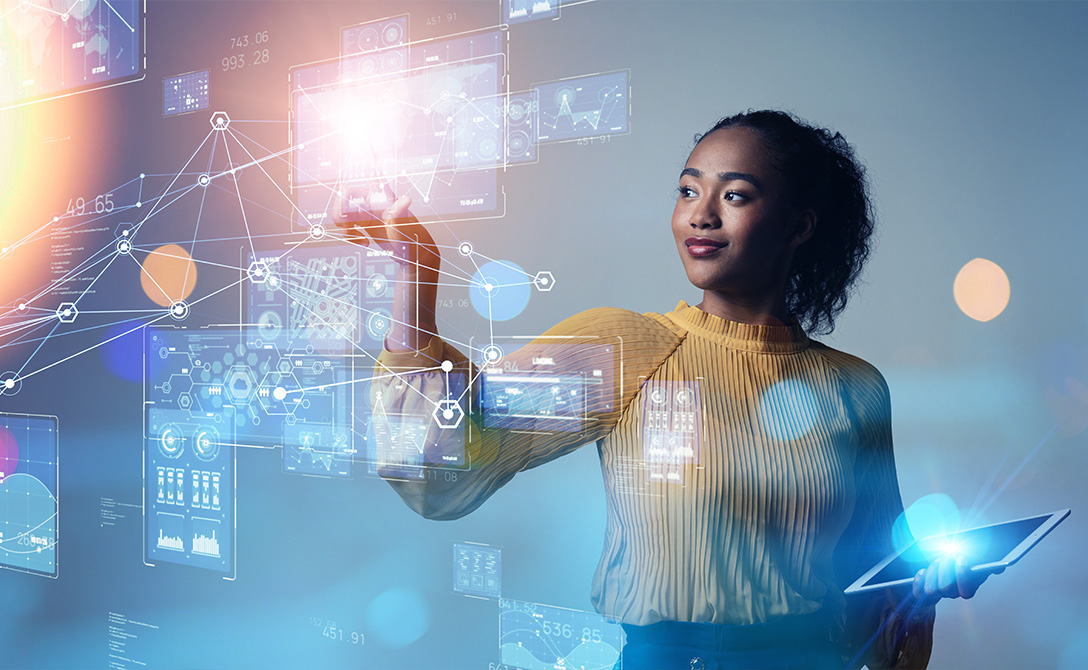
The demand for data scientists has skyrocketed across industries over the past few years, making the field highly competitive for students and employers. The entry of Industry 4.0 technologies and the acceleration of digital transformation efforts have further made data science a priority for future-forward business leaders, who now look for teams that can not only deliver insights but also engage in targeted value engineering projects that guarantee ROI and transformative impact. The pandemic has profoundly impacted the data science industry. One of the most immediate effects was a loss of trust in predictive modeling. For years, data science has relied on past data to predict future trends. However, the pandemic showed that the past is not always a reliable predictor of the future. As a result, many organizations lost faith in data-driven decision-making. Another significant impact of the pandemic was a shift in focus from predictive analytics to descriptive analytics. In the short term, organizations were more concerned with understanding what was happening in the present moment than with predicting future trends. This increased reliance on tools like real-time dashboards and streaming data analytics. The pandemic taught us to be prepared for black swan events in the future. Organizations must have contingency plans in place in case traditional models fail. Furthermore, data scientists must be able to explain their models to non-experts. Only by regaining trust can data science continue to thrive in the post-pandemic world.
Following are 5 key trends that are likely to shape the future of the field.
1. Shift of reliance to external data
While large quantities of data are being gathered and processed by data science teams to build predictive and prescriptive models, the need of the hour is to choose the right kind of data. The pandemic demonstrated that internal data cannot always be a reliable source of predicting future outcomes since external environments are often dynamic and volatile. As a result, businesses are affected by myriad external variables in quickly changing market environments – from competition activity to consumer behavior patterns. External datasets are often publicly available, such as government resources and published market studies, and firms now realize the importance of incorporating these into their data science practices. According to a 2019 Deloitte survey, 92% of analytics professionals said their companies need to expand their use of external data sources, and this trend continues in the post-pandemic world.
2. The resurgence of descriptive analytics
As the name suggests, predictive analytics derive insights from historical data and patterns for predicting future events. While this continues to be important for businesses to preempt and forecast market conditions, we are also witnessing a resurgence in descriptive analytics. As firms deal with increasingly large datasets, descriptive analytics enable the streamlining of the data analysis pipeline and reveal unique insights and trends that cannot be found through other analysis methods. So, we are beginning to see the value in integrating both methods to yield actionable insights.
