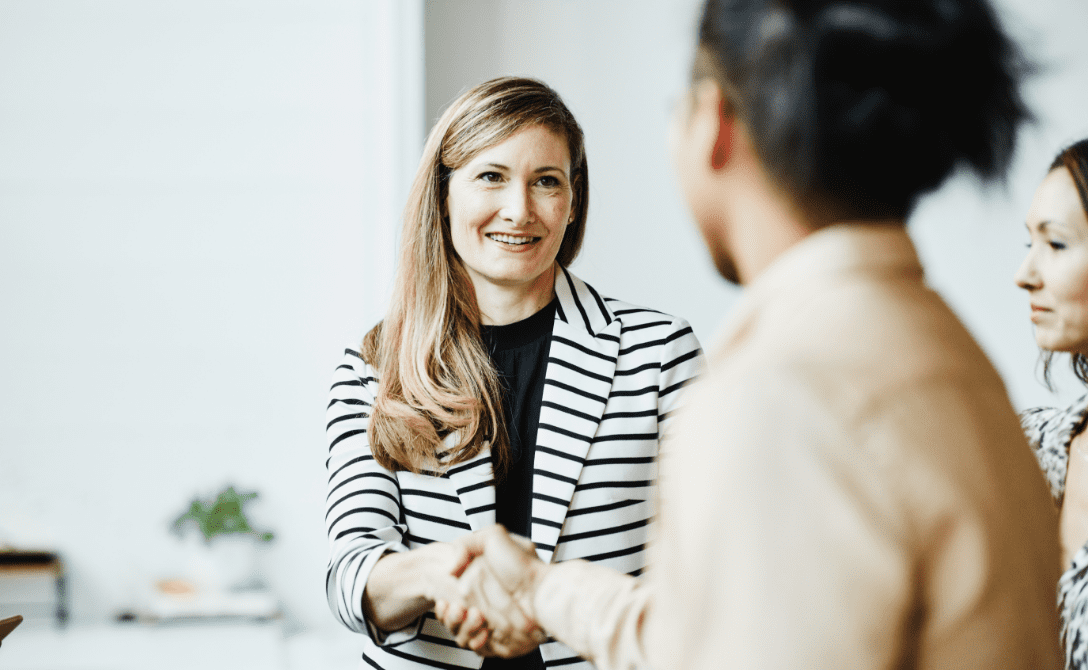
A case study on using Machine Learning in E-Commerce business
The Era of Speed and Customer Satisfaction
Customer satisfaction has become the central point in the E-commerce industry to attract new customers and, most importantly, retain them in the long run. Companies are competing to deliver products as fast as they can in a fast-paced environment. Corporations like Walmart, Amazon, and Home Depot are launching initiatives in this direction like the same day, 1-day, and 2-day delivery promises.
Technical and operational nuances in Supply Chain and Logistics play a crucial role in determining the speed of delivery. Placement of fulfillment centers, strategic utilization of Brick & Mortar stores as shipping centers, Vendor SLAs, effective transportation systems from the first mile to last mile – all contribute to the speed at which a product is delivered.
Traditional Conservative Approaches
Companies are currently struggling to promise the right delivery time due to the ample and mercurial dynamics involved in the supply chain. Traditionally, companies are using hard-coded business rules based on their experience and average SLAs to determine the promise days to be shown on the website. Due to the constant change in businesses, dynamic SLAs, peak sales periods, the accuracy of promise becomes significantly lower and possess two huge problems –
- Very conservative promise time would lead to a loss in customer conversion as higher promise time would not attract customers.
- Very aggressive promise time will lead to bad customer experience if the company fails to deliver within the promised time window.
Companies these days, fearing mostly about the latter point on the delivery experience and mainly concentrating on the ‘On-Time’ metric, are adding additional buffer times across the system. This buffer keeps increasing since every business unit incorporates its own buffer to reach the ‘On-Time’ goal. Research and analysis from top E-commerce companies suggest that the customer conversion is strongly correlated with the delivery promise days, and the website conversion metrics drop exponentially as the promise days increase.
How Machine Learning can help promise accurately?
The applicability and efficacy of Machine Learning, pioneered primarily by the technology giants, is still at a nascent phase across certain business functions like Supply Chain and Operations. Tredence is collaborating with multiple clients in Supply Chain, to bridge this gap and make it a reality. Tredence has worked with an E-commerce leader to predict the promise days accurately while identifying the right trade-off between on-time delivery and a more aggressive delivery promise. In other words, driving a trade-off between better customer experience and better customer conversion.
