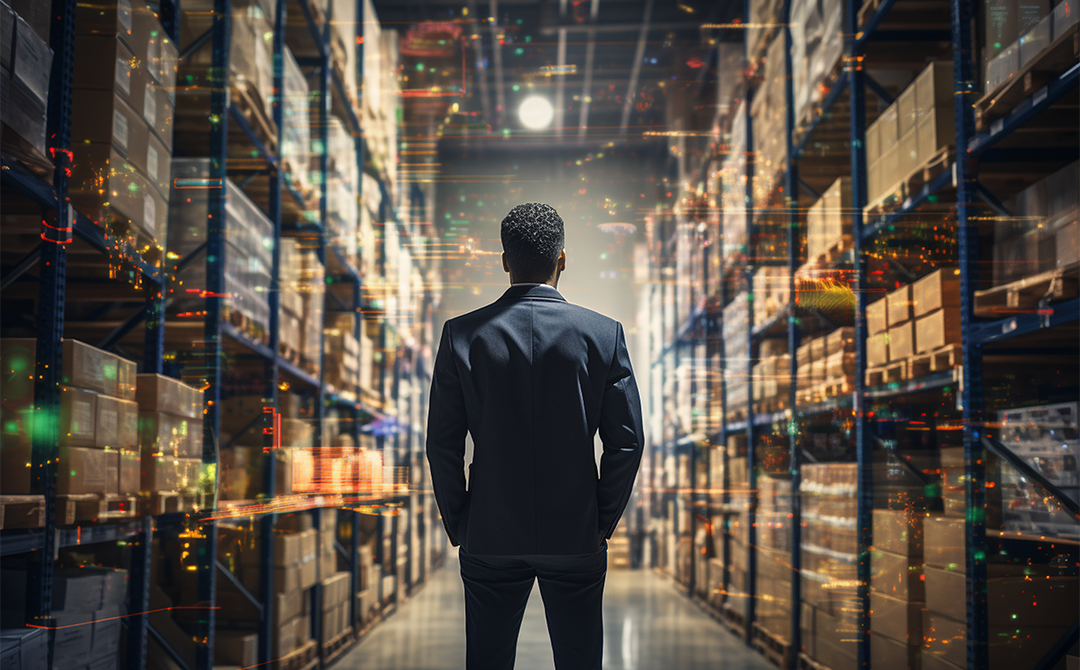
Businesses face mounting challenges in navigating unpredictable consumer demand. Even minor forecasting errors can result in costly consequences—oversupply leads to inventory stagnation, while undersupply means lost sales and dissatisfied customers.
Did you know? Inaccurate demand forecasting and overproduction cause $163 billion in inventory waste annually due to discarded stocks, emphasizing the urgent need for precision. (Source: Bloomberg).
Traditional demand forecasting methods rely on historical data and linear forecasting models and cannot keep up with modern complexities such as market shifts, supply chain disruptions, and fluctuating consumer behavior. Agile demand-sensing strategies, which leverage real-time insights, have become essential for businesses aiming to stay competitive in an increasingly unpredictable landscape.
What is Demand Sensing?
Demand sensing uses AI & real-time data to predict customer demand, reducing forecast errors. Leveraging ML, IoT, and big data, it enhances supply chain agility, cuts inventory costs, and boosts customer satisfaction through optimized stock levels.
This agility enables businesses to respond to fluctuations more effectively, reducing overstocking or stockouts and improving overall operational efficiency.
Research indicates that AI-driven demand sensing can reduce forecast errors by 20% to 50%, potentially saving millions annually in supply chain and inventory costs (Source: McKinsey). For instance, during seasonal peaks such as Black Friday or unforeseen events such as pandemics, demand sensing ensures that businesses can precisely anticipate and address demand surges or drops.
Demand sensing improves supply chain resilience and enhances customer satisfaction by ensuring product availability and reducing lead times. This real-time adaptability makes it an essential tool for organizations aiming to remain competitive in volatile markets.
By integrating demand sensing into their operational strategy, businesses can turn agility into a competitive advantage, seamlessly aligning supply chains with consumer demand (Source: McKinsey).
Importance of Real-time Data
Businesses that integrate real-time data with demand sensing achieve an upper hand over their competitors, it enables then to drive smarter decisions, optimal allocation of resources and control cost, enabling them to adapt to market dynamics
Key advantages include:
- Enhanced responsiveness: Real-time insights from demand sensing help minimize stockouts and excess inventory, ensuring products are available when and where needed.
- Optimized inventory management: Demand sensing enables leaner inventory while reducing the risk of stockouts.
