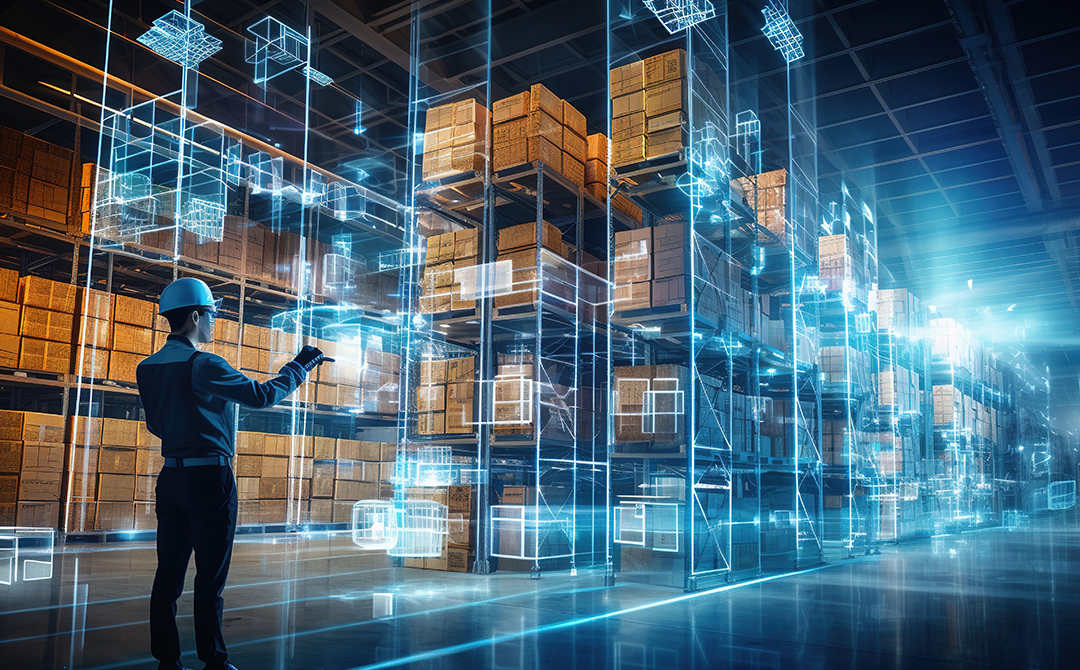
Modern supply chains sit at a unique confluence of factors. Their mastery of optimization and reliability at scale is being tested by the rapidly rising expectations of the digital-first customer. And if this wasn’t enough, the vagaries of the weather and geopolitical instability, which were thought to be challenges of the past, have re-emerged with considerable force.
It is almost as if, in response to these daunting difficulties, generative AI in supply chain has arrived as new technology.
What is Gen AI, and how does it differ from traditional AI in supply chain management?
Supply chains have long used traditional AI as a part of their automation to improve employee productivity and the efficiency and accuracy of critical processes such as demand forecasting and inventory optimization. Tools like decision trees and statistical models perform pre-set tasks that comprise these processes very reliably based on learnings from historic data sets.
However, navigating current supply chain volatility requires more than automation and fixed learnings. Next-generation generative AI (Gen AI) with its ability to support innovative, live strategizing based upon near-real-time insights, can provide the responsiveness supply chain teams today need and is rapidly emerging as the cutting-edge problem-solving tool of the hour. Generative AI solutions are becoming integral in addressing these challenges.
Generative AI stands tall over traditional AI
Generative AI supply chain models like natural language processing (NLP) and graph analysis learn on the fly from live data streams and create new content in formats that are easy for teams to understand and apply. Generative AI has one more key advantage over traditional AI. From numbers to reams of text and images, it is not limited by data types and sources and can effortlessly scan massive volumes of diverse structured and unstructured data fast for inputs. These powerful abilities that distinguish gen AI from other analytics engines can help supply chain teams shatter data and communication barriers throughout their ecosystem.
Half of leaders planning to implement generative AI in supply chain within a year.Chief supply chain officers (CSCOs) are dedicating 5.8% of their function’s budget, on average, to generative AI.
Source: Gartner
Real-world applications of generative AI in supply chain management: Improving stakeholder relationships
The effectiveness of a supply chain depends on the quality of its relationships with diverse stakeholders across numerous ecosystems. Financial service organizations, regulatory bodies, multinational retailers, and small and medium-scale suppliers are all equally important partners. Supply chain leaders and their teams have to work with FSIs for trade finance, cooperate with regulatory bodies for reporting and compliance, and identify suppliers, retailers, and logistics partners who comply with local, cross-border, and internal regulations that span business and ESG dimensions, and build strong relationships with them.
While large organizations have automation and traditional supply chain AI in place to help teams with these activities, generative AI applications in supply chain can significantly enhance these efforts.
Generative AI in supply chain involves tools such as LLMs and Reinforcement Learning (RL) agents to scan the latest updates on a range of influencing variables, such as business and ESG regulations, legal frameworks, and the reputational history of a partner, and create new approaches on the go. This capability can empower both supply chain teams and their ecosystem partners during key negotiations and conversations and also help in the accurate drawing up of contracts and reports. Graph Neural Networks (GNNs) can help supply chain analytics teams proactively identify potential areas of non-compliance so they can rapidly alter internal mechanisms or alert external partners and avoid serious regulatory and reporting concerns.
