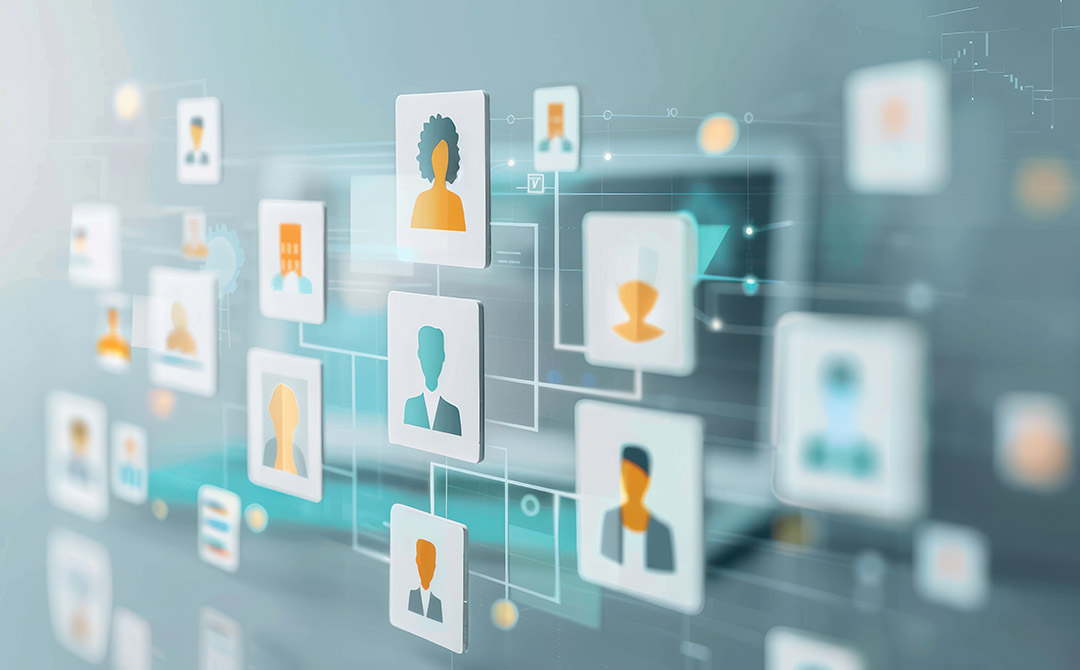
Customer data platform (CDP) effectively supports organizations' efforts to achieve a unified, 360-degree view of their customers. It helps mid- and large-scale organizations organize data collected from multiple sources, including websites, in-store visits, email interactions, and app usage.
The global CDP market is likely to surge at a CAGR of 39.9% from 2024 to 2028 and reach USD 28.2 billion by 2028 (Source: Markets and Markets).
Thus, CDP is likely to be a game-changer for organizations; however, static processes and the need for manual intervention are hampering its performance. AI CDP is a promising development that combines data management with automation.
AI CDP promises the next innovation in data-driven decision-making, from hyper-personalized marketing campaigns to highly optimized customer journeys.
What is AI CDP
AI CDP refers to a software solution that consolidates and manages data from various sources and integrates AI to uncover patterns and derive meaningful insights in real time. This enables businesses to optimize various facets of customer engagement, from marketing strategies to personalized communication.
Evolution of CDPs with AI Integration
The integration of AIs in CDPs has been in the making for a long time. By 2017–2018, AI integration started to redefine CDPs' capabilities. This period marked the beginning of machine learning in these platforms.
AI could no longer just collect data but analyze it and provide actionable insights. It could also offer predictive analytics, enabling businesses to anticipate customer needs and personalize experiences at scale. This is when CDPs began their journey toward intelligence-driven platforms.
In the 2020s, generative AI and advanced algorithms created further sophistication in CDPs. Real-time automation in these platforms involved managing data quality, monitoring compliance, and hyper-personalization.
AI-driven CDPs handle massive amounts of data without compromising compliance under GDPR or CCPA.
Going forward, businesses will need AI-driven CDPs to implement data-driven strategies and engage customers with personalized experiences to gain a competitive edge.
Role of Artificial Intelligence in CDPs
Of late, AI has become a crucial component of customer data platforms, enhancing their ability to collect, analyze, and utilize customer data effectively.
AI enables CDPs to process large amounts of data from various sources, creating comprehensive and accurate customer profiles. This helps businesses gain a deeper understanding of customer behavior and preferences.
AI also enables CDPs to predict customer behavior using machine learning algorithms that analyze past interactions and transactions. These predictive capabilities enable the creation of more targeted outreach strategies and offerings. Additionally, AI-based CDPs can automate marketing processes, thereby reducing manual efforts and increasing operational efficiency.
