Predictive I/O leverages deletion flag (or vector) to optimize the update performance. It is a tradeoff between re-write (compute) and storage. According to Databricks, predictive I/O is comparable to Snowflake’s Search Optimization Index, but this index comes with a cost. Predictive I/O (as well as Photon) is on by default in DB SQL Pro and Serverless and works with no added cost.
The drawback of the deletion vector-enabled table is that it cannot be shared via Delta sharing.
Streaming/Project Lightspeed
Streaming is not just a game for IoT; nowadays, many real-time apps also require streaming data support. Databricks gave an update on Project Lightspeed to make it a more open platform. The goals of the initiative include:
- Improvements in performance and providing consistent latency
- Enhanced functionality for processing data
- Improvements in observability and troubleshooting
- Ecosystem expansion with new connectors
Lightspeed’s notable achievement is 3x performance improvements with a max of 250 ms latency on 1M events/s. Comparatively, there’re only, on average, 11k messages being sent on Whatsapp per second.
Conclusion
With Databricks' unified toolset, there is no better time to build our Gen AI solutions on Databricks. We no longer need to worry about the different licensees and tooling, and we can also be assured that Databricks will continue to invest in the tooling and giving away for free. Along with the open standards - Apache Spark, Delta format, and MLflow continue to be governed by the open source community, practitioners can continue to innovate in a safe environment.
About the author:
Jason Yip is a Data and Machine Learning Engineer and Director of Data and AI at Tredence, Inc. He helps Fortune 500 companies to implement Data and ML Ops strategies on the cloud, including T-Mobile, Clorox, and Microsoft. He serves on the Product Advisory Board at Databricks as well as a Solution Architect champion, making him the top 1% Databricks expert in the world.
Jason also uses his programming skills for philanthropy, where he started lite.chess4life.com, an online chess learning platform used by hundreds of thousands of grade school students across the USA as well as a founding member of Robert Katende Initiative, home to the Disney movie Queen of Katwe. Jason also publishes technical articles in datasciencecentral.com and Analytics India Magazine.
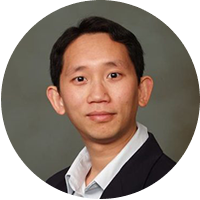
AUTHOR - FOLLOW
Jason Yip
Director of Data and AI, Tredence Inc.
Topic Tags