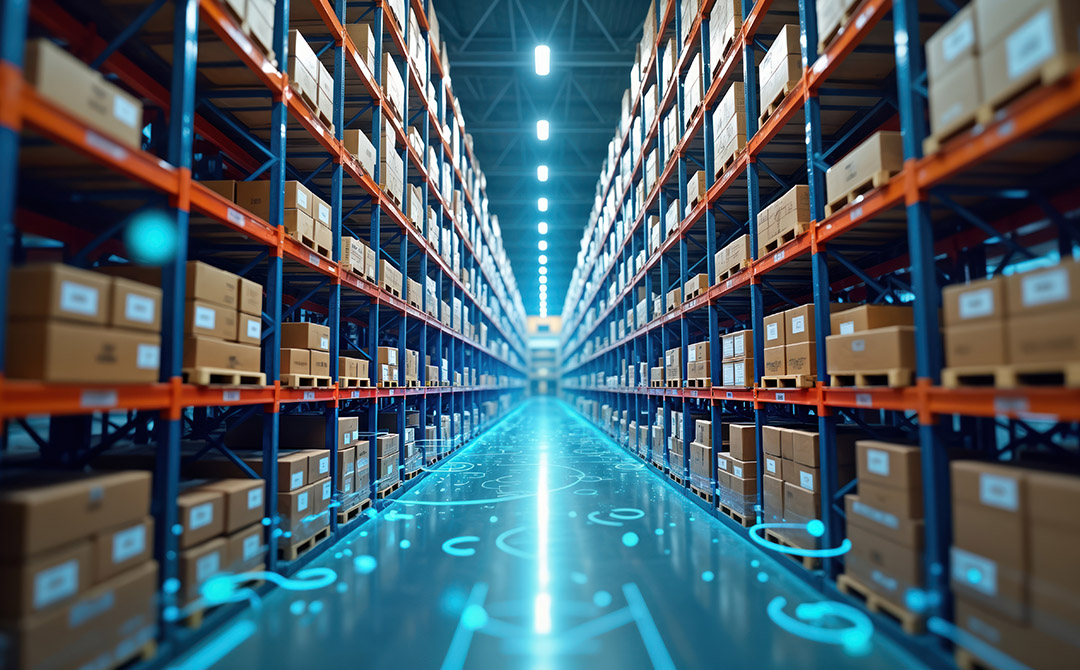
Imagine a retailer gearing up for the holiday season but miscalculating demand trends, resulting in overflowing warehouses filled with unsold items or empty shelves for sought-after products. This not only strains profitability but also disappoints customers—driving them to competitors.
Retail demand forecasting enables businesses to anticipate demand changes, ensure optimal product availability, reduce waste, and drive both profitability and customer satisfaction.
This article explores retail demand forecasting, examining its significance, various types, benefits, challenges, and best practices—illustrated with real-world examples—to highlight its transformative potential.
What is Retail Demand Forecasting?
Retail demand forecasting leverages historical data, market trends, and advanced analytics to project consumer demand for a product or service accurately. Its predictive capability empowers retailers to make informed decisions on inventory levels, pricing strategies, demand planning, and supply chain optimization.
A retail chain may use historical sales data from previous holiday seasons to forecast which products will experience a surge in demand during the upcoming holiday period.
Driven by technologies such as Machine Learning (ML) and Artificial Intelligence (AI), demand forecasting models now offer a higher level of granularity in understanding consumer behavior. These insights boost business agility as market dynamics continue to shift.
Importance of Retail Demand Forecasting
Accurate retail demand forecasting is critical to mastering the complexities of modern retail. Without it, businesses are prone to overstocking or understocking, which can result in significant financial losses and potential damage to customer relationships.
Retail demand forecasting plays a key role in:
- Inventory Management: Accurate forecasting ensures that retailers maintain the right inventory levels, thus reducing the risk of overstocking or stockouts. This directly enhances revenue by reducing storage costs and preventing lost sales due to unavailability.
- Customer Satisfaction: Accurate forecasting allows retailers to consistently meet customer demand, fostering trust and building long-term brand loyalty. Consistently having popular products in stock creates a smooth shopping experience, building customer confidence and driving repeat sales.
- Achieving Cost Efficiency: Accurate demand forecasting streamlines operations and saves costs associated with storage, logistics, and unsold stock. It frees up resources that can be reinvested into growth initiatives or innovation.
- Strategic Decision-making: Demand forecasting helps retailers refine pricing strategies, optimize promotions, and plan product launches. Informed decisions improve overall competitiveness and ensure a closer product-market fit.
- Obtaining Competitive Advantage: The ability to anticipate and respond to consumer needs distinguishes businesses in a cluttered marketplace. Proactive demand planning empowers retailers to become industry leaders, enabling them to thrive in dynamic market conditions.