CDP vs AI CDP
Customer data platforms have long served organizations well. However, as data complexity grows and customer expectations rise, businesses need more advanced CDPs.
Let us understand the differences between CDP and AI CDP in detail:
Aspect |
Traditional CDP |
AI-powered CDP |
Overview |
A platform that collects and unifies customer data from multiple sources into a single customer profile. |
A platform that combines data unification with AI to provide predictive analytics, automation, and actionable insights. |
Data Handling |
Primarily focused on collecting, organizing, and integrating customer data. |
Enhances data handling by cleansing, deduplicating, and analyzing data using AI algorithms. |
Segmentation |
Rule-based segmentation requiring manual input. |
AI-driven segmentation using machine learning (ML) to identify patterns and create predictive groups. |
Personalization |
Limited to basic personalization, such as grouping customers into predefined segments. |
Real-time, dynamic personalization based on customer behavior, preferences, and predictive analytics. |
Scalability |
Suitable for managing moderate volumes of customer data but may struggle with large-scale operations. |
Highly scalable; can process and analyze vast amounts of data in real time. |
Real-time Insights |
Insights are often static or delayed due to manual reporting processes. |
Provides real-time insights and triggers for marketing actions using predictive analytics. |
Automation |
Limited automation, relying on pre-set workflows. |
Advanced automation capabilities, such as auto-triggering personalized campaigns and recommendations. |
Customer Journey Mapping |
Relies on static journey mapping tools; requires manual updates. |
Continuously refines customer journeys based on AI-driven behavioral predictions and trends. |
Use Case Examples |
Data integration, basic marketing segmentation, and analytics reporting. |
Predictive lead scoring, dynamic campaign optimization, and hyper-personalized customer engagement. |
Data Privacy and Compliance |
Primarily ensures compliance through data organization and tracking. |
AI tools help automate compliance checks, ensuring data privacy across complex ecosystems. |
Implementation Complexity |
Easier to implement but offers limited advanced functionality. |
More complex to implement due to AI models but offers superior long-term benefits. |
Cost |
Generally lower cost due to simpler features. |
Higher cost due to AI integration and advanced processing capabilities. |
Key Limitations |
Limited to descriptive analytics; cannot predict future behaviors or automate high-level decision-making. |
Requires high-quality data and significant resources for effective implementation and maintenance. |
Traditional CDPs are ideal for basic data unification and segmentation, while AI-powered CDPs leverage advanced algorithms to enhance personalization and automation. AI-driven platforms offer significant benefits in terms of real-time insights and predictive analytics despite the higher complexity and cost.
Benefits of Implementing an AI CDP
Implementing an AI-powered Customer Data Platform (CDP) offers benefits beyond traditional ones, offering capabilities that improve businesses' interactions with data.
AI CDPs empower organizations to elevate customer engagement and operational efficiency by unlocking new avenues for precision, automation, and foresight.
Enhanced Multi-channel Attribution
AI CDPs leverage advanced attribution models to provide a deeper understanding of how different channels contribute to conversions. Unlike traditional CDPs, AI-powered platforms optimize resource allocation across marketing campaigns, ensuring a higher return on investment (ROI) through predictive insights and real-time adjustments.
Continuous Data Improvement
AI-powered CDPs use self-learning algorithms that improve data quality over time. They automatically detect anomalies, fill in missing values, and adjust to changes in data sources. This ensures that organizations always work with up-to-date and reliable data, leading to better decision-making and less reliance on manual data cleansing processes.
Proactive Customer Health Monitoring
AI-powered CDPs analyze engagement signals such as customer sentiment, inactivity patterns, and service feedback to identify at-risk churn proactively. Early identification helps you roll out targeted retention strategies, resulting in sustained loyalty. This helps reduce attrition levels and boost long-term relationships with customers.
Predictive Capacity Planning
AI CDPs can forecast resource requirements based on trends in customer interactions, demand spikes, and seasonal patterns. These predictions help you optimize staffing, inventory management, and operational efficiency. Proactive planning also helps you reduce downtime, avoid overstocking or understaffing, and ensure readiness for future demand surges.
Thus, combining AI with CDPs enhances the capabilities of both technologies, enabling businesses to quickly adapt to changing customer behaviors and evolving market conditions.
Challenges in Adopting AI CDPs
The adoption of AI-driven CDPs entails certain challenges. Fragmented customer data spread across different silos poses a significant barrier to seamless integration. This leads to low-quality data, impacting the performance and creating inaccurate insights.
Another challenge is the complexity of implementation and overall cost. Deploying AI-powered CDPs requires advanced technical expertise, infrastructure upgrades, and significant financial investment, which can be intimidating, especially for small—to medium-sized enterprises.
