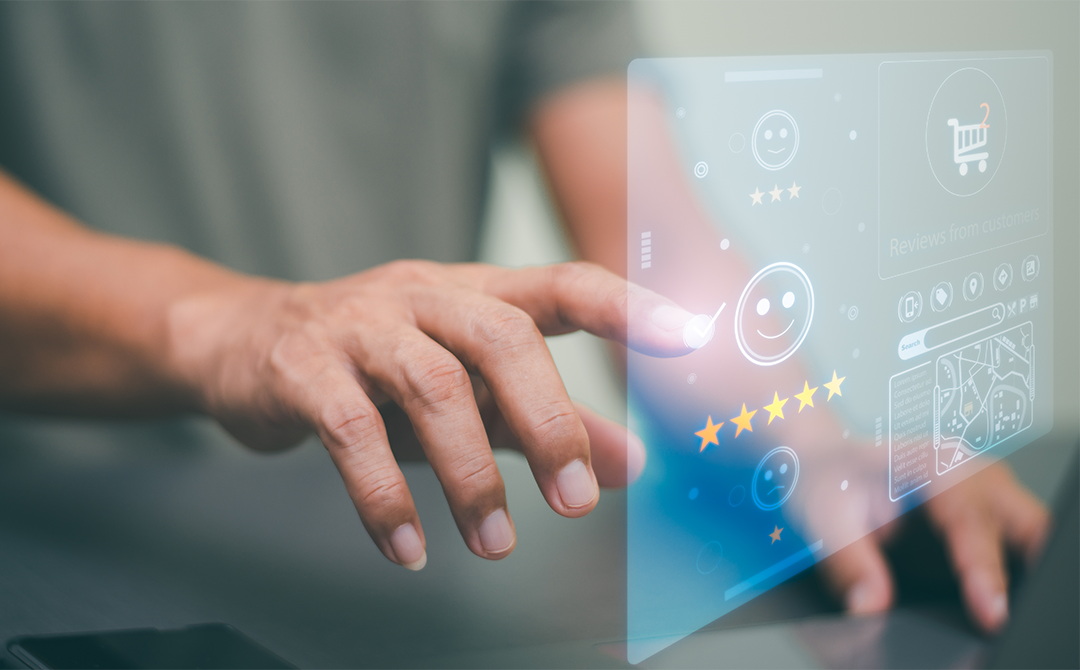
A recent consumer survey found that nearly three in four (71%) expect companies to be transparent in using GenAI. This is not surprising. As companies leverage LLMs and other AI models to provide personalized experiences at scale, consumers ask brands why they should trust a technology that even data scientists and professionals say is a ‘black box’.
The initial and valid push-backs came from organizations and professions who said AI models had used their copyrighted data. However, individual consumers quickly follow suit, asking how these models use their data and the rationale they adopt when making decisions. For example, consumers recently sued insurance firms for allegedly using AI-backed technology for claims denial.
This scenario unequivocally calls for companies to set up comprehensive and robust AI governance and operationalize it post-haste.
By 2026, organizations operationalizing artificial intelligence (AI) transparency, trust, and security will see their AI models achieve a 50% improvement in adoption, business goals, and user acceptance. Source: Gartner Says CISOs Need to Champion AI TRiSM to Improve AI Results |
What is AI Governance?
AI governance refers to a set of guidelines and practices a company follows to ensure that all AI-related activities are fair, ethical, and secure – otherwise known as responsible AI. This includes frameworks used to establish that the data sets used are representative and accurate, norms enforced to comply with privacy and security regulations worldwide, and communication with the end-users, such as consumers, that give the latter the highest possible level of transparency.
Some Approaches
An organization looking to build or improve its AI governance will gain by examining the best practices of leading players. Google has clearly stated its responsible AI principles and so has Microsoft.
To operationalize these principles, consulting firms have suggested a few frameworks. In early 2024, McKinsey proposed a framework for AI governance for companies to use as they scaled. It recommended identifying all the use cases across the company that would see improvement with gen AI deployment–customer journeys, marketing content creation, and summary generation for sales and service teams, to name a few.
The next step would be to enumerate each use case's governance parameters. For example, fairness, data privacy, and explainability are crucial to building and retaining trust along customer journey touchpoints. Once this enumeration has been done, you can define repeatable mitigation approaches that minimize risk every time a task is performed and can also be deployed across use cases, enabling scale. For instance, technical mitigation to list sources of information as part of a query answer can be embedded in customer service and HR chatbots.
Finally, to ensure that alignment with the governance framework is maintained as AI adoption happens at speed and scale, the organization can create a cross-functional group led by a chief AI governance officer, not necessarily hiring from outside.
