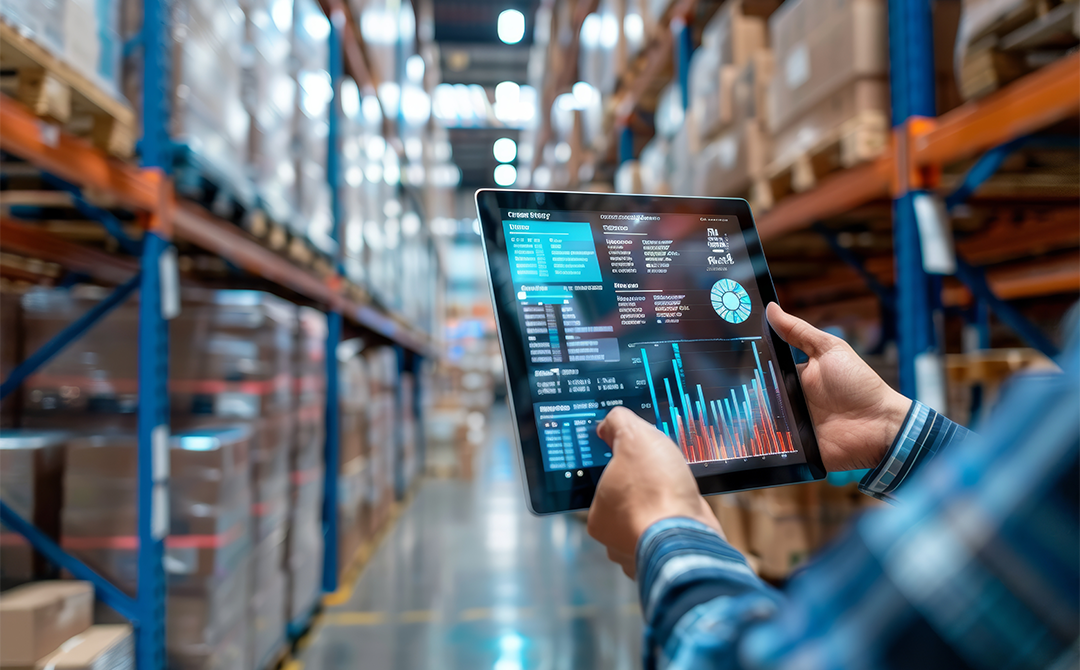
According to a recent survey, nearly 76% of CEOs have invested in AI to enhance their supply chain management. With the same study reporting that 70% of CEOs are seeing RoI from their artificial intelligence (AI) initiatives, it is time for any company that does not use AI across its supply chain to act, or be left behind.
However, AI is an umbrella term that includes many techniques. Predictive AI such as machine learning and statistical models leverage past and real-time data to analyze patterns and forecast likely outcomes to aid decision-making. Most large companies have predictive AI embedded across their supply chain to enable 24/7 agility and enhance long-term strategic thinking.
Meanwhile, generative AI, comprising techniques such as large language models (LLMs), is of a creative bent. It rapidly scans diverse data to offer sharp insights and innovative suggestions to boost the profitability and effectiveness of short and long-term business initiatives. Companies are experimenting with generative AI across their activities including the supply chain.
“In 10 years, supply chains could be highly autonomous, with AI-driven systems managing much of the processes, from procurement to delivery.”
Dr. Ilya Jackson, The MIT Center for Transportation and Logistics
Source: Consumer Goods
If AI has to make your supply chain more agile and competitive than it is today, it is key to understand which type of AI matches best with which activity and optimize or enhance existing AI tools.
Below is an illustrative roadmap of the optimal AI landscape to modernize your supply chain for maximum RoI.
AI for Rapid Response: Handling Short-Term Decision Making
Rapid response has never been more critical. What we once termed volatility and disruption are becoming the norm, creating a huge challenge for supply chain teams to meet parallel growing customer demand.
Already, teams use predictive AI tools that tap into data such as weather forecasts to sense disruptions proactively and help respond optimally and fast. Now, companies can build generative AI capability into their rapid response. It is far more effective at scanning qualitative data such as news reports and social media trends to anticipate sudden, unexpected shifts in supply or demand, facilitating richer data analysis and more accurate responses to emergencies.
