
Imagine a global retailer struggling to forecast demand accurately and, therefore, facing frequent stockouts and overstocked inventory. This can be resolved using generative AI-based data analytics, which transforms raw data into actionable insights, thus optimizing inventory levels and increasing revenue.
This is just one example of how generative AI for data analytics is revolutionizing businesses worldwide. By 2026, 80 percent of organizations worldwide are expected to integrate GenAI into their workflows and applications, thus establishing its rapidly growing influence (Source: Gartner).
Considering the unprecedented volume of data, traditional analytics often fails to deliver actionable insights at the required speed. This is where Generative AI steps in. It automates complex analysis, identifies hidden patterns, and offers hyper-accurate predictions across vast datasets in real time, thereby helping organizations elevate decision-making and drive business efficiency.
This article explores how generative AI is changing data analytics, highlighting use cases, industries, and tools that will shape its future.
What is the Role of Generative AI in Data Analytics?
Generative AI in data analytics leverages AI models to create insights, patterns, and predictions by learning from existing datasets. It automates tasks such as data preprocessing, anomaly detection, and trend forecasting, enabling businesses to derive deeper, faster, and more actionable insights for decision-making.
For instance, generative AI can model "what-if" scenarios for the prediction of outcomes, an ability increasingly being used across industries such as finance, healthcare, and retail. This not only enhances planning but also makes businesses agile to act as per market dynamics.
Let us now understand how generative AI seamlessly integrates with data analytics.
Understanding Generative AI
Generative AI refers to algorithms capable of creating new content or patterns based on input data. Unlike traditional AI models that analyze data, generative AI produces outputs such as text, images, or simulations. It identifies trends, predicts behaviors, and automates data preparation in the context of analytics.
Generative AI is a subfield of artificial intelligence that focuses on creating new content—be it text, images, or data—based on existing datasets. Advanced models, such as GPT, are used to simulate human-like creativity and problem-solving.
Generative AI is not a one-size-fits-all solution; it does not inherently "understand" context or make ethical judgments without human oversight. Generative AI model largely relies on quality, diversity, and relevance of the data it is trained on.
In 2022, OpenAI's GPT-4 highlighted generative AI’s ability to create coherent narratives and solve complex data problems. Thus, it exposed the gap between raw data and actionable insights. Generative AI can assist businesses in predicting customer needs by simulating possibilities and optimizing their workflows.
The following section describes how data analytics has developed into the cornerstone of modern business intelligence.
Role of Data Analytics
Data analytics involves processing and interpreting data to uncover patterns, trends, and actionable insights. It has become indispensable in today’s data-driven economy, with organizations using analytics to optimize operations, enhance customer experiences, and drive innovation.
Generative AI takes this a step further by automating labor-intensive tasks such as data cleaning and visualization.
According to a 2024 study, companies leveraging AI-driven analytics reported 2.5x higher revenue growth from 2019 to 2023 compared to their peers with the lowest operations readiness. Businesses can drive deeper insights faster by integrating generative AI, thus setting the stage for transformative outcomes (Source: Accenture).
Let us now explore the benefits of generative AI in data analytics and the impact of generative AI on efficiency, accuracy, and innovation.
Benefits of Generative AI in Data Analytics
Generative AI is revolutionizing data analytics, enabling the discovery of new insights and steering business decisions toward greater intelligence.
Generative AI is helping organizations become more effective and obtain better returns on their investments in data, due to its ability to simplify complex tasks and reveal hidden trends.
Major advantages of generative AI include:
- Enhanced Data Preparation and Cleaning: Data preparation often consumes 50 to 70 percent of analysts' time. Generative AI automates this process by efficiently identifying inconsistencies, filling gaps, and structuring data (Source: IBM).
- Improved Predictive Analytics: Generative AI excels in creating accurate predictive models. It analyzes historical data to forecast trends, enabling businesses to make proactive decisions.
- Real-time Insights: Generative AI offers real-time insights by processing large datasets instantaneously. This is particularly beneficial in industries such as finance, where rapid decision-making is critical.
- Scalability and Efficiency: Generative AI allows businesses to scale their analytics operations without additional resources. AI-powered cloud platforms can handle massive datasets, ensuring consistent performance even as data volumes grow.