- Competitive advantage: Businesses using demand sensing can quickly adapt to market changes, outpacing competitors who rely on traditional forecasting, optimizing inventory, and better meeting customer demand for a leadership position in a dynamic market.
How Does Demand Sensing Work?
Demand sensing transforms how businesses forecast customer needs by integrating real-time data and advanced analytics. It shifts the focus from static historical trends to dynamic, real-time insights, enabling organizations to respond proactively to market changes.
The process unfolds in three interconnected steps:
1. Data Collection
Demand sensing begins with gathering data from diverse sources, such as sales transactions, inventory levels, weather patterns, social media sentiment, and economic indicators. The purpose is to create a comprehensive dataset that captures both external influences and internal trends.
Data is collected through automated pipelines and IoT devices, ensuring timely and accurate data feeds. This step provides a rich and diverse data pool, laying the foundation for identifying demand signals.
Best practice: Ensure data sources are standardized and validated to minimize errors and inconsistencies in downstream analytics.
2. Pattern Recognition
The next step involves using advanced algorithms, such as neural networks or clustering techniques, to analyze the collected data. The goal is to detect short-term changes and trends in customer behavior, such as sudden spikes in product demand due to weather events or viral social media trends.
This analysis helps organizations understand emerging patterns and prepares them to adapt them in their operations.
Best practice: Employ visualization tools to help teams interpret algorithm-detected patterns and take immediate action when anomalies arise.
3. Predictive Analytics
ML models synthesize identified patterns and historical data in this final stage to predict future demand. These models continuously learn and adapt, providing more accurate actionable forecasts.
This step enables organizations to optimize inventory, align supply chains, and make data-driven decisions.
Best practice: Regularly retrain ML models using the latest data to ensure they remain accurate and relevant in evolving markets.
By integrating these steps, demand sensing empowers organizations to enhance operational efficiency and stay in sync with the fluctuating market demands.
What is Demand Sensing Technology
Demand sensing uses AI/ML to analyze real-time data (sales, weather, trends) for accurate short-term demand forecasts. It helps reduce inventory waste, prevents stockouts, and boosts supply chain agility. It is ideal for retail, CPG, and e-commerce to optimize stock and meet customer demand efficiently.
Technologies Enabling Demand Sensing
Demand sensing leverages a combination of advanced technologies such as AI, ML, big data analytics, cloud platforms, and IoT sensors to enable real-time demand forecasting and optimize supply chain processes.
These technologies collectively analyze data from multiple sources, identify patterns, and make accurate predictions, empowering businesses to respond quickly to market shifts.
ML and AI
AI and ML algorithms analyze vast amounts of historical and real-time data, such as sales figures, consumer behavior, and external factors such as weather and economic conditions. Predictive models and generative AI can foresee demand spikes with great accuracy, offering an advantage over traditional forecasting methods. For example, during high-demand seasons like Black Friday, AI can predict a sudden surge in product demand, ensuring retailers maintain optimal stock levels.
ML also helps continuously improve forecasts by learning from each cycle of data, which ensures that predictions evolve with changing trends.
Big data analytics
Big data analytics plays a crucial role in demand sensing by providing deep insights into complex demand patterns. It aggregates data from various sources, such as social media, customer feedback, and transaction records, and applies prescriptive analytics to recommend actionable strategies.
FMCG companies launching a new product can use big data to analyze purchasing trends, consumer preferences, and demographic insights to predict product demand better and optimize pricing strategies. This enables businesses to make data-driven decisions for inventory management and marketing campaigns.
Cloud data platforms
Cloud platforms support the seamless integration and real-time processing of massive data required for demand sensing. Cloud-based systems allow businesses to scale their demand-sensing operations dynamically, adapting to fluctuations in demand.
Amazon uses cloud technology during events such as Prime Day to adjust inventory levels, update pricing strategies in real-time, and ensure its supply chains remain agile. Cloud data platforms ensure that businesses can process vast amounts of data quickly, offering the flexibility to scale as needed without compromising performance.
IoT sensors
IoT sensors capture real-time data from physical assets in the supply chain, offering unprecedented visibility into demand trends. These sensors track inventory levels, monitor environmental conditions, and provide critical insights during transport.
In cold chain logistics, IoT-enabled temperature sensors monitor perishable goods such as vaccines or fresh produce during transit. If any conditions, such as temperature fluctuations, fall outside the safe range, the system sends alerts, preventing product spoilage and ensuring customer satisfaction. These real-time updates help businesses stay on top of demand trends and ensure product integrity.
