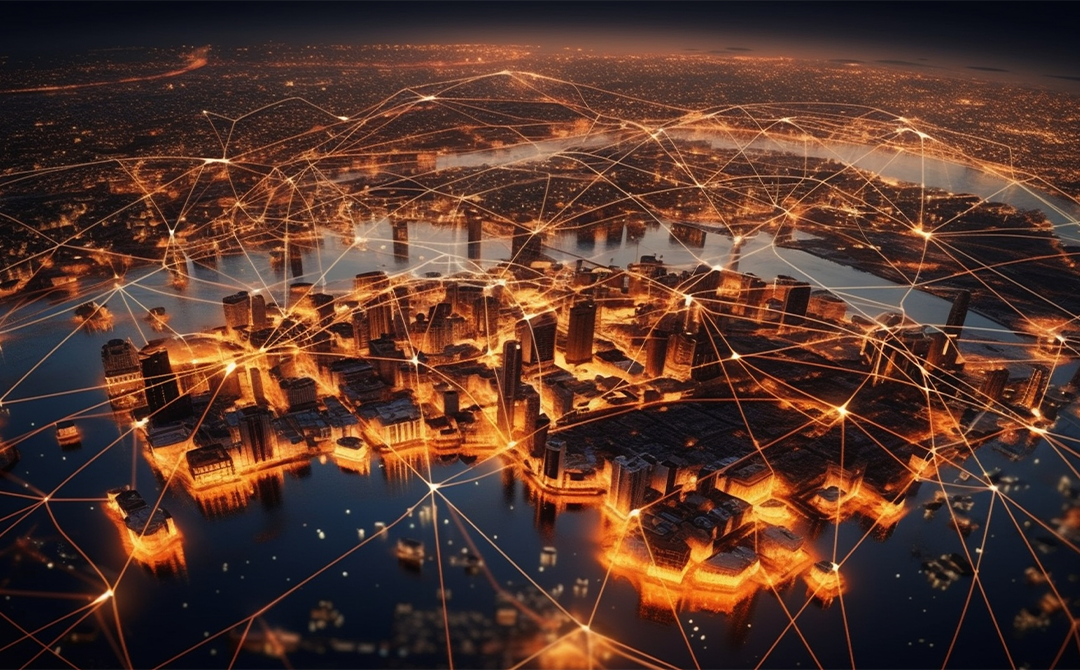
After the launch of ChatGPT in 2022, which was a significant moment in promoting English as the primary programming language and introducing advanced AI technologies like Generative Pretrained Transformers (GPT), a new era of innovation emerged. By mid-2023, about one-third of respondents in a McKinsey survey reported using generative AI regularly in at least one business function. However, these efforts usually started with ambitious objectives and significant momentum, only to experience a decrease of about 42% in their expected value later in the transformation program as the focus shifted towards reaping tangible benefits.
Is Generative AI (GenAI) a Valid Investment for Supply Chains, or Just One More Expensive Add-On?
The guiding principle in the Supply Chain sector is ‘We swiftly learn from mistakes and adjust quickly.’ This motivates companies to accelerate their adoption of GenAI in the supply chain to promptly turn concepts into valuable results, emphasizing the improvement of productivity, efficiency, and technology with a focus on human involvement. While elements of GenAI might appear mystical, they frequently depend on human input to achieve their maximum effectiveness. Let's delve deeper and explore how generative AI could revolutionize supply chain planning – a function dealing with numerous uncertainties, global tensions, and diverse strategies.
Demand Planning Today: GenAI and Outside-In Approach for a Sustainable Supply Chain
Demand planning, a crucial aspect of successful supply chain operations, has always relied heavily on historical data analysis and statistical models. However, these traditional methods often fail to capture dynamic shifts in market trends and unforeseen events, prompting the pursuit of more adaptable and forward-thinking solutions. Despite the advancements in AI and machine learning (ML) models, such as tree or ensemble-based approaches, there remain limitations in capturing external factors that impact forecast accuracy.
While current AI/ML methodologies integrate various data sources, both internal and external, to businesses, GenAI is viewed as an additional layer of analytics that could augment existing approaches, pushing predictive capabilities to new heights. This technological leap means planners can now spend less time fine-tuning forecasts manually and instead focus on strategic decision-making and rapid responses to market dynamics.
Traditional supply chain planning often adopts an inside-out approach, where forecasts and decisions rely predominantly on internal data and historical trends. However, this method may overlook variations in demand across different customer segments or product categories, leading to inefficiencies like overstocking or stockouts. Conversely, recent outside-in planning methodologies prioritize understanding customer demand signals and market dynamics to inform supply chain decisions. By analyzing diverse external data sources, such as customer feedback and social media sentiment, outside-in planning empowers organizations to adapt swiftly to changing market conditions, enhancing overall agility. Within this promise lies a critical assessment of GenAI's practicality.
Can GenAI deliver on its potential to transform demand planning processes, or does it introduce complexities and costs that outweigh its benefits?