Along with the benefits mentioned above, generative AI in data analytics presents challenges that must be addressed to ensure accurate, reliable, and ethical insights while preserving transparency and trust in the decision-making process.
Challenges in Implementing Generative AI in Data Analytics and How to Overcome Them
Implementing generative AI in data analytics has transformative potential; however, it comes with its own set of challenges. Proactively addressing these challenges will enable companies to streamline the adoption process and fully maximize the value of AI integrated into their operations.
1. Data Privacy Concerns
Generative AI deals with sensitive data and requires strong security measures to prevent data breaches and maintain compliance.
According to a 2024 report, 40 percent of businesses identified data privacy as a key challenge to AI adoption. To overcome this challenge, organizations must adopt robust encryption methods, implement access controls, and comply with regulations such as GDPR and CCPA.
Leveraging synthetic data for training can also help protect sensitive information while maintaining data utility. Regular security audits help ensure compliance with evolving standards and reduce potential risks (Source: PureAI).
2. Algorithmic Bias
Generative AI models, when trained on biased datasets, run the risk of perpetuating unfair outcomes such as discriminatory predictions. This could undermine trust and ultimately hamper decision-making.
Companies need to factor in diverse, representative training datasets, regular auditing of models, and inclusion of fairness metrics in model evaluations to mitigate this risk. Interdisciplinary teams reviewing AI outputs can also help reduce bias and improve accuracy. Transparent reporting of the AI decision-making process can boost stakeholder confidence.
3. High Implementation Costs
The cost of developing and deploying generative AI systems, including the required infrastructure, tools, and talent, can be prohibitively expensive. Companies can lower these costs by starting small, focusing on high-impact use cases, and using pre-trained AI models from OpenAI or Google.
They can also collaborate with technology partners to access scalable cloud-based solutions that lower upfront costs. Over time, the efficiency and insights provided by Gen AI typically yield significant returns, transforming it into a strategic asset.
4. Integration Issues
Integration of generative AI in the legacy system is technically complex and resource intensive. It generally requires an upgrade in infrastructure and skillsets. Organizations must follow a phased implementation approach, starting with pilot projects, to identify integration gaps.
The use of middleware solutions bridges the compatibility divide, enabling legacy systems to work with advanced AI platforms. Workforce training ensures that teams are equipped to handle new tools and technologies effectively.
By being proactive with these challenges, organizations can unlock the full potential of generative AI for data analytics to drive efficiency, accuracy, and innovation in their operations.
Use Cases of Generative AI in Data Analytics: Real-world Success Stories
Generative AI is revolutionizing how businesses process data and how companies across various industries achieve tangible, real-world results.
Let us take a closer look at some of the real-world success stories that prove generative AI has the power to resolve critical challenges within data analytics.
Fraud Detection: Mastercard's Real-time Security Enhancement
The surge in cyber fraud cases worldwide has prompted Mastercard to leverage generative AI to strengthen its fraud detection capabilities, doubling the speed at which it identifies potentially compromised cards and enhancing the security of the payment ecosystem.
Fraudsters steal millions of payment card numbers through methods such as spyware, malware, and card skimming. They then sell partial card details to illegal websites, posing significant risks to financial institutions and consumers alike.
Mastercard uses a generative AI system to predict the full details of potentially compromised cards within its network, thus enabling banks to take swift action.
The system scans billions of transactions and millions of interactions with merchants at unprecedented speed to detect the most complex patterns of fraud in real time-achieved through results such as the following:
- Doubled rate of compromised card detection.
- Decrease in false positives by up to 200 percent, with better accuracy in detection.
- 300 percent increase in the speed of detecting at-risk merchants.
These innovations allow Mastercard to alert banks with increased accuracy and speed as soon as fraud is detected. Thus, banks can block and reissue compromised cards, thereby stopping further unauthorized transactions and helping regain customer confidence.
By integrating generative AI into its Cyber Secure suite, Mastercard showcases its commitment to combating fraud and enhancing the safety of the global payments ecosystem for businesses and individuals (Source: MasterCard).