Together, these technologies enhance the accuracy and agility of demand sensing, offering businesses the tools to respond proactively to dynamic market conditions and optimize supply chain management.
Benefits of Demand Sensing
Demand sensing is a powerful tool that enhances supply chain operations by enabling businesses to respond promptly to market fluctuations using real-time data and predictive analytics.
- Improved forecast accuracy
Demand sensing allows businesses to generate highly accurate forecasts by analyzing real-time data, which helps make informed purchasing and inventory decisions.
For example, retailers can avoid stockout during peak seasons such as Black Friday, ensuring they meet customer demand efficiently. - Cost reduction
By aligning inventory with real-time demand, businesses can reduce warehousing, logistics, and waste costs. Manufacturers, for instance, can avoid overproduction, thereby optimizing operational costs and minimizing inventory holding costs. - Enhanced customer satisfaction
With accurate demand predictions, businesses can keep high-demand products in stock, improving customer satisfaction. This is especially vital for e-commerce businesses that need to ensure popular products are available during major sales events such as Cyber Monday.
Challenges of Demand Sensing and Strategies to Overcome Them
Businesses need to follow certain strategies to overcome the challenges associated with demand sensing.
Given below are the challenges and strategies that businesses can implement for the successful adoption of demand sensing:
- Data quality and integration
Challenge: Poor data quality and siloed systems can hamper the effectiveness of demand sensing, making it difficult to accurately forecast demand.
Strategy: Implement a robust data governance framework that ensures consistent, accurate, and real-time data integration across all systems. Adopting cloud-based data platforms and data lakes can help integrate and centralize data from disparate sources.
- Technological investment
Challenge: Adoption of demand sensing requires significant investment in advanced analytics platforms, real-time data infrastructure, and machine learning models. For smaller organizations, the cost of acquiring technology and hiring skilled professionals can be a barrier.
Strategy: Start with scalable solutions and incremental investments. Small-to-medium enterprises (SMEs) can leverage cloud-based demand sensing solutions, which offer flexibility and cost efficiency, allowing them to scale as needed. Partnering with external consultants or using managed services can also help mitigate costs.
- Complexity of implementation
Challenge: Integrating demand sensing with existing enterprise systems (ERP, SCM, CRM) and fostering collaboration across departments can be resource-intensive. Additionally, there may be resistance to adopting new technologies and workflows.
Strategy: Adopt a phased implementation approach, starting with pilot projects in less complex areas of the business. Establish a cross-functional team early in the process to ensure that departments work together towards common goals. Focus on change management strategies to help ease the transition and build internal buy-in.
The benefits of demand sensing—such as improved forecasting, reduced costs, and enhanced customer satisfaction—are significant. However, overcoming the challenges related to data integration, technology investment, and organizational readiness is crucial for successful implementation.
By addressing these challenges with tried and tested methods, businesses can integrate demand sensing into their process to optimize the supply chain, better inventory management and gain analytical insights in real-time.
How to Implement Demand Sensing for Your Business: A Step-by-Step Guide
Implementing demand sensing requires technological as well as domain expertise which should be put togather in a structured approach keeping in mind the business use case and goal. Here’s a step-by-step guide to help you incorporate demand sensing and drive supply chain efficiency.
Step 1: Define your objectives and scope
Before exploring demand sensing, it is crucial to define your business objectives. Understand what challenges you want to address, such as improving forecast accuracy, reducing stockouts, or enhancing customer satisfaction.
For instance, a retailer might focus on managing peak season inventory, while a manufacturer might prioritize demand forecasting for raw materials. Clearly outlining these goals helps tailor the demand-sensing process to meet specific needs.
Best practice tip: To track progress, set measurable key performance indicators (KPIs) such as forecast accuracy, reduced lead times, or inventory turnover.
Step 2: Gather and integrate data
Demand sensing requires high-quality, real-time data from various sources, including historical sales data, weather conditions, social media trends, and economic indicators. The more diverse your data sources, the more accurate your demand predictions will be.
Modern businesses use cloud-based platforms for seamless data integration across ERP, CRM, and SCM systems.
Best practice tip: Ensure data consistency by establishing a robust data governance framework to prevent silos.
Step 3: Select the right technology stack
Choosing the right technology is vital for effective demand sensing. AI, ML, big data analytics, and IoT sensors are key enablers. AI and ML models are particularly useful in detecting demand patterns and predicting future fluctuations.
Big data analytics helps analyze complex datasets, while IoT sensors provide real-time updates on inventory levels or transportation conditions.
Best practice tip: Start with pilot projects focusing on specific use cases (e.g., seasonal demand or stockouts) to avoid overwhelming your system and teams.