“The models are as good as the data being trained.”
Historical data has a lot to say about the delivery performance of vendors, FCs, and transportation carriers across different geographies. Key factors that impact delivery time and thereby, the promised time, are – Type of flow path in Supply Chain (Owned FC, Vendor Fulfillment), Service Level Agreement by the Vendor and FC, inconsistency between the SLAs vs. the ground reality, geographic location and distances, product category and SKU types, transportation vendors and truck availability, real-time traffic
With the constant change in business dynamics and seasonal effects, right anomaly detection methods and trend shift models will help identify the right balance between recency and sample availability for model development. The prediction of promise days can become more accurate if the proper techniques and algorithms are used to identify trends across all the touchpoints mentioned above. Traditional Machine Learning algorithms like regression and classification, utilize mean-optimized cost functions. These standard algorithms fail where the cost of over-promising versus under-promising differs significantly. In such cases, error metrics like MAPE, WAPE will not represent the actual efficacy of the model, and custom error metrics and loss functions are required to model the asymmetric costs. These custom models, combined with ensembles like boosting and bagging algorithms, are proved to work best under various business conditions. Finally, regular feedback loops and failing-experimenting-improving mechanisms should be in place to achieve higher accuracies.
Impact of COVID
The COVID-19 pandemic has disrupted supply chains all over the world. Everything, right from the production to the final consumer behavior, has been changing dynamically, posing some extraordinary challenges for Machine Learning. For some of the businesses, it is not exaggerating to state that the historical data has become obsolete. As a Supply Chain modeler, one must be too aware of the changes in consumer behavior, government policies, and the local trends to estimate the impact on their delivery times. At Tredence, we are studying the recent trends and changes in business dynamics to select the right training data and features amidst the chaotic noises. Also, wider prediction intervals and the conservative approaches are put in place in addition to the ML models to achieve better customer satisfaction during these pandemic times.
IT Compliance and steps towards ML-Ops
Data and system-related aspects present certain hurdles while model development. Hence, necessary data lakes must be created and pipelined for building multiple models. The data systems from order management, shipping, and transportation should be integrated for streamlining analytical datasets creation. Data and model refresh cycles must be decided to accommodate real-time changes in vendor agreements, transportation routes, etc. Models go in-vain when they are not compliant with the existing deployment technology. Hence, identifying the bottlenecks during the development phase itself will help the Data Scientists concentrate on viable solutions. MLOps is an emerging practice that helps in collaboration between Data Scientists and IT Operations to help manage production ML. The key architecture includes automation, scaling, governance, and diagnostics, which will smoothen the process of deploying and managing multiple promise models across the organization.
Is your e-commerce business suffering from similar On-Time problems? Tredence can help you predict accurate and reliable promise time using advanced Machine Learning techniques.
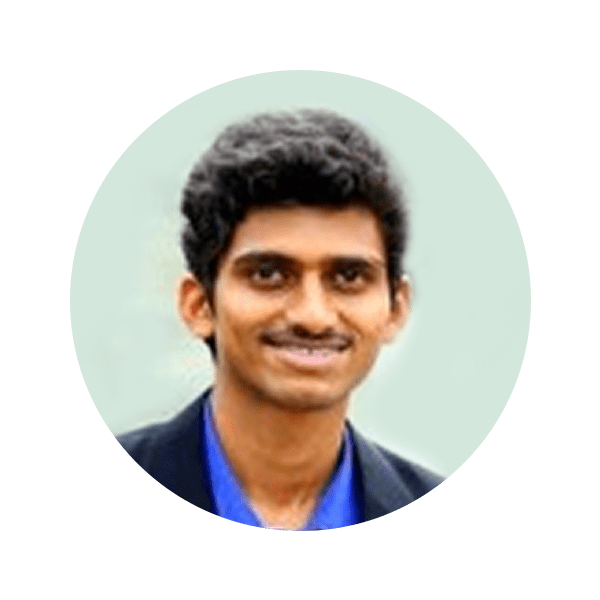
AUTHOR - FOLLOW
Murali Krishna
Analytics Consultant, Tredence
Topic Tags