Dynamic Supply Network Planning with AI: Medium-Term Decisioning
Supply chains comprise many moving pieces. Changing demand patterns, varying raw material prices, and supplier availability are just some causes that alter the mosaic within days or even hours. Hence, teams have to constantly make never-before decisions and implement changes even during BAU. This can lead to errors due to decision-making fatigue and unintentionally omitting important or new information. Techniques that span predictive and generative AI such as reinforcement learning algorithms can address this concern. By using the diverse causative variables to generate optimal scenarios for dynamic planning throughout the network, blended AI can take the load off supply chain teams, so they can focus on execution and relationship-building.
Here is an example of a successful blended AI innovation for dynamic planning.
One of the biggest logistics companies in the US uses a proprietary AI platform. The platform is used to optimize picking routes within its warehouses, increasing workforce productivity by about 30% while drastically reducing operational costs through optimized space and materials handling. While this is a general use case of AI, the Gen AI touch is its additional layer of customization. The Gen AI-powered application optimizes delivery routes by considering fuel efficiency, delivery priorities, inclement weather, and other route-specific contributing factors. There’s more. The app also enables the company to identify if its trade network is optimized and offers suggestions for improvisations.
Source: EY
Large Language Models for Institutionalizing Expert Best Practices
Modern supply chains are becoming more complex and agile. This makes capturing and institutionalizing expert knowledge even more critical and LLMs can play a valuable role. For example, negotiations are one of the trickiest parts of the procurement process. Teams need to scan past and present supplier contracts, internal and external financial requirements, and contextual risk variables such as political stability in the supplier geographies, to arrive at the most optimal offer.
LLMs can enhance the efficiency and accuracy of this mammoth task by analyzing all the relevant documents, and incorporating recorded and real-time expert input, to speedily make suggestions aligned with the company’s goals. With the best practices comprehensively updated during each run, the negotiations process is continually refined and there is no fear of losing out on recent learning.
Long-Term Scenario Analysis with AI: Strategic Decisions
Long-term strategic decisions in supply chain management, such as network design, capacity expansion and investment in new technologies are well supported by scenario analysis. A blend of predictive and generative AI can serve as an excellent framework to generate these varied scenarios. For instance, a consumer goods giant may be deliberating over transforming its supply chain toward greater sustainability and customer-centricity. This may involve moving away from palm oil suppliers, building more plants and fulfilment centres closer to areas of high demand, and increasing the use of self-drive vehicles and robots within warehouses.
Existing predictive AI can delve into the company’s rich data troves to predict consumer demand via various channels in the next five and even ten years. Using this, the generative AI in the supply chain can help build multiple scenarios incorporating cost analysis and automation-led efficiencies for new supplier networks, manufacturing facilities and fulfilment centres. Thus armed, decision-makers can set the direction for how to shape the supply chain for competitive differentiation over the next few years.
Optimizing AI for a Future-Ready Supply Chain
These are but a few examples of how AI will transform the supply chain. Some lateral enhancements are possible too. Generative AI provides a friendly interface that democratizes innovation. In the completely data-driven modern business landscape, generative AI can generate clean, tailored synthetic data, to supplement ongoing data collection, which can at times prove expensive or inadequate in new, emerging, or sensitive information areas.
As always, optimizations can provide a much-needed fillip to these AI efforts.
- A company can consolidate scattered AI across its supply chain into a single platform that provides E2E visibility and harnesses the expertise of all teams in real time.
An AI-led supply chain control tower that serves as a command centre for the extended supply network can drive the autonomous planning, competitive innovation, and robust resilience that converts the supply chain of the future into a true profit centre.
- Two, it can ensure that the AI algorithms used are constantly upgraded - in terms of the data they ingest from across the ecosystem, be it the IoT systems or data streams from the extended network, and advanced analytics capability.
- New and improved initiatives must be test-run for latency and accuracy before scaling.
- Robust data visualization tools can be continually incorporated so teams waste no time in getting the complete picture and act quickly.
With this approach, AI may soon take over many repeatable supply chain tasks, freeing teams to drive greater innovation and profitability.
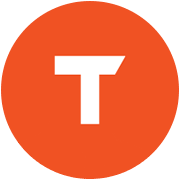
AUTHOR - FOLLOW
Editorial Team
Tredence
Topic Tags
Next Topic
The Impact of Data Analytics on Key Opinion Leader Engagement in Healthcare
Next Topic