3. The rise in disrupted industries investing in AI/data science
The pandemic upended existing practices across all industries, with certain impact sectors like logistics and health care nearing collapse. However, every crisis is an opportunity for disruptive innovation. We are witnessing a dramatic acceleration of digital transformation in these sectors, which are now increasing investments in emerging technologies like AI and data science to better preempt, predict, and adapt to changing conditions. A recent report by Bain & Company, Microsoft, and IAMAI revealed that 87% of Indian firms would hike AI spending by 10% in the next three years, with the same report also indicating that around 80% of enterprises in India have a minimum of one AI model in production. Thus, we can expect extensive penetration of these technologies across sectors.
4. Data explosion due to surge of digital users
The ‘Digital India’ movement has created a steep upward trajectory in the number of digital users, resulting in a rapid and exponential increase in the quantity of data generated. Big data is constantly getting bigger, and the conflation of the physical and digital worlds in the metaverse will amplify this trend to a great degree. Today, consumers interact with various online touchpoints through different platforms, creating large and varied datasets. On the one hand, businesses must capitalize on this opportunity by improving data science maturity and investing in the right data infrastructure and analysis tools. On the other hand, robust security measures are essential to prevent data breaches in a data environment with multiple entry points.
5. Marrying model predictions to business outcomes
When it comes to data science, top business leaders often struggle to understand what their insights are worth. In this context, insights generated from model predictions should be viewed as merely the first step, not the result. Real business transformation moves the needle from insight generation to value realization. Real business transformation moves the needle from insight generation to value realization. Model predictions should be used to answer questions such as ‘Which regions have the most demand for the solution that we are offering,’ ‘What is the current state of demand fluctuation?’ and ‘What are our target customer’s current demands and expectations?’ This, in turn, can be used for short-term outcomes (such as increasing sales, optimizing processes, reducing immediate risk, etc.) and long-term, transformational impact (such as entering new markets, reducing time-to-market, and unlocking new revenue pockets.) Overall, the post-pandemic world is an exciting time for the data science industry. Making the right decisions can convert each of these trends into lucrative opportunities for growth and transformation. R&D teams across the country are working on more robust models and better model accuracy to improve the richness of predictive analytics and generate better ROI from data science investments. We see increasing importance given to explainable AI and AI ethics to improve business leaders’ trust in models. While innovation in the field is promising, adoption must keep pace with the developments in the field. We are seeing a good rise in business model adoption, with more pilots and POCs moving into production. Overall, forward-thinking leaders must expand the boundaries of data science and use business-centric innovation to go beyond the possible and make the most of these opportunities to stay ahead of the curve. (The author is Mr. Aravind Chandramouli, Head- AI COE, Tredence, and the views expressed in this article are his own)
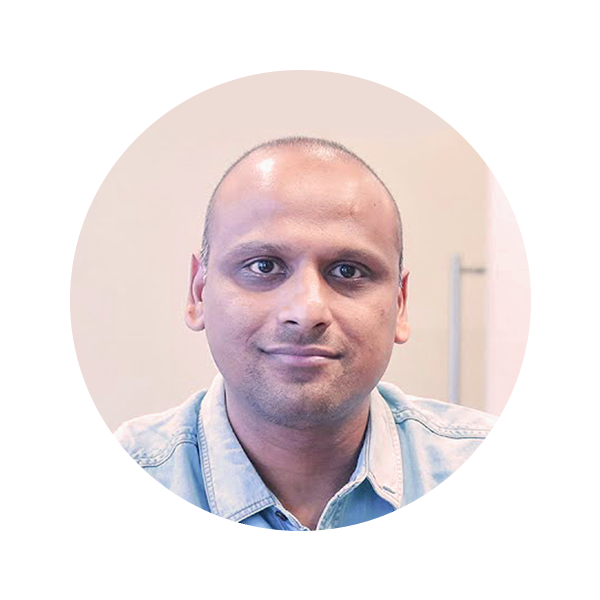
AUTHOR - FOLLOW
Aravind Chandramouli
Head AI COE, Tredence
Topic Tags
Detailed Case Study
Driving insights democratization for a $15B retailer with an enterprise data strategy
Learn how a Tredence client integrated all its data into a single data lake with our 4-phase migration approach, saving $50K/month! Reach out to us to know more.