Integrating retail demand forecasting into operations not only raises retailers’ profitability but also consolidates their market position. Thus, demand forecasting is a must-have tool for retail business success.
Types of Retail Demand Forecasting
Understanding the different types of retail demand forecasting enables businesses to select the most suitable methodology for their unique needs. Each type offers specific benefits for handling diverse market conditions and improving retail demand planning.
Different types of retail demand forecasting include:
- Quantitative Forecasting: This method utilizes statistical models and historical sales data to predict demand. It is particularly effective for businesses with rich data histories and consistent patterns, such as grocery stores or consumer electronics retailers.
Techniques such as time-series analysis and regression models provide precise predictions. However, these methods may struggle with unexpected market shifts or entirely new products.
- Qualitative Forecasting: This method relies on expert opinions, market insights, and customer surveys to forecast demand. It is ideal for launching new products or entering unfamiliar markets where historical data is unavailable.
The method provides valuable directional insights. However, its subjective nature can introduce bias, thus limiting precision.
- Short-term Forecasting: This method focuses on predicting demand for the upcoming weeks or months. Retailers leverage it to manage inventory for short sales cycles or seasonal peaks, such as back-to-school supplies or holiday decorations. The short-term forecasting method can optimize immediate inventory requirements; however, it may lack the foresight required for long-term planning.
- Long-term Forecasting: This method examines demand trends over a year or more, facilitating strategic decisions such as market expansion, product development, or large-scale investments.
Its comprehensive view of future demand provides valuable insights for growth initiatives. However, the approach may require regular updates to adapt to changing market dynamics.
- Causal Forecasting: This method analyzes external factors, such as economic conditions, weather, seasonality, and marketing campaigns, to predict demand. It is ideal for fashion and outdoor equipment industries, where external influences significantly impact sales. Its accuracy depends on the quality of input data and modeling.
By understanding the nuances of these forecasting methods, businesses can tailor their strategies to specific needs, enhancing both accuracy and relevance. Combining multiple approaches often yields the most robust and actionable insights for retail demand forecasting.
Benefits of Retail Demand Forecasting
Retail demand forecasting enables retailers to navigate a dynamic and fast-changing market environment. Business forecasting techniques offer operational efficiency and customer satisfaction and help retain competitiveness.
Advantages of implementing retail demand forecasting include:
-
Optimized Inventory Management: Accurate demand forecasting allows retailers to maintain optimal inventory levels and predict sales trends. Understanding demand for a specific product helps retailers avoid overstocking and understocking, preventing capital from being tied up and minimizing lost sales and customer dissatisfaction due to unmet needs.
Advanced forecasting tools analyze historical sales data, seasonality, and market trends to strike the right balance between supply and demand, thus ensuring inventory efficiency.
-
Improved Customer Satisfaction: Customer satisfaction plays a critical role in competitive retailing. Retailers can ensure product availability through demand forecasting, thus preventing stockouts and delays in delivery.
Companies can stock popular items in sufficient quantities during the holiday seasons. This fosters customer loyalty and improves the shopping experience, directly contributing to increased brand trust and repeat business.
-
Cost Reduction: Demand forecasting minimizes overproduction, excessive inventory, and emergency procurement costs. Retailers can reduce waste and streamline logistics.
Predictive models can help identify slow-moving products, enabling targeted discounting or marketing campaigns to clear inventory more efficiently. According to a study, AI-based forecasting techniques could help decrease costs by 10 percent to 15 percent (Source: Mckinsey).
-
Improved Supply Chain Efficiency: Demand forecasting allows for better communication and coordination in the supply chain. Accurate predictions enable suppliers, manufacturers, and logistic partners to plan their operations more effectively.
This synchronization reduces lead times, prevents bottlenecks, and assures timely delivery of products. Forecasting data in supply chain management allows retailers to ensure a smooth flow of goods from production to customers.
-
Adaptability to Market Trends: Retail demand forecasting allows a business to react quickly to market changes. For instance, several retailers used demand forecasting to identify changes in customer behavior and adapt their strategies accordingly during the e-commerce surge.
-
Better Financial Planning: Demand forecasting provides the insights necessary for accurate revenue projections and effective budget planning.
Retailers can leverage projected sales data to optimize resource allocation, set achievable sales targets, and monitor financial performance. This proactive financial management leaves less room for uncertainty and paves the way for sustainable growth.
Accurate demand forecasting improves the management of perishables in grocery retail, ensuring that fresh stock is maintained without surplus. This minimizes not only financial loss but also supports sustainability initiatives through waste reduction.
These retail demand forecasting advantages allow businesses to balance operational efficiency and customer satisfaction. Advanced analytics, combined with proactivity, allows retailers to remain resilient and competitive in the rapidly-changing market landscape.