To delve deeper into this inquiry, we will explore a few critical demand planning GenAI use cases:
Generative AI Use Case 1: Strategic Decision Support as a Conversation Chatbot (Knowledge Management)
Gartner predicts that 25% of KPI reporting will be supported by GenAI by 2028, establishing how GenAI can serve as a value-adding feature, enhancing existing supply chain functions through productivity gains and user-friendliness. It accelerates operations and decision-making, offering a chatbot-like interface that accesses a wide array of real-time datasets, both internal and external. GenAI facilitates faster, more accurate decision-making processes by streamlining complex queries and data analysis, eliminating the need for laborious SQL or code-based queries and extensive data science resources.
In a typical demand planner's routine, a GenAI chatbot can provide insights into the impact of events across various supply chain nodes, allowing planners to make informed decisions swiftly. For instance, a demand planner overseeing a regional distribution center can query the chatbot to assess the ramifications of product-related shortfalls or transport delays in preceding nodes.
Models like Picard Big (T5-3B) and GPT 3.5 can be leveraged to create an iterative prompting strategy to recommend alternative stocking and service level strategies to mitigate risks effectively. Additionally, LLM-powered multi-agents aid in Correlation Analysis, Anomaly Detection, and Root Cause Analysis (RCA), providing comprehensive support for end-to-end visibility and strategic decision-making for companies striving to manage a balanced scorecard.
Generative AI Use Case 2: Streamlining Demand Planning with Generative AI's Data Labeling Automation
Demand planning has long been a labor-intensive practice in traditional supply chain management, encompassing two crucial steps: forecast review and collaboration. These processes typically involve manual scrutiny of forecasting results and extensive coordination with various teams to ensure forecast accuracy. Recognizing the need for automation in these areas, supply chain organizations are turning to GenAI to streamline demand planning operations.
By leveraging GenAI's advanced deep learning techniques, supply chain planners can now automate the laborious task of data labeling. Traditionally, supply chain organizations relied on discriminative AI models like logistic regressions and decision trees for predictive analytics. However, these models often faced limitations in scalability and explainability, impeding their effectiveness. GenAI addresses these shortcomings by utilizing deep learning algorithms and neural network architectures to analyze vast datasets and automatically assign relevant labels to different data points. This automated labeling process saves time and effort and allows planners to focus on more strategic tasks rather than manual data labeling.
Techniques like transfer learning enable GenAI to adapt pre-trained models to specific supply chain contexts, minimizing the need for manual annotation efforts and accelerating the deployment of AI solutions. Additionally, tools like GPT 3.5 turbo can be utilized to create search engines that enable the use of planning data models, further enhancing the capabilities of GenAI in demand planning.
Generative AI Use Case 3: GenAI for Scenario Planning and Future-Projected Data Synthesis
ChatGPT has an intriguing feature known as "what-if-what-now GPT," which allows users to simulate various future scenarios, determine probabilities, and plan for prescriptive actions. Users can input the contexts and boundaries for these scenarios, or GenAI can derive them from a large volume of real-time media and industry-related data. This process generates multiple potential instances to aid demand planners or supply chain decision-makers in better planning for future scenarios. GenAI then suggests prescriptive actions to mitigate these risks preemptively, based on user-specified planning timelines.
Drawing insights from a recent HBR article, this type of scenario planning was explored in-depth, particularly from a category manager's or demand planner's perspective in a hypothetical retail setting. While ChatGPT may lack finer details like revenue or current availability within the category, it can be trained on these aspects by providing relevant data and prompts. Users can interact with ChatGPT like a personal assistant, conveying inputs, scenarios, and constraints and then request it to devise risk mitigation strategies for the next 1-3 months based on potential risk-based events.
GenAI's utility lies in modeling these future-risk-based scenarios, determining the probability of underlying events from diverse real-time external datasets, including shipping, media, market research, economic, and regulatory sources. This process, which would take days or weeks for a human to summarize entirely, is executed swiftly by GenAI.
As a demand planner, one can establish potential boundaries for normal, best-, and worst-case scenarios and task the chatbot to synthesize data and simulate possible outcomes. The objective is to devise recommendations to maximize profitability and customer service levels. Generative AI techniques, such as generative adversarial networks (GANs) and variational autoencoders (VAEs), play a crucial role in synthetic data generation. Synthetic data addresses data scarcity issues and allows for the creation controlled and diverse datasets for testing and experimentation without exposing sensitive information.
Planners and domain experts can then evaluate recommendations based on merit and value as needed by the business. Alternatively, GenAI can prioritize scenarios and recommendations based on likelihood of occurrence, value to the business, potential risks, and other objectives as required. Using Reinforcement Learning from Human Feedback, the models can constantly learn and assess.
