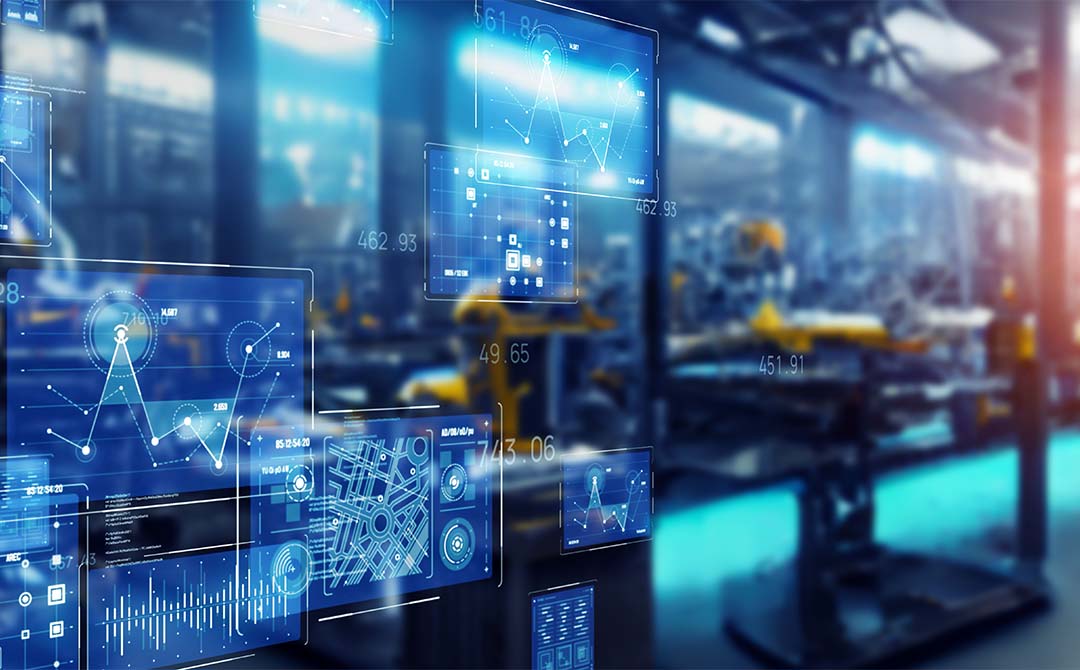
The rise in usage of analytics to resolve complex challenges and realize new opportunities is significantly transforming the manufacturing sector. Companies increasingly use manufacturing analytics to optimize processes, reduce costs, and improve productivity.
With global manufacturing output expected to reach $13.8 trillion in 2025, businesses must quickly adjust their operations to changing market demands and conditions to remain competitive (Source: Statista).
Consider a manufacturer of FMCG products encountering inconsistent demand cycles and unexpected machinery breakdowns.
Without analytical insights into the manufacturing process, the manufacturer cannot assess if production lines meet daily targets. Furthermore, a machine breakdown would abruptly halt or reduce capacity, leading to underproduction. These inