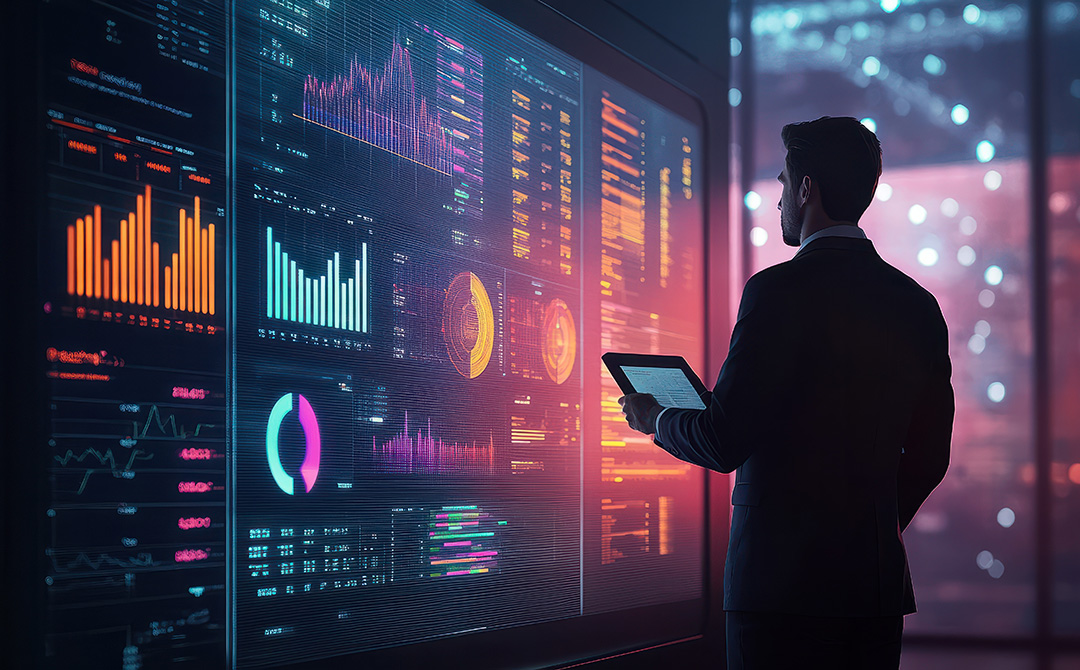
In 2023, a central European bank found a complex fraud scheme that had previously evaded typical detection systems for months. By examining vast volumes of transaction data and creating predictive fraud scenarios, generative AI abnormalities that human analysts had overlooked. Millions of dollars in potential losses were stopped within days. Source
This is only one instance where Generative AI is changing finance. Financial institutions use such technologies AI for individualized investment strategies, automated risk modeling, and AI-driven compliance audits to improve decision-making, security, and efficiency.
Yet, what exactly is generative AI in a financial context,? How does it differ from conventional artificial intelligence models? Which main prospects and difficulties should financial firms give thought to? Let's find out.
What is Generative AI in Finance? Why Does it Matter for the Future of Financial Services?
Generative AI in financial services relies on highly advanced machine learning models–particularly large language models (LLMs) and deep learning networks trained on massive datasets–to generate new insights, automate complex processes, and enhance decision-making. Unlike traditional AI systems, which often follow predefined rules or focus on narrow predictive tasks,,generative AI can create novelfinancial models, simulate realistic market conditions, and even automate customer interactions with human-like responses.It enables banks, insurance firms, and investment companies to automate tasks that once required extensive human effort, such as portfolio management, fraud detection, and financial forecasting.
Traditional AI vs. Generative AI in Finance: Key Differences
Feature |
Traditional AI in Finance |
Generative AI in Finance |
Functionality |
Follows rule-based algorithms |
Generates new data, insights, and scenarios |
Application |
Predictive modeling, automation |
Market simulation, personalized financial advice |
Decision-making |
Data-driven but limited by pre-defined inputs |
Can synthesize new insights based on multiple data points |
Examples |
Credit scoring models, automated trading |
AI-generated financial reports, synthetic market data |
Key Components of Generative AI in Financial Services
Generative AI is reshaping financial services by introducing advanced tools that drive innovation and efficiency. As financial institutions strive for smarter decision-making and enhanced customer experiences, a few core components stand out:
- Natural Language Processing (NLP): NLP empowers systems to understand and generate human-like financial reports, summaries, and customer interactions. This capability streamlines communication and enhances data interpretation across diverse financial documents.