Generative AI Use Case 4: Retail Media Networks and Customer Propensity Across Channels
The notion that supply chain excellence hinges solely on balancing cost, customer service, and inventory oversimplifies the dynamic landscape. As retail media networks and omnichannel strategies increasingly shape customer purchasing behavior, GenAI is increasingly needed to capture, contextualize, personalize, and optimize promotions and content across channels.
GenAI's role is pivotal in analyzing correlations between digital content types, product attributes, promotions, and their impact on customer purchasing behavior across various channels. By leveraging vast volumes of historical customer-level data, GenAI can discern patterns and preferences, enhancing predictive capabilities to nudge customers toward purchasing specific products through targeted content.
Over time, these models refine their predictions, enabling the generation of optimized content, such as promotions and images tailored to maximize conversion rates. By recognizing and managing distinct flows based on user personas – efficient, responsive, and agile supply chains –, GenAI facilitates the design of supply chains to efficiently cater to diverse customer demands. From a supply chain perspective, Understanding customer demand drivers across channels is imperative for CPG and retail companies. Whether demand sensing is performed at a segment or customer level varies depending on the use case. While segment-wise approaches are suited for demand planning, customer-level insights are invaluable for personalized marketing initiatives.
However, fulfilling customer demand and ensuring visibility into availability remain core supply chain challenges. Incorporating data from GenAI and retail media into demand planning at a segment level remains essential for capturing maximum market share for specific product-region combinations.
How Generative AI is Redefining Supply Chains for Sustained Competitiveness
Beyond the Financials: Implementation Challenges and Considerations
Despite the nascent stage of GenAI adoption in demand planning and lingering skepticism, its potential value in bi-directional orchestrations is evident. The value of GenAI extends beyond mere cost savings. It encompasses strategic advantages such as increased agility, competitive differentiation, and proactively meeting customer needs.
Data Quality and Availability
The effectiveness of GenAI is contingent on the quality and comprehensiveness of the data fed into it. Ensuring data integrity and accessibility is a prerequisite for success.
Talent and Expertise
The scarcity of talent with the requisite skills in GenAI and demand planning poses a challenge. Investing in training and development is crucial. Given recent instances of hallucination in recommendations and poor accuracy, human intervention becomes key to re-train and improve existing models on the go. Most key decisions will still rely on human judgment as GenAI continues to be a productivity improvement support system.
Ethical and Privacy Concerns
The use of GenAI raises questions about data privacy, the ethical use of AI, and potential biases in AI-generated forecasts. Navigating these concerns with transparency and integrity is essential. While GenAI holds immense promise, it's crucial to acknowledge its limitations and ensure its implementation aligns with business objectives.
Data Dependency
The effectiveness of GenAI heavily relies on the quality and quantity of available data. Businesses lacking robust data infrastructure might struggle to leverage GenAI effectively.
Explainability and Transparency
Understanding the rationale behind GenAI-generated insights can be challenging. Businesses must prioritize solutions that provide clear explanations for forecasts, fostering trust and enabling informed decision-making.
Integration Challenges
Integrating GenAI seamlessly into existing planning processes is crucial. Businesses must invest in building the necessary technical expertise and infrastructure to ensure smooth integration.
GenAI - A Tool, not a Silver Bullet
In conclusion, Gen-AI should be looked at as an intelligent enabler. The induced strategies are to be best used as raw ideas and jump-off points for further thinking, detailing, and refinement rather than a final plan that should be adopted without reservation or further analysis.
While GenAI holds promise in planning processes, it is not a cure-all. It is essential to assess the existing digital capabilities, including unified planning models, master data management, and S&OP playbook, to ascertain operational feasibility and potential return on investment (ROI). By acknowledging its limitations, pinpointing right generative AI use cases in supply chain, and fostering the necessary competencies, organizations can harness GenAI as a potent instrument to elevate demand planning precision and adaptability. This transformation can shift its role from a potential burden to a substantial value enhancer.
Leverage Generative AI Services/Solutions for a Strategic Supply Chain Advantage with Tredence
Supply chain leaders and their IT partners must prioritize solutions that are not merely off-the-shelf but thoughtfully built or customized to harness GenAI’s potential. This requires a nuanced understanding of GenAI, deep domain knowledge in the supply chain, and change management expertise to guide these intelligent systems' adoption and ongoing evolution.
Tredence has a proven history of deploying enterprise-wide and customer data platforms that enhance visibility, capabilities, and predictive intelligence across all business facets. Our tailored services and accelerators help you swiftly achieve your goals with data, personalization, and analytics, thus ensuring a quicker and more substantial return on investment. Our team's extensive experience in deploying and fine-tuning large language models (LLMs) addresses complex challenges effectively. With successful implementations using Open AI for many AI generative AI solutions, we're ready to bring this expertise to your enterprise. With Tredence, you can amplify your AI and automation efforts with GenAI as a layer of augmentation and a collaborator for innovation.
We’re offering a complimentary 60-minute discovery call to help you explore new business opportunities you can unlock with our generative AI services.
Connect with our GenAI experts.
Topic Tags