A Journey to Operationalized AI GovernanceIn 2018, telecom major Telefonica laid out its broad AI principles
Source: UNESCO |
A Look at the Parameters
There are multiple parameters that an AI governance framework has to consider to ensure it meets its stated objectives completely and faithfully.
Here are a few.
Explainable AI
Increasingly, regulations across the world require businesses to be more transparent with customers. This includes the usage of AI. Europe’s General Data Protection Regulation (GDPR) and the California Consumer Privacy Act (CCPA) already needed businesses to be able to tell consumers how any AI that makes decisions using their data works. As governments realize the implications of large-scale AI use, more targeted regulation is rapidly being formulated.
Key AI regulatory developments around the world
Region | Country | Regulation | Status |
Americas | US | Algorithmic Accountability Act 2023 (H.R. 5628) | Proposed (Sept. 21, 2023) |
AI Disclosure Act of 2023 (H.R.3831) | Proposed (June 5, 2023) | ||
Digital Services Oversight and Safety Act of 2022 (H.R.6796) | Proposed (Feb. 18, 2022) | ||
Canada | Artificial Intelligence Data Act (AIDA) | Proposed (June 16, 2022) | |
Europe | EU | EU Artificial Intelligence Act | Proposed (April 21, 2021) |
Asia | China | Interim Administrative Measures for the Management of Generative AI Services | Enacted (July 13, 2023) |
Source: S&P Global
The route to compliance is implementing an explainability layer (XAI). Many of the models are like black boxes which work by identifying patterns almost in an intuitive manner. Explainability tools like SHAP and LIME and many more can be used to dive deeper into what these models are doing. For instance, a SHAP run might tell you that glucose readings are the biggest predictor of diabetes trumping age and BMI. Heat maps of neural network layers and What-IF tools are other ways of understanding what your model is doing. In this way, you are not caught unawares and are always well-equipped to explain to any customer, ecosystem stakeholder or external authority what your AI is doing.
J.P. Morgan set up an Explainable AI Center of Excellence (XAI COE to perform cutting-edge research in explainability and fairness. The XAI COE brings together researchers and practitioners to develop and share techniques, tools, and frameworks to support AI/ML model explainability and fairness. Source: J.P.Morgan |
The human-in-the-loop approach that requires you to run the model’s behaviour by a diverse set of experts is also a tested way to ensure the model is fair and unbiased. A final approach that enhances AI explainability is data governance – something we will now explore.
Data governance
Of course, data underpins everything and its integrity is paramount. Businesses have considerable experience ensuring this but are now faced with the new challenge of scaling their data management practices in the face of a complex explosion. AI deployment is racing ahead, pulling in varied data from across the ecosystem, and being used not just by diverse teams but even end-consumers who share their information with it on the go.
Against this backdrop and to completely address the critical regulatory compliance we earlier mentioned, a good data governance approach that covers the following dimensions is key:
- Where is the data coming from: Provenance
- Whether it is accurate: Accuracy
- Who can access how much of it: Access Control and Privacy
- Whether it is secure and how to keep it secure: Security.
A sure-shot way of tackling the challenge is the setting up of a unity catalog that serves as a single source of truth. It is a central data repository with best practices and norms in place even before data enters the business engines to ensure accuracy, access, and security. With no room for doubt about which source to turn to when data is questioned anywhere in the AI lifecycle, and the flexibility to enforce these dimensions at scale, a unity catalog equips companies to confidently deploy new technologies like LLMs.
Walmart’s Digital Trust Commitments provide a foundation for the company to earn and maintain customer trust in an omni-channel, data- and technology-driven world. Cybersecurity programs work 24/7/365 to protect data and infrastructure. The company has pledged to use artificial intelligence (AI) transparently and responsibly, and always in line with Walmart’s values. Source: Walmart |
Reproducibility
While much is said about how models like LLMS learn on the go and boost innovation, like in all other activities, businesses need to have well-defined workflows to develop, deploy and monitor these and other AI models – possibly even more so. This will minimize unpredictability throughout the lifecycle such as not having enough data, facing failure mid-deployment and struggling to scale.
This streamlining is known as reproducibility – always performing tasks in adherence to best practices and has under its umbrella explainability, data governance, documentation, mitigation and much more. From an operational perspective, the comprehensive set of practices is known as MLOps and spans data engineering, data science and IT systems.
This list of parameters only scratches the surface. The business world is clear that the deployment of AI will be for the greater good and not purely for profit. Areas like bias mitigation and ethics are central to this thought process and will see significant focus in the next couple of years. Data scientists will work closely with social scientists and ethicists so businesses build models that help people, and never tread on their privacy or beliefs.