- Deep Learning Models: By identifying intricate patterns within large datasets, deep learning models optimize risk assessment and bolster fraud detection. Their continuous learning abilities allow institutions to refine strategies and respond proactively to emerging threats.
- Synthetic Data Generation: This approach creates simulated financial scenarios that enable rigorous testing of risk models. By replicating a variety of market conditions, synthetic data supports improved investment strategies and robust risk management.
- Conversational AI: Driving AI-powered chatbots and virtual financial advisors, conversational AI enhances customer service and portfolio management. Its real-time interaction capabilities ensure personalized support and efficient resolution of client queries.
Importance of Generative AI in Financial Services
The financial services industry, driven by billions of daily transactions and stringent regulatory requirements, is rapidly evolving. Generative AI is emerging as a transformative force, empowering institutions to automate complex processes, derive actionable insights, and elevate customer experiences.
1. Enhancing Risk Assessment and Fraud Detection
Generative AI analyzes historical transaction data to detect anomalies and predict fraudulent activities before they occur. AI-powered systems can flag suspicious patterns in real time, significantly outperforming traditional rule-based models. Additionally, generating synthetic fraud scenarios allows continuous refinement of detection algorithms.
Example: JPMorgan Chase leverages AI models to analyze payment transactions and accurately detect fraudulent activities, reducing false positives and improving security. (Source: JPMorgan)
2. Personalized Financial Services and Customer Engagement
With AI-driven insights, financial institutions can offer tailored financial advice, investment recommendations, and streamlined loan approvals. AI-powered chatbots and virtual assistants provide instant, personalized guidance, while dynamic portfolio management strategies cater to individual risk profiles.
Example: Morgan Stanley’s AI-powered assistant analyzes vast amounts of financial data to provide personalized investment insights to clients in real-time. (Source: Morgan Stanley’s)
3. Automating Compliance and Regulatory Processes
Facing strict regulatory mandates, financial institutions utilize Generative AI to automate compliance functions. The technology extracts key insights from legal documents, generates audit reports with minimal human intervention, and streamlines KYC (Know Your Customer) and AML (Anti-Money Laundering) processes.
4. Optimizing Trading Strategies and Market Predictions
Generative AI processes massive datasets to simulate market conditions and build predictive models, empowering real-time algorithmic trading. This capability enables hedge funds and institutional investors to better assess risks and capitalize on emerging market trends.
5. Streamlining Financial Document Processing
The heavy reliance on documentation—from loan applications to insurance claims—is eased by AI. Automated summarization and extraction of key insights reduce manual processing and accelerate overall document handling.
In essence, Generative AI is not just a technological advancement but a strategic asset that redefines operational efficiency and innovation in financial services. By harnessing its capabilities, institutions can navigate market complexities with agility and precision, ensuring they remain competitive in a dynamic industry.
Key Challenges in Implementing Generative AI in Financial Services
Although generative AIis transforming financial services, adoption of it presents certain difficulties. To use AI responsibly, financial organizations have to reach an agreement on moral challenges, security threats, and legal issues. Dealing with these challenges guarantees that AI-driven solutions improve rather than cause disturbance of financial operations.
Regulatory and Compliance Complexity
The financial industry operates within strict regulatory frameworks to protect consumers and maintain market integrity. AI-driven decision-making must comply with laws like GDPR, SEC regulations, and financial reporting standards. A key concern is the explainability of AI models—regulators and institutions need to understand how AI reaches its conclusions, especially when determining creditworthiness or detecting fraud. Failure to align AI operations with regulatory expectations can lead to legal repercussions and reputational damage.
Data Privacy and Security Risks
Generative AI uses massive datasets with sensitive financial data. Possible data breaches, unauthorized access, and compliance with data protection rules arise. AI-driven operations must be protected by improved encryption, access controls, and continual monitoring. When AI models generate synthetic data for risk modeling and consumer predictions, accuracy and data manipulation remain issues.
Lack of Explainability and Trust
The black-box nature of AI models makes it difficult for financial professionals to interpret AI-generated insights. Without clear explanations, stakeholders may hesitate to trust AI-driven decisions like investment forecasting, loan approvals, and fraud detection. The industry is increasingly focused on developing explainable AI frameworks that provide transparency while maintaining the efficiency of generative AI systems.