Integration with existing systems and tools often demands tailored solutions, further increasing complexity and expenses.
Finally, privacy and regulatory compliance issues deter companies from leveraging AI CDPs. Data privacy is a major concern, requiring strict regulations in the handling of customer information. Organizations are hesitant to adopt AI-based systems that are not fully compliant with regulations.
Use Cases of AI CDP
AI CDP has the potential to benefit organizations across a range of industries. Although its adoption is still in its infancy, it holds immense potential as AI adoption grows.
63% of Chief Technology Officers (CTOs) said that their generative AI budgets increased in 2024 compared to 2023, while 24% said they doubled or more. Additionally, 31% of CTOs said their budgets rose by up to 99%, while 36% said they remained flat (Source: Softbank Vision Fund).
Let us take a look at some of the most prominent use cases of AI CDP:
Predictive Customer Segmentation
Traditional CDPs use rule-based systems to collect data from various sources. This helps in seamlessly creating unified customer profiles; however, rule-based systems have certain limitations. They fail to account for inconsistencies in data, such as missing fields or name variations.
On the other hand, AI CDPs use probabilistic matching and ML models to analyze complex patterns in data. They operate in real time and continuously update with new data as it arrives, ensuring accurate and dynamic customer segmentation.
Starbucks uses AI-powered customer segmentation to incentivize customers to visit its stores more often and spend more during each visit. Its Deep Brew program helps create customizable menu boards that use AI to suggest items based on various factors. These factors include weather, time of day, store inventory, popularity, community preferences, and the customer’s purchase history, all of which aim to identify customer cohorts (Source: PYMNTS).
Best Action Recommendation
Unlike rules-based systems, AI dynamically evaluates an individual's online behavior, preferences, and historical trends and recommends personalized offers, communications, or services. This capability helps you engage with customers in a more relevant and timely manner, ultimately driving conversions and loyalty.
A telecom company may leverage AI to analyze customer usage patterns, complaints, and tenure. AI may suggest upgrading a data plan for a heavy user or offering a loyalty reward to a long-term subscriber who indicates dissatisfaction. Customizing offers to meet individual needs allows the company to boost customer satisfaction and minimize churn.
Netflix, a global streaming giant, offers recommendations to users based on their preferences and viewing habits. With nearly 300 million subscribers, manually offering highly personalized content recommendations is not feasible. To deliver this at scale, Netflix uses AI and ML algorithms to recommend the right content to the right user (Source: CDP).
The benefits of AI CDP in best action recommendation are significant: hyper-personalization creates stronger connections, predictive insights trigger proactive engagement, and automation empowers operational efficiency. For instance, e-commerce retailers can suggest more relevant upsells and ‘you may also like’ products based on browsing and purchase history.
A set of AI-powered best action recommendations can transform engagement by predicting customer needs and suggesting the most enjoyable and relatable actions.
Churn Prediction and Retention
AI-powered CDPs can facilitate churn prediction and retention strategies by analyzing and acting upon customer data. These platforms use ML models to identify behavior patterns and churn signals, such as low engagement, reduced spending over a period of time, and frequent complaints.
For instance, an AI-based CDP can analyze factors such as usage frequency, customer support tickets, and past transaction history to assign a churn risk score to each customer. Based on this, it can recommend tailored retention strategies such as discounts, loyalty rewards, or personalized outreach to prevent churn and boost retention.
AI CDP can automate and optimize retention campaigns. It continually improves its churn prediction models by learning from the outcomes of past strategies. Thus, retention efforts become more precise and impactful over time, maximizing overall operations.
By leveraging AI CDPs, you can predict churn and implement real-time, data-driven interventions to enhance customer satisfaction, reduce attrition, and build long-term loyalty.
Dynamic Pricing Optimization
Dynamic pricing optimization using AI-driven CDP transforms how businesses set and adjust prices.
With AI and ML, CDPs analyze vast customer data, including browsing behavior, purchase history, and engagement patterns, to identify demand trends and customer preferences. This allows businesses to implement real-time price adjustments, ensuring they remain competitive while maximizing revenue opportunities.
Besides internal data, AI-powered CDPs consider external factors such as competitor pricing, market conditions, and seasonal trends in the pricing strategy.
These platforms can predict the best price for a product or service by synthesizing multiple data sources, allowing businesses to respond quickly to market dynamics. Such flexibility ensures that pricing strategies align with profitability goals and customer expectations.
The impact of AI-driven dynamic pricing extends beyond profitability—it enhances customer experiences by offering personalized price points or timely discounts based on individual preferences and behaviors.
This fosters trust and loyalty, while real-time adaptability of these platforms positions businesses to stay ahead in competitive markets, making dynamic pricing an essential strategy in modern commerce.