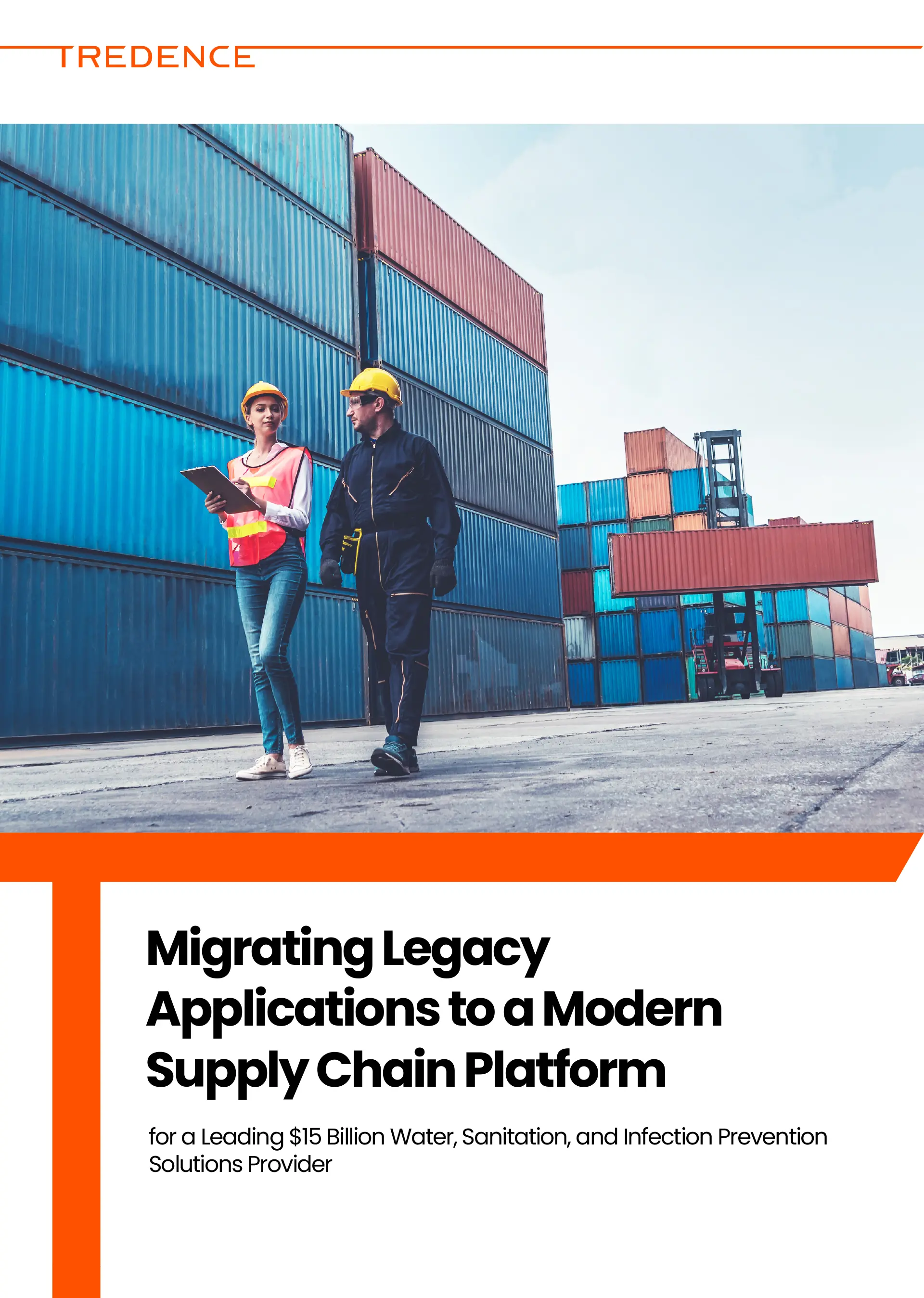
Detailed Case Study
MIGRATING LEGACY APPLICATIONS TO A MODERN SUPPLY CHAIN PLATFORM FOR A LEADING $15 BILLION WATER, SANITATION, AND INFECTION PREVENTION SOLUTIONS PROVIDER
Learn how a Tredence client integrated all its data into a single data lake with our 4-phase migration approach, saving $50K/month! Reach out to us to know more.