Step 4: Implement predictive models
Once your data and technology are in place, it is time to build and deploy predictive models. These ML models analyze the collected data to forecast future demand more accurately. The models can also adapt over time, learning from new data to refine predictions.
Best practice tip: Use cross-functional teams from IT, data science, and supply chain departments to monitor and fine-tune models continuously. Collaboration is essential to bridge gaps between technology and operational knowledge.
Step 5: Test and validate
Before full-scale implementation, test your demand sensing model with historical data and validate its predictive accuracy. Simulate real-world scenarios to see how the system performs under various conditions, such as fluctuating market demands or external disruptions.
Best practice tip: Run a controlled pilot phase to measure performance and tweak your models or data integrations as needed before going live.
Step 6: Monitor and optimize
Once demand sensing is implemented, the next step is continuous monitoring. Track performance against your KPIs, such as forecast accuracy and supply chain efficiency. Over time, the system should provide insights that help optimize inventory levels, supplier relationships, and pricing strategies.
Best practice tip: Adopt a continuous improvement mindset. Regularly review and refine your models and data to ensure demand sensing remains agile and effective as market dynamics evolve.
By following these steps, businesses can successfully implement demand sensing, optimize supply chain operations, reduce costs, and enhance customer satisfaction.
The initial setup can be resource-intensive; however, the long-term benefits, such as improved forecast accuracy and more agile decision-making, can significantly outperform the investment.
Case Study: Data-driven Inventory Strategy
Walmart faced the challenge of efficiently managing inventory during the holiday season while keeping costs low and meeting customer demand.
To address this, the company implemented an AI- and ML-powered inventory management system that leverages historical sales data, predictive analytics, and real-time insights to optimize stock placement and distribution.
The system also incorporated external data, such as weather patterns, macroeconomic trends, and regional demographics, to predict demand more accurately.
The AI-driven model ensures products are available when and where customers need them, whether shopping online, through the app, or in stores. It continuously learns and adjusts, factoring in regional demand variations—pool toys are stocked in sunny states, while warm sweaters are prioritized in colder regions.
The AI model is designed to filter out anomalies, such as one-off weather events, ensuring future inventory plans remain unaffected by rare occurrences. Overall, Walmart’s AI-driven strategy improved inventory flow and enhanced customer satisfaction during peak shopping periods. (Source: Walmart).
Difference between Demand Sensing vs Demand Forecasting
Demand forecasting predicts long-term trends using historical data. Demand sensing, on the other hand, uses real-time data and AI to capture short-term demand shifts. While forecasting guides strategic planning, sensing improves agility and accuracy in fast-changing markets.
Conclusion
Demand sensing technology has become essential for optimizing supply chains, as businesses face increasing pressure to be more responsive and customer-centric. Technologies such as AI, ML, and advanced analytics play a key role in capturing real-time demand signals.
According to Gartner, 87% of supply chain experts plan to invest in resilience and AI/ML in the next 2 years. By leveraging these tech stacks, companies can enhance forecast accuracy, optimize inventory, and swiftly adapt to market shifts. (Source: Gartner).
Tredence empowers organizations with advanced analytics, AI, ML, and data engineering to unlock actionable insights and drive calculated decisions. Our supply chain analytics solutions help you build consensus between demand and supply, analyze real-time data to provide actionable insights, reduce shipping costs gives you Its tailored solutions address complex challenges, optimizing operations across industries.
Partner with Tredence to transform your data into a valuable asset and achieve measurable outcomes that provide a competitive edge. Contact Tredence today to discover how it can help you drive growth and innovation.
FAQs
- What is the difference between demand forecasting and demand sensing?
Demand forecasting is a long-term approach that uses historical data to predict future demand over a certain period, typically months or years. Demand sensing is a short-term technique that leverages real-time data and advanced analytics to identify and respond to immediate demand fluctuations.
- How does demand sensing differ from demand planning?
Demand planning is a comprehensive process that involves forecasting, supply chain adjustments, and inventory management to meet the expected demand. Demand sensing is a more focused component of demand planning that specifically addresses the short-term accuracy of demand predictions by using up-to-the-minute data for rapid response to demand changes.
- How can demand sensing improve inventory management?
Demand sensing enhances inventory management by providing real-time insights into demand changes, allowing businesses to optimize stock levels, reduce excess inventory, and avoid stockouts. This results in a more balanced and efficient inventory system.
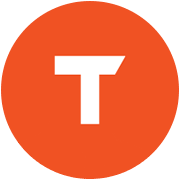
AUTHOR - FOLLOW
Editorial Team
Tredence