Let us look at the best practices for adopting an AI CDP in your organization.
Best Practices for Using AI CDP in Your Organization
Achieving AI CDP's full potential involves adhering to best practices that promote high-quality data and ethical use in strategic integration. A fundamental best practice is ensuring data accuracy and consistency.
AI CDPs need superior-quality data to create actionable insights. Regular audits of data sources and formats can help avoid errors and ensure that insights derived from the platform are reliable and actionable.
Data privacy and compliance are equally important. AI CDPs handle sensitive customer information; therefore, organizations must comply with privacy regulations, such as GDPR, CCPA, or regional standards.
Clearly communicating with customers about the collection and use of their data helps build trust while implementing strong encryption and access controls that protect against breaches. Ethical AI, including avoiding bias in decision-making, helps maintain an organization's credibility and confidence.
The strategic integration of AI CDPs into other business functions helps maximize its usage. A CDP must be integrated and not used in silos like most other standalone marketing, sales, customer service, or other related business tools to enable a comprehensive and integrated customer experience.
Sharing insights from the CDP across the team ensures aligned messaging and strategy across multiple channels, resulting in seamless and personalized customer experiences.
Continuous learning and optimization are required to increase the value of AI-driven CDPs. Over time, AI models improve with more data, but it is necessary to review them periodically to align them with business goals.
Key performance indicators of conversion rates, customer retention, and campaign ROI are used to measure the effectiveness of business strategies and make improvements based on data-driven insights.
Finally, team training and skill development are essential for successfully implementing AI CDP. Teams must understand the platform's capabilities and limitations to make informed decisions and use it effectively.
Businesses can unlock the full potential of their AI CDP by fostering a culture of data-driven decision-making and encouraging collaboration between technical and non-technical teams, driving growth, innovation, and superior customer experiences.
Future Trends in AI CDP
The future of AI-powered Customer Data Platforms (CDPs) is likely to involve greater automation and smarter decision-making. AI will help to automate the data integration and mapping process, making insights faster and more accurate.
Self-learning algorithms enable CDPs to learn and adapt, thus enhancing performance without extensive manual input.
The next-generation CDPs will evolve to include advanced predictive and prescriptive analytics. AI will no longer just predict customer behavior but also give actionable recommendations that can be applied to improve engagement and conversion.
From suggesting ideal products to optimizing the timing of outreach, CDPs will arm businesses with actionable data-driven decision-making.
With the growing stringency of data privacy regulations, AI CDPs will focus on safe frameworks such as federated learning and edge computing. These innovations will allow for analysis without compromising sensitive information, ensuring compliance while maintaining the power of AI-driven insights.
Unleashing the Full Potential of AI CDP with Tredence
The advantages of AI CDP are multi-pronged. However, incorporating it successfully requires expertise, understanding of client objectives, and being pragmatic of any limitations. That’s where a trusted solutions provider such as Tredence steps in.
Organizations are striving to fully integrate AI and ML to harness their full potential in transforming business operations. Tredence supports these efforts by helping companies achieve their data objectives.
Tredence combines deep data engineering, data science, and ML expertise with extensive domain experience to help organizations modernize their data foundation and transform enterprise data into actionable insights.
Its portfolio includes over 140 AI/ML accelerators designed to address even the most complex data science and ML challenges. These accelerators empower organizations to unlock unprecedented value from their data. Using Tredence accelerators, clients can achieve meaningful results in weeks, not months.
Tredence’s approach delivers tangible results, supported by an industry-leading 94 percent Net Promoter Score across 1,000+ project engagements. Contact Tredence today to transform your organization with advanced data science and ML solutions.
FAQs
Does an AI CDP replace human-driven marketing strategies?
No, AI CDP does not replace the creativity and expertise of human-driven marketing strategies. It acts as a powerful tool to automate repetitive tasks while providing data-driven insights at scale. This enables marketers to focus on more creative and strategic tasks.
Can an AI CDP predict customer churn before it happens?
Yes, AI-powered CDP analyzes customer behavior across multiple touchpoints—such as customer service interactions, website or app engagement, and overall sentiment—providing businesses with insights into potential churn risk. This helps businesses adopt a proactive rather than a reactive approach to mitigate churn.
How does an AI CDP handle duplicate or conflicting customer data?
AI CDP resolves duplicate or conflicting data using fuzzy matching, confidence scoring, source reliability weighting, and recency-based resolution to create a unified, accurate customer profile.
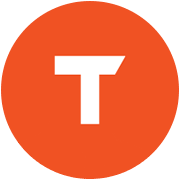
AUTHOR - FOLLOW
Editorial Team
Tredence