In this way, generative AI in supply chain supports every single process that drives relationships between the supply chain team and external stakeholders with accurate, well-researched, and contextual information and recommendations, leading to frictionless, long-standing trust-based equations. Generative AI services ensure that these interactions are smooth and productive.
In a recent successful application, a US retailer built a generative AI-led bot to conduct negotiations with its vendors, and over 65% of vendors found that they preferred dealing with the bot over humans. The technology’s ability to rapidly grasp complex discussion points and marry them with facts and processes to propose several alternative scenarios is likely to have led to this preference.
Boosting resilience
Supply chains in large companies are vast, complicated international networks spanning thousands of suppliers, distributors, retailers, and numerous owned and contractor manufacturing plants. Since the multidimensionality of the challenges to the reliability of this interconnected network is growing in tandem with its complexity, the abilities of existing decision-making engines, such as traditional AI, are proving inadequate for proactive risk mitigation.
However, generative AI supply chain models, which can ingest the varied causative data and provide multiple, granular strategies on the go, can help effectively address current, real-time supply chain visibility challenges.
For instance, NLP techniques can use social media sentiment analysis to predict customer demand or weather forecasting alerts to spot likely logistical disruptions. Simulation models can mimic the impact of real-world disruptions (e.g., delayed shipment, sudden demand surge) and provide live recommendations for mitigation. Outlier detection can identify anomalies and potential disruptions in real-time data streams, like unexpected traffic congestion or social media buzz around product safety concerns. Additionally, generative AI in supply chain management can synergize with the digital twins to test and optimize responses to potential disruptions.
Currently, a global CPG leader uses NLP models to draw data from social media, news publications, and information bureaus and determine the real-time impact of extreme weather, port congestion, traffic, and socio-political happenings on its supply chain. This has delivered 78% stock-out prediction accuracy and saved the company nearly $90 million in losses.
Enhancing Employee Satisfaction and Collaborative Innovation
Employee satisfaction leads to customer satisfaction. And with seamless digital experiences in their personal lives, employee expectations are now rising. It is no different with generative AI solutions. Empowering supply chain teams with the technology will boost morale, unlocking far more than efficiency and cost savings.
Since generative AI’s advanced capabilities can support the existing automation of daily activities like invoicing, drawing up contracts, credit notes, estimates, and order placement with real-time updates to function more accurately and speedily, this will free up employees for strategic work and innovation. Since genAI learns dynamically, it will also spot new and dangerous fraudulent activity faster than current technologies and practices, saving time and money and significantly reducing worry for supply chain teams. Generative AI services from leading providers help build cross-disciplinary capabilities to enhance user experience (UX) design, model development, governance, change management, and ongoing managed services.
Crucially, when employees utilize the time freed up by generative AI for upskilling or innovation, the technology can be a very helpful co-pilot, thanks to its growing accessibility. Supply chain teams can leverage the user-friendly interfaces of gen AI tools, creative content generation and data analysis to:
- Upskill themselves continuously, learning new skills and adapting to evolving trends in supply chain management and data analysis.
- Create collaborative lab and CoE scenarios to rapidly and continuously build value-added innovation that will improve processes and profitability.
Top multinationals have recognized that generative AI applications will motivate supply chain teams to transform the supply network into an innovation engine with a competitive edge and are setting up labs to explore the powerful combination of generative AI and human ingenuity.
Generative AI Playbook: Navigate Growth Horizons with Tredence
Current adoption trends of genarative AI in supply chains and who's leading the way?
Currently, Gartner has found that the most impactful generative AI use cases in supply chain include code augmentation, providing more insights into supply chain KPIs, and staff assistance chatbots. In addition to materials and goods-intensive sectors like retail, e-commerce, manufacturing, and logistics, knowledge-focused sectors like healthcare are also leveraging the technology for their critical supply chains.
Since the supply chain lags other business functions in gen AI adoption, it can convert this into an opportunity by capitalizing on the early learnings and technology investments of other teams like marketing and sales, Gartner advises.
Potential obstacles in implementing generative AI in supply chain?
A few critical points need to be kept in mind during generative AI adoption for supply chains. Since these generative AI models run on data within and outside the organization, and reformulate learnings on the go, a thorough understanding of the models used, any potential bias and ethics concerns, and the local, global, and organizational security and privacy norms is non-negotiable. Since models are only as good as what goes into them, data quality cannot be ignored.
Preferably, these models should not be 100% off-the-shelf but built or customized in partnership with consultants with a nuanced understanding of Gen AI, the supply chain domain, and expertise in change management during the adoption phase, and monitoring the models as they evolve. Finally, supply chain teams and their IT partners must avoid reinventing the wheel in their enthusiasm to leverage Gen AI for efficiency and innovation. Gen AI will be much faster in creating impact and positive RoI when it starts off as an additional layer to augment the existing rules-based AI and automation and as a collaborator for innovation.
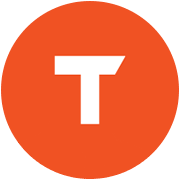
AUTHOR - FOLLOW
Editorial Team
Tredence
Topic Tags
Next Topic
How Databricks and MLflow Could Accelerate LLM and GenAI Competency in Data Science Frameworks and Internal Tools
Next Topic