Challenges in Retail Demand Forecasting
Retail demand forecasting, while highly valuable, presents several challenges. These arise from the constantly evolving consumer behavior, shifting market trends, and external factors that can disrupt supply chains.
Addressing these challenges requires a strategic approach to accuracy and reliability in forecasting:
-
Poor Data Quality: Inaccurate, inconsistent, or incomplete data adversely affects forecasting accuracy. Retailers often find it challenging to integrate data from different sources, such as sales, inventory, and market research, resulting in gaps or errors within the dataset.
This challenge affects inventory management, resulting in either overstocking or stockouts, which in turn hampers profitability. Businesses must invest in data cleaning and validation processes and advanced data integration tools to ensure consistency and reliability.
-
Demand Volatility: Market trends, economic conditions, or unforeseen events may impact consumer preferences. For instance, retailers were caught unawares by the sudden swing in demand for daily essentials during the COVID-19 pandemic. As a result, relying solely on historical information becomes impractical.
Real-time analytics and adaptive forecasting models enable quick and precise adjustments based on current market conditions and shifting consumer sentiment.
- Supply Chain Disruptions: External factors such as natural disasters, geopolitical tensions, or transportation bottlenecks may cause supply chain disturbances. Such a disruption creates a rippling effect on demand forecast, resulting in delayed deliveries or unsatisfied customers.
Retailers must mitigate this challenge by using predictive tools that incorporate external variables into the forecast and develop strong contingency plans that ensure supply chain resilience during unforeseen disruptions.
-
Integration of Technologies: Advanced forecasting tools powered by AI and ML require significant financial investment and specialized technical expertise. Retailers often face challenges when integrating these technologies into their existing systems, which can delay implementation and reduce their effectiveness.
Businesses must adopt a phased approach to technology integration, prioritize training programs for staff, and partner with experienced technology providers to ensure seamless adoption.
-
Seasonal Variations: Retail demand, such as back-to-school shopping or holiday sales, can be seasonal. Traditional models often fail to capture these trends accurately, leading to misaligned inventory levels.
To effectively manage seasonal variations, retailers must implement specialized forecasting models that are regularly updated to reflect changing trends and customer preferences.
By proactively addressing these challenges, retailers can enhance demand forecasting accuracy and ensure their operations remain efficient and focused on customer needs.
Retail Demand Forecasting Best Practices
Effective retail demand forecasting entails the adoption of practices that improve accuracy, adaptability, and alignment with business objectives.
The following best practices will enable retailers to optimize operations, improve customer satisfaction, and stay ahead of the competition.
-
Invest in Data Quality Management: High-quality data is the foundation of accurate demand forecasting. Retailers need strong data cleaning, validation, and integration processes in place to eliminate inaccuracies and inconsistencies.
For example, consolidating data from sales channels, inventory systems, and market research ensures a holistic view of demand. This can be further enhanced by using advanced tools for real-time data monitoring to improve forecast reliability.
-
Leverage Advanced Forecasting Tools: AI-driven forecasting and machine learning algorithms are valuable tools for recognizing patterns and predicting demand. These tools analyze large datasets to provide insights that traditional models might miss.
Retailers can leverage these technologies to identify emerging trends, anticipate demand surges, and respond quickly to changes. Investing in user-friendly platforms ensures teams can extract the maximum value without requiring extensive technical expertise.
-
Adopt Real-time Analytics: Retailers can keep track of demand changes by continuously monitoring live data analytics. For example, e-commerce activity tracking enables dynamic inventory allocation during a flash sale. Real-time analytics mitigates risks pertaining to stale or static forecasts.
-
Account for External Factors: External events such as economic trends, weather conditions, and geopolitical events may affect demand. If integrated into the forecasting models, such variables provide a clearer picture of possible market fluctuations.
For example, a retailer anticipating a cold snap may begin stocking up on winter apparel. Scenario planning and predictive modeling tools enable businesses to stay proactive in the face of such uncertainties.
-
Segment Forecast by Product and Location: Demand patterns vary significantly across product categories and geographic regions. Retailers should create segment-specific forecasts to develop customized strategies for each group.
A coastal store, for instance, would require more sunscreen, whereas an urban location would prioritize office supplies. Segmenting forecasts allows for better inventory allocation and reduces waste.
-
Collaborate Across Teams: Collaboration among marketing, sales, supply chain, and finance teams ensures forecasts are in line with broader business objectives. Regular cross-functional meetings create a sense of shared insight and coordinated effort.
For example, marketing campaigns can be aligned with supply chain readiness to maximize the impact of promotions. This is how forecasts can be transformed into actionable insights that align with business goals.
-
Continuously Monitor and Refine Forecasts: Forecasting demand is not an event but a continuous refinement process. Regular forecast review and feedback integration allow businesses to highlight areas that require improvements.
