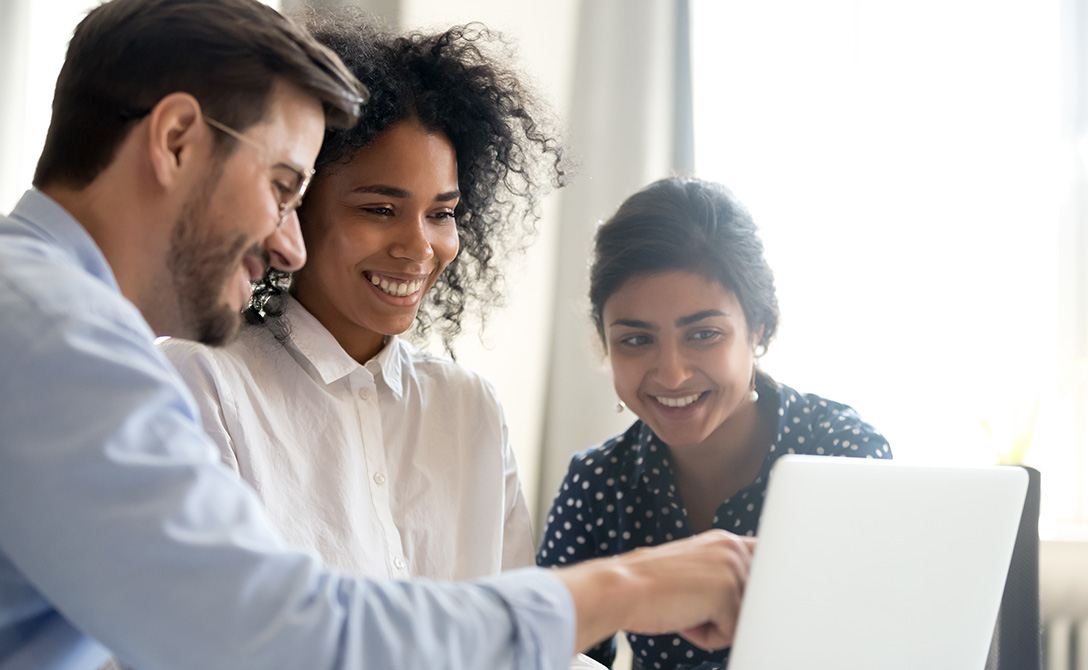
AI has become the pillar of growth for companies when it comes to maintaining relevance as well as an edge over the competition. What’s more, AI based models have become the new revenue drivers for companies looking to capitalize on data as a competitive advantage. The rise in algorithmically driven successes can be attributed primarily to enhancements on the hardware side. Big data tools, and an infrastructure based on both on-premise and cloud services, have paved the way for this fully evolved AIML ecosystem.
According to a study, AI is the next digital frontier and organizations that leverage models have a 7.5% profit margin advantage over their peers. With AI models becoming the key pillar for building valuable IP and revenue, Tredence shows the way with a new approach to model management.
With more research being plowed into tweaking neural networks, businesses face a bunch of tricky questions-how profitable it is to go full ML? Is the available compute infrastructure sufficient enough to take the leap? Can the deployed model adjust to the changing grounds and business requirements?
From training personnel to acquiring tools, business leaders are also grappling with critical questions related to model management — model validity in the face of changing business realities. Models lose validity over time as market realities change, new contingencies emerge, and new variables come into the picture. Hence all models need to be revamped and refreshed regularly to ensure they remain relevant. However, the refresh process is often manual and possesses a lot of scope for improvement.
Tredence employs machine learning algorithms to develop analytics solutions for its customers. Our solutions range from providing prediction frameworks for online retailers in the US to cutting costs for manufacturers of thermal insulation materials. We are embracing a factory approach to building AI models.
Need For A Move to a Factory Approach
There are multiple reasons models need to move to a factory approach. Setting up models for the first time is a highly ad hoc process which is over-dependent on the skill of the data scientist building the model. The process is also highly susceptible to human biases and is very labor intensive. Model refreshes, on the other hand, are reactive and end up following a blind process, and remain labor intensive.
The term ML model refers to the model artifact that is created by the training process. The training data must contain the correct answer, which is known as a target or target attribute.
The learning algorithm finds patterns in the training data that maps the input data attributes to the target (the answer to be predicted), and it outputs an ML model that captures these patterns. A model can have many dependencies and to store all the components to make sure all features available both offline and online for deployment, all the information is stored in a central repository.
The new set up for a model factory approach should start with a strong clarity about the business requirements and environment. When building the model for the first time, the bounds for the model should be clearly defined, and the best model identified. If necessary, an ensemble of multiple models should be used. A good model can be identified basis multiple criteria, such as quality metrics, cumulative gains, heat maps, bootstrapping methods and other techniques.
The model refresh process should go through the following steps:
- Define frequency of refresh, as well as exception conditions under which an out-of-turn refresh must be done
- Define when the refresh will occur – is it when the current scenarios repeat, or when new scenarios emerge
- Automate the refresh process, with clear bounds of the process defined. Data collection, splitting the dataset into training and validation samples, running the models, and validating and analyzing them for accuracy, are all steps than can be automated.
Importance of the AI-human Interface
The ultimate goal of any AI research is to derive insights about the business. Highly accurate AI models are usually harder for a human (especially a non-data scientist) to interpret, so the right model which balances accuracy vs. interpretability should be deployed. Since the eventual value of a model lies in its usage by business teams to meet targets or achieve goals, human review and understanding of models is essential. The model factory is intended to save human time in refreshing models through automation. This human time can in turn be used to analyze and derive the right insights from the mode results.
Since humans are the ones to benefit from this, there is a rising need for the augmentation of AI. Tredence has developed augmented intelligence solutions like Word Craft, Sancus and DCC (Digital Content Categorizer). For instance, Sancus is a machine learning and deep learning-based data management solution that aims to deliver reliable data to businesses.
Future Direction
Traditional data storage and analytic tools can no longer provide the agility and flexibility required to deliver relevant business insights. An AIML based factory model approach augmented within human intelligence can help organizations overcome and maintain competitiveness and relevance. Organizations seeking transition to an AIML based model factory setup can get an idea of how to scale by looking at Tredence’ s approach.
Topic Tags