In conclusion
Setting up strong governance as your AI scales is non-negotiable. It’s early days so you gain by starting today. With the involvement of every stakeholder right from the company board to functional teams, and end-consumers, openness to feedback and robust accountability mechanisms, and an expert partner you can ensure your deployments enhance your brand’s reputation at every touch point and rarely dent it. The instituted frameworks will also boost AI performance and save costs, delivering an additional win.
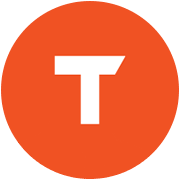
AUTHOR - FOLLOW
Editorial Team
Tredence
Topic Tags
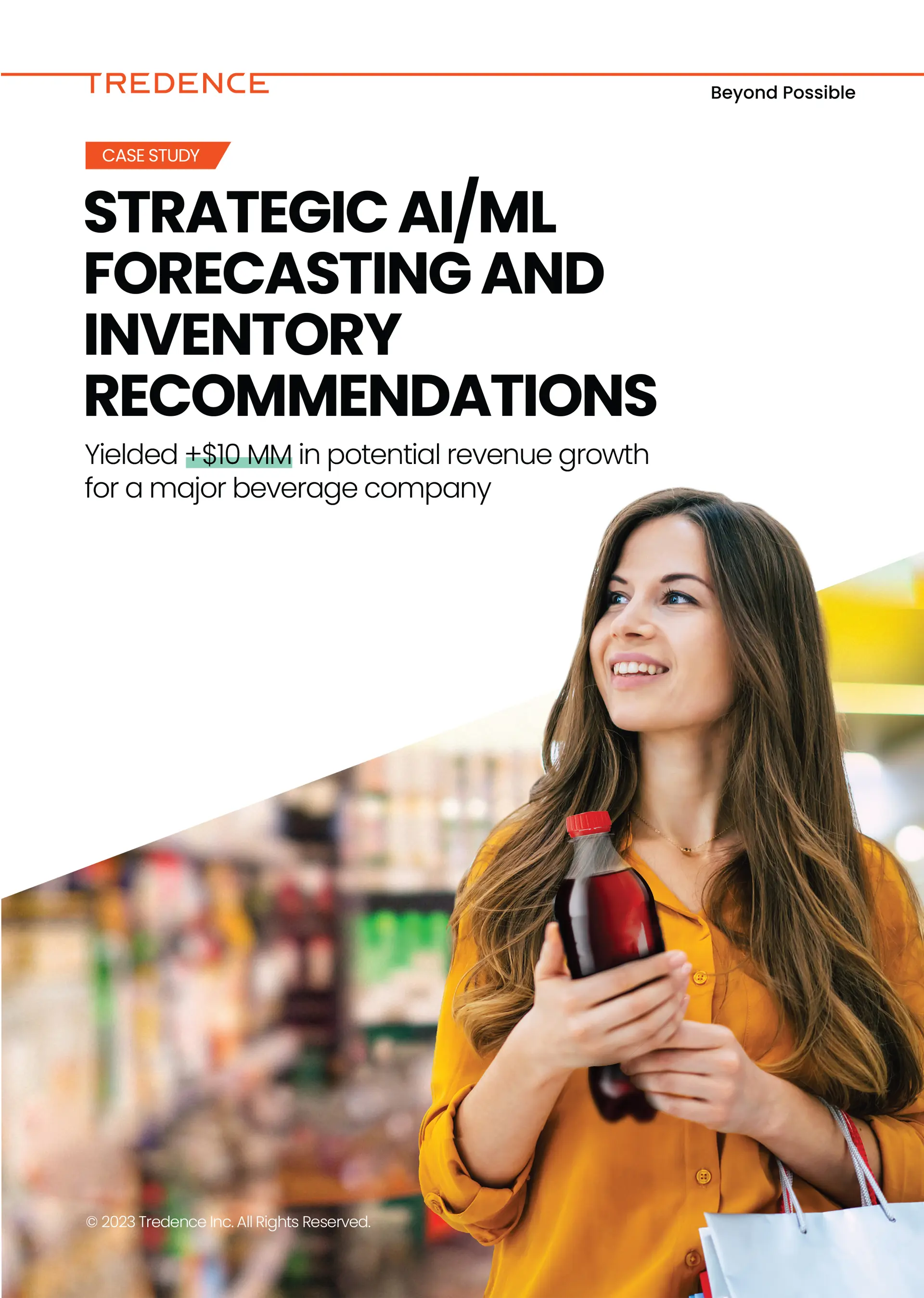
Detailed Case Study
AI/ML forecasting yielded revenue growth of $10MM for a beverage giant
Learn how a Tredence client integrated all its data into a single data lake with our 4-phase migration approach, saving $50K/month! Reach out to us to know more.