Predictive Maintenance: Siemens' Downtime Reduction
Siemens enhanced its Senseye Predictive Maintenance solution by integrating generative AI, creating a robust system that reduces downtime and addresses maintenance skill shortages. This innovative functionality transforms traditional machine learning, enabling intuitive, conversational interactions that streamline maintenance decision-making.
The system analyzes machine and maintenance data, directing attention to critical issues and suggesting actionable solutions. Generative AI scans past cases, even in multiple languages, to offer insights and propose prescriptive maintenance strategies. It helps organizations make efficient, informed decisions by contextualizing data and factoring in maintenance protocols.
BlueScope, an Australia-based steel manufacturer, leveraged this advancement to scale knowledge-sharing globally. Colin Robertson, Digital Transformation Manager at BlueScope, noted that Senseye is “a catalyst for change” in their operations.
By securely processing data in a private cloud, Siemens’ solution combines machine learning’s predictive capabilities with generative AI’s adaptability, thus ensuring reduced downtime and optimized maintenance processes (Source: Siemens).
These case studies underscore the potential of generative AI to revolutionize data analytics by addressing specific industry pain points. Next, we will explore the tools and technologies that empower businesses to harness the full potential of generative AI.
Popular Generative AI Tools for Data Analytics
Specialized tools have been designed for GenAI-based analytics, each one serving a specific purpose, benefit, and use case. Let us explore some of the leading tools available today.
Tableau GPT
Tableau's generative AI capability enables users to create data visualizations using natural language queries, making complex data exploration simple.
Benefit: Tableau GPT makes it possible to convert natural language queries into complex visualizations, thus making data accessible to non-technical stakeholders.
When to use: It is ideal for businesses seeking to empower teams with quick insights without requiring deep technical skills, particularly through interactive reporting and dashboards.
Azure OpenAI Service
GPT models now reside under the Azure architecture to perform deep data analysis at scale, promising secure solutions that are tailor-made for enterprises.
Benefit: Azure OpenAI Service provides scalable enterprise solutions, deep data analysis with OpenAI embeddings, and comprehensive security and compliance features.
When to use: It is used when large-scale organizations require a cloud-based, well-secured system to work on vast datasets or advanced analytics based on natural languages.
DataRobot
This platform automates the workflows of machine learning, thereby allowing analysts to easily build and deploy predictive models.
Benefit: DataRobot saves time and improves accuracy in the machine learning lifecycle, starting from building models to deployment.
When to use: Use DataRobot when predictive modeling and automation are required, especially when an organization needs end-to-end solutions without extensive coding expertise.
KNIME
KNIME's open-source platform integrates generative AI capabilities for advanced analytics, offering flexibility and scalability for businesses.
Benefit: KNIME is an open-source platform with customizable workflows that integrates generative AI for versatile data analytics.
When to use: It is ideal for companies that require a low-cost, adaptable tool for complex data transformations and exploratory analysis.
These tools open the door to further advancements, which will be discussed in the next section on how generative AI enhances analytics capabilities to drive smarter decision-making and optimize business operations.
How Generative AI Integrates with Analytics Solutions to Build Better and Efficient Systems
Generative AI integrates with analytics solutions to revolutionize data-driven decision-making. Advanced language models and neural networks power generative AI, which enhances traditional analytics with predictive insights, natural language processing, and automated data generation.
This integration allows businesses to dig deeper into insights, optimize processes, and deliver accurate forecasts. Generative AI augments analytics by automating data preparation—something that is both time-consuming and laborious.
Tools such as Tableau GPT and DataRobot use AI to clean, structure, and enrich raw data, reducing manual effort and improving data quality. Furthermore, generative AI models synthesize large datasets, generating scenarios for predictive analytics that forecast trends and risks.
Generative AI powers real-time analytics through integration with Internet of Things (IoT) platforms and data lakes. For instance, retail companies can analyze point-of-sale data and customer behavior in real time to optimize inventory and personalize marketing.
Similarly, in the healthcare sector, generative AI combines patient data with advanced analytics to predict medical outcomes and recommend treatments with unmatched precision.