Using tools that offer automated recalibration ensures forecasts stay up-to-date with changing conditions. Continuous monitoring helps retailers stay attuned to market shifts and customer needs.
These best practices enable retailers to build robust forecasting systems that improve operational efficiency, increase profitability, and provide better customer experiences.
Real-world Retail Demand Forecasting Examples
Understanding the application of retail demand forecasting becomes easier with practical examples that highlight its transformative impact. The following real-world case studies illustrate how businesses leverage forecasting to optimize operations, reduce costs, and improve customer satisfaction.
Walmart’s AI-Powered Forecasting System that Optimizes Holiday Shopping Experience
Walmart has installed state-of-the-art AI and ML technologies to improve inventory management, making holiday shopping at its stores a seamless experience. The AI system analyzes historical sales data, weather patterns, and other external factors to strategically position holiday items in its 4,700 stores, fulfillment centers, and distribution hubs.
It optimizes inventory flow, ensuring that everything from popular gifts to seasonal decorations is always available for customers. This data-driven approach integrates physical and digital sales, enabling Walmart to forecast demand and streamline its omnichannel operations accurately.
Walmart’s AI models go beyond traditional sales data; they incorporate macroeconomic trends, local demographics, and potential disruptions to adjust inventory levels dynamically and match demand fluctuations.
Walmart is able to disregard "outlier" events, such as a one-time snowstorm, ensuring that such anomalies do not affect future inventory planning. The company has started using a patent-pending attribute that improves the system's accuracy in forecasting and optimizing supply chain requirements.
Such AI-driven systems enable Walmart to predict customer preferences based on regional demand and stock levels, ensuring a smooth shopping experience and faster customer deliveries (Source: Walmart).
Improving Demand Forecasting with Machine Learning for a Top 10 Retailer
A leading global retailer faced challenges in demand forecasting, with high Mean Absolute Percent Errors (MAPEs) and forecast accuracy rates falling below 90 percent. Its Financial Planning & Analysis team would use a manual, spreadsheet-based approach, which was inefficient, inconsistent, and lacked real-time insights.
The retailer collaborated with Tredence to develop a solution powered by ML at its core. Using ensemble modeling, Tredence built over 10,000 bespoke ML models for different types of products—continuous, promotional, and seasonal.
This advanced approach replaced the retailer’s archaic time series regression and provided it with more accurate demand predictions.
The solution featured automated anomaly detection to notify planners of demand changes and inventory shortages, as well as causal modeling to pinpoint factors behind forecast deviations, such as weather or promotions. It also continuously optimized seasonal inventory, alerting planners to potential overstock or stockout risks.
Results
- 600 basis point improvement in forecast accuracy
- 50 percent time savings for planners
- 6 percent improvement in seasonal inventory costs
The integration of ML in the demand forecasting process enabled the retailer to achieve high accuracy, improve operational efficiency, and reduce costs, thus driving improved business outcomes (Source: Tredence).
Overcoming Retail Challenges with Tredence’s Advanced Forecasting Solutions
Retail demand forecasting is not just a strategy; it is an important tool in overcoming the complexities of the modern retail landscape. It enables companies to accurately forecast customer demand, optimize inventory, and anticipate market fluctuations.
Partnering with the right analytics service provider is key for retailers looking to enhance their forecasting accuracy and drive better business outcomes.
Tredence leads the way in advanced customer analytics and AI-driven solutions, offering customized services to address the unique challenges of the retail industry.
From advanced demand forecasting models to streamlining data integration and optimizing supply chains, Tredence helps companies stay competitive in the market. It enables you to transform your demand forecasting process into a strategic advantage, ensuring efficient and customer-centric operations.
Ready to revolutionize your retail demand forecasting? Contact Tredence today to take a step toward smarter, data-driven decisions that drive growth and resilience.
FAQs
1. How does seasonality impact demand forecasting in retail?
Seasonality causes fluctuations in demand based on recurring events such as holidays, weather changes, or back-to-school periods. Retailers adjust inventory, pricing, and promotions to align with these predictable demand shifts and avoid stockouts or overstocking.
2. What types of data are used for accurate demand forecasting?
Accurate demand forecasting relies on historical sales data, market trends, customer preferences, and external factors such as economic conditions and competitor activity. Combining structured data (e.g., transaction records) with unstructured data (e.g., social media insights) improves prediction accuracy.
3. What’s the difference between short-term and long-term demand forecasting?
Short-term forecasting focuses on immediate trends, typically covering days to weeks, helping retailers manage inventory and promotions efficiently. Long-term forecasting spans months or years, guiding decisions such as capacity planning, product launches, and supply chain investments.
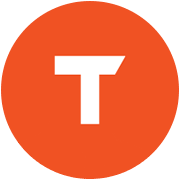
AUTHOR - FOLLOW
Editorial Team
Tredence