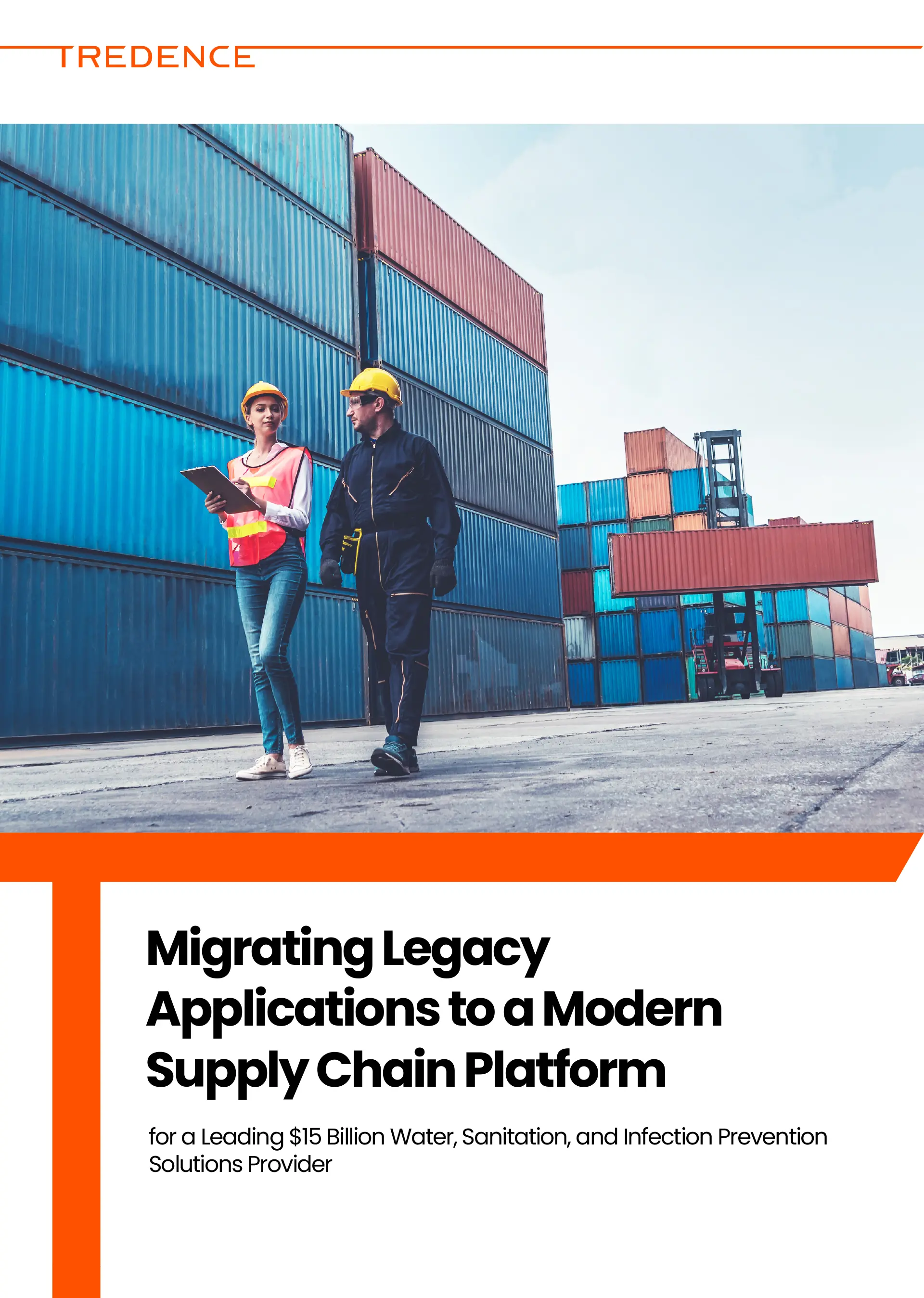
Detailed Case Study
MIGRATING LEGACY APPLICATIONS TO A MODERN SUPPLY CHAIN PLATFORM FOR A LEADING $15 BILLION WATER, SANITATION, AND INFECTION PREVENTION SOLUTIONS PROVIDER
Learn how a Tredence client integrated all its data into a single data lake with our 4-phase migration approach, saving $50K/month! Reach out to us to know more.