AI-driven natural language interfaces democratize analytics by allowing non-technical users to access data through simple queries. Combining human intuition with the computational power of generative AI enables organizations to arrive at actionable insights more rapidly, thus driving innovation and speed.
Integration in this manner thus underscores the transformative potential of generative AI in building analytics solutions that are not only efficient but also precise and impactful.
Future Trends in Generative AI and Data Analytics
Generative AI is reshaping the future of data analytics, making opportunities that were previously unimaginable a reality. From increasing transparency to driving real-time insights, the following emerging trends will redefine how organizations harness data for innovation and growth:
1. Explainable AI (XAI)
The processes of AI systems must be transparent and understandable as these systems become central to decision-making. Explainable AI (XAI) sheds light on how AI models come up with certain outputs for the "black box" problem.
For example, XAI can break down a loan approval decision by analyzing factors such as the applicant's income, credit history, and risk scores. This makes users more likely to accept the system's way of doing things. It also brings in more accountability, as organizations can prove and improve model validity through precise feedback.
2. AI-powered Decision Support Systems
Generative AI is likely to revolutionize strategic decision-making by simulating different scenarios and analyzing possible outcomes. It can provide executives with a clearer view of risks and opportunities, enabling data-driven planning.
Generative AI can be used in investment firms to simulate market dynamics for portfolios to optimize asset allocation. This will help decision-makers adopt proactive strategies with higher precision by visualizing complex trends of data and forecasting future scenarios.
3. Integration with IoT
The fusion of generative AI and IoT enables real-time analytics on data generated by connected devices. For instance, in a smart factory, AI can analyze sensor data to predict when machines need maintenance, thus lowering downtime.
Generative AI in logistics can use real-time traffic and weather information to optimize the routes taken in delivering packages, thus driving better efficiency. Similarly, health organizations can use wearable IoT devices and AI to monitor patient vitals and predict health risks.
Such integration not only enhances operational efficiency but also fosters innovation across industries by enabling dynamic, data-rich ecosystems. Organizations can strike a balance between innovation and practical implementation by adopting Tredence’s generative AI solutions.
Acing Your GenAI Initiatives with Tredence’s Solutions
Generative AI is revolutionizing data analytics, enabling businesses to uncover deeper insights that enhance decision-making and foster innovation.
As the technology continues to evolve, organizations that embrace generative AI will be best placed to thrive in a data-driven world. By overcoming adoption barriers and leveraging cutting-edge tools, you can transform your business’s analytics capabilities, setting new benchmarks for efficiency and growth.
Tredence allows businesses to reap the full potential of generative AI in data analytics by addressing their unique challenges through tailored solutions. From designing powerful GenAI strategies to implementing seamless GenAI solutions and optimization, Tredence ensures that organizations achieve tangible, measurable results.
With expertise in data privacy, bias mitigation, and legacy system integration, Tredence is your trusted partner in navigating the complexities in the adoption of generative AI. Ready to transform your data analytics journey? Visit Tredence today to explore its innovative solutions that drive actionable insights and competitive advantage.
FAQs
1. Can generative AI help uncover insights that traditional analytics might miss?
Yes, generative AI can identify hidden patterns and relationships in complex datasets that traditional analytics might overlook. It helps businesses gain deeper insights and make more informed decisions by generating simulations, predictions, and new data representations.
2. How does generative AI handle unstructured data in analytics?
Generative AI processes unstructured data—text, images, and audio—by using advanced models to recognize patterns, summarize information, and generate meaningful insights. It can convert raw data into structured formats, making it easier for businesses to analyze and act upon.
3. What’s the difference between traditional data analytics and generative AI-driven analytics?
Traditional analytics focuses on analyzing historical data to identify trends and support decision-making, often relying on predefined models. Generative AI goes beyond by creating new data, predicting scenarios, and providing deeper contextual understanding, making it valuable for innovation and complex problem-solving.
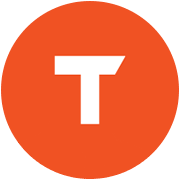
AUTHOR - FOLLOW
Editorial Team
Tredence
Next Topic
The Essential Guide to Effective Demand Forecasting: How it Drives Retail Profitability
Next Topic