Integration with Legacy Systems
Many financial institutions operate on legacy infrastructure without the flexibility to support AI-driven automation. Integrating generative AI with existing banking platforms, risk management tools, and compliance systems requires significant investment and expertise. Without seamless integration, AI solutions may lead to operational inefficiencies rather than enhancing productivity.
Ethical Considerations and Bias
Ensuring AI models operate without bias is critical, especially in lending, hiring, and customer profiling areas. Poorly trained AI systems can inadvertently reinforce discrimination, leading to unfair financial outcomes. Institutions must actively audit and refine AI models to align with ethical AI standards, ensuring fairness and inclusivity in economic decision-making.
Addressing these challenges is essential for financial institutions looking to leverage generative AI effectively. Financial organizations can unlock AI's full potential while mitigating its risks by implementing robust governance frameworks, enhancing transparency, and strengthening cybersecurity measures.
Benefits of Generative AI in Financial Services
Generative AI redefines the financial sector by automating complex processes, improving accuracy, and enhancing customer experiences.
Improved Risk Management and Fraud Detection
Financial institutions rely on risk assessments to make informed decisions, whether approving loans or managing investment portfolios. Generative AI enhances these processes by analyzing vast datasets in real time, identifying patterns, and predicting potential risks more accurately. It strengthens fraud detection by recognizing unusual transactions, reducing false positives, and preventing financial crimes before they occur.
Enhanced Customer Experience
AI-powered chatbots and virtual assistants transform customer interactions in banking and financial services. These systems provide instant, personalized responses to queries, reducing wait times and improving user satisfaction. Additionally, AI-driven financial advisors generate tailored investment insights based on customer profiles, helping individuals and businesses make smarter financial decisions.
Optimized Trading Strategies and Market Predictions
In financial markets, timing and accuracy are crucial. Generative AI enables predictive analytics that helps traders and investment firms simulate different market conditions and adjust strategies accordingly. AI models process historical and real-time market data, offering insights that enhance algorithmic trading and portfolio management. This results in better-informed investment decisions and reduced exposure to market volatility.
Automated Compliance and Regulatory Reporting
Regulatory compliance is critical for financial institutions, requiring meticulous documentation and adherence to global financial laws. Generative AI automates compliance reporting by extracting key insights from legal documents, monitoring transactions for anomalies, and generating audit-ready reports. This reduces human effort while ensuring financial institutions meet regulatory standards efficiently.
Cost Efficiency and Operational Productivity
By automating time-consuming tasks such as document processing, risk analysis, and financial modeling, generative AI significantly reduces operational costs. Banks and financial firms streamline their workflows, allocate resources more effectively, and minimize human errors. The ability to process large volumes of data quickly translates into faster decision-making and improved overall productivity.
Scalability and Continuous Learning
Unlike traditional systems that require constant manual updates, generative AI continuously learns from new data, adapting to emerging trends and challenges. This allows financial institutions to scale AI-driven solutions effortlessly, ensuring they stay ahead in an ever-evolving financial landscape. The ability to process dynamic market conditions and customer behaviors ensures financial services remain relevant and competitive.
The integration of generative AI is paving the way for a more intelligent, secure, and efficient financial ecosystem. As the technology continues to evolve, financial institutions that embrace AI-driven innovations will lead the industry in providing faster, safer, and more personalized financial services.
Best Practices for Implementing Generative AI in Financial Services
Successfully deploying Generative AI in financial services means balancing innovation with robust security and compliance. By adhering to best practices, institutions can unlock value while managing risk effectively.
- Regulatory Compliance and Ethical AI Usage: Ensure AI solutions adhere to global standards (GDPR, SEC, Basel III) and incorporate explainability tools for transparent decision-making. Establish ethics committees to monitor bias and uphold fairness in areas like loan approvals and fraud detection.
- Data Security and Privacy: Protect sensitive financial data with end-to-end encryption, multi-factor authentication, and anomaly detection. Leverage privacy-preserving methods like federated learning and align with ISO 27001 and SOC 2 standards to minimize security risks.
- Model Interpretability and Explainability: Avoid black-box AI by using techniques such as SHAP and LIME. This approach guarantees that AI-driven insights support human oversight in critical functions like risk assessment and investment advisory.
- Seamless Integration with Financial Systems: Deploy cloud-based AI solutions that integrate effortlessly with legacy systems, risk management frameworks, and trading platforms. Utilize APIs and orchestration layers to ensure smooth, uninterrupted automation.
- Continuous Model Improvement and Monitoring: Adopt reinforcement learning and regular performance audits to adapt AI models to changing market dynamics and evolving compliance requirements. Continuous monitoring ensures models remain fair, accurate, and aligned with financial goals.
- Aligning AI with Business Strategy: Connect AI initiatives directly to strategic objectives—enhancing fraud detection, automating compliance, and improving customer engagement. Establish measurable KPIs, such as cost reduction and improved detection efficiency, to gauge success and refine strategies.
Embracing these best practices enables financial institutions to harness the full potential of Generative AI. At Tredence, we believe that a disciplined approach to AI implementation mitigates risks and drives transformative growth and competitive advantages.
Generative AI in Financial Services: Transforming Operations with Use Cases and Applications
Generative AI is rapidly reshaping the financial landscape by driving innovation, enhancing decision-making, and streamlining processes across risk management, customer engagement, compliance, and trading. At Tredence, we believe that successful AI deployment requires a balanced approach—one that marries transformative technology with robust security, compliance, and operational integration.
- Enhanced Risk Management and Fraud Detection: AI systems analyze historical data, detect real-time anomalies, and even continuously generate synthetic fraud scenarios to refine detection algorithms. Continuous monitoring, reinforcement learning, and regular performance audits ensure that these models remain accurate and adaptive.
- Personalized Customer Engagement and Financial Services: By processing vast datasets, AI delivers tailored financial advice, dynamic portfolio management, and real-time chat support, transforming customer experiences. Aligning these initiatives with strategic KPIs such as cost reduction and customer satisfaction guarantees measurable business impact.
- Automated Compliance and Regulatory Processes: AI streamlines compliance through automated extraction of key insights from regulatory documents, efficient KYC/AML checks, and audit report generation. Adhering to global standards like GDPR, SEC, and Basel III—and integrating explainable AI techniques—ensures that outcomes are transparent and ethically sound.
- Seamless Integration and Scalability: To avoid disruptions, AI solutions should integrate smoothly with legacy systems via APIs and orchestration layers, leveraging cloud-based platforms for scalability. This approach ensures that automation enhances rather than disrupts existing financial operations.
- Data Security and Model Interpretability: Given the sensitivity of financial data, robust security measures—such as end-to-end encryption, multi-factor authentication, and privacy-preserving techniques like federated learning—are essential. Employing interpretability tools like SHAP and LIME fosters transparency, ensuring that regulators and stakeholders can confidently validate AI-driven decisions.
Financial institutions can leverage Generative AI to unlock substantial value while mitigating risk by fusing innovation with discipline. At Tredence, we’re committed to guiding organizations through this transformation, ensuring technology powers efficiency and drives sustainable growth. Embracing these integrated practices paves the way for a competitive, agile, and future-ready financial ecosystem.
Examples of Generative AI in Financial Services
Generative AI is already significantly impacting the financial sector, with leading institutions deploying AI-driven solutions to improve operational efficiency, enhance customer experience, and strengthen risk management. Below are real-world examples of how financial organizations are successfully integrating Generative AI.
Fraud Detection and Security Enhancement
Banks and payment providers use Generative AI to detect fraud in real time, minimize false positives, and improve cybersecurity. AI models discover new fraud tendencies to improve detection.
- Global Banks: Institutions like JPMorgan Chase and HSBC use AI-powered fraud detection systems that analyze transaction patterns and flag suspicious activities with greater precision.
- Payment Processors: Companies like PayPal and Visa employ AI to detect unauthorized transactions, reducing fraud-related financial losses.
AI-Driven Customer Support
Financial service companies are using AI-powered chatbots and virtual assistants to answer questions, process transactions, and offer advice 24/7. AI assistants customize responses based on consumer history and preferences.
- Banking Services: Wells Fargo and Bank of America use AI chatbots like Erica to offer instant financial guidance, helping customers check balances, track spending, and schedule payments.
- Wealth Management: Robo-advisors such as Betterment and Wealthfront utilize AI to provide personalized investment strategies based on individual financial goals.
Regulatory Compliance and Risk Management
Regulatory compliance remains a top priority for financial institutions, and AI plays a crucial role in automating compliance processes and reducing regulatory risks.
- Audit and Compliance Automation: Deloitte and PwC employ AI models to monitor transactions, detect compliance breaches, and generate regulatory reports efficiently.
- AML (Anti-Money Laundering) Monitoring: AI-driven systems from FICO and NICE Actimize analyze suspicious financial activities, ensuring compliance with AML regulations.
Algorithmic Trading and Market Analysis
Hedge funds and investment firms use Generative AI to predict market trends, execute algorithmic trading strategies, and optimize portfolio management. AI models process massive datasets to identify profitable trading opportunities.
- High-Frequency Trading: Firms like Renaissance Technologies and Citadel use AI algorithms to execute trades milliseconds, maximizing gains and minimizing risks.
- Market Sentiment Analysis: AI-powered analytics platforms like AlphaSense assess financial news, social media sentiment, and economic reports to inform trading strategies.
Loan Underwriting and Credit Decisioning
AI is transforming lending by enhancing credit risk assessments and streamlining loan approvals. Financial institutions use AI-generated models to assess borrowers' financial behavior more accurately.
- AI-Enabled Loan Approvals: Companies like Upstart and Zest AI leverage AI-driven underwriting systems that evaluate a borrower’s creditworthiness beyond traditional credit scores.
- Mortgage Automation: AI-powered platforms like Blend help banks and mortgage providers automate home loan applications, reducing processing times and improving accessibility.
Insurance Claims Processing and Risk Evaluation
Insurers are integrating AI to automate claims assessments, detect fraudulent claims, and refine policy pricing models. AI-generated insights help insurers reduce delays and enhance customer trust.
- Claims Automation: Companies like Lemonade use AI bots to process claims within minutes, eliminating lengthy manual reviews.
- Risk Modeling: Insurers like Allstate and AXA employ AI to analyze policyholder data and predict potential risks, optimizing coverage offerings.
Personalized Financial Planning and Wealth Management
Wealth management firms and financial advisors use AI to generate customized financial plans, optimize retirement savings, and provide data-driven investment recommendations.
- AI-Driven Financial Advisory: Morgan Stanley and Goldman Sachs integrate AI models that offer tailored investment advice based on historical market performance and client preferences.
- Portfolio Optimization: AI-powered platforms like BlackRock’s Aladdin provide institutional investors with AI-generated risk assessments to optimize asset allocation.
Driving Financial Innovation with Generative AI: Tredence’s Approach
Generative AI is changing financial services, compliance, fraud detection, and financial services. Financial companies need artificial intelligence solutions guaranteeing accuracy, security, and scalability to maximise their potential.
With AI-driven automation, risk management, and predictive analytics, Tredence helps financial institutions to guarantee flawless connection with current systems. Emphasizing explainability and ethical artificial intelligence, Tredence enables companies fully utilize AI while preserving compliance and confidence. Take the next step in your AI-driven financial transformation—contact Tredence today!
FAQs
1. What is the responsible use of Generative AI in financial services?
Responsible use of Generative AI in financial services involves ensuring fairness, transparency, and compliance with industry regulations. AI models should be explainable, free from biases, and aligned with data privacy laws like GDPR and SEC guidelines. Financial institutions must establish ethical AI frameworks, conduct regular audits, and implement security measures to prevent AI-driven risks such as fraudulent activities or biased decision-making.
2. How does Generative AI assist with financial decision-making?
Generative AI enhances financial decision-making by analyzing large datasets, identifying trends, and generating predictive insights. It supports financial institutions in risk assessment, investment strategy optimization, fraud detection, and credit scoring. AI-driven automation improves accuracy, reduces manual workload, and enables real-time financial analysis for more data-driven and strategic decision-making.
3. What role does Generative AI play in security within the financial services industry?
Generative AI strengthens financial security by detecting fraud, monitoring transactions for anomalies, and enhancing cybersecurity protocols. AI models analyze behavioral patterns to identify suspicious activities and prevent financial crimes. Institutions also use AI for anti-money laundering (AML) compliance, secure authentication methods, and risk mitigation to protect customer data and ensure regulatory adherence
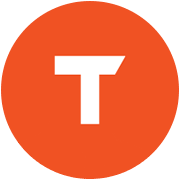
AUTHOR - FOLLOW
Editorial Team
Tredence
Next Topic
Unlocking Personalization: How Businesses are Tailoring Experiences to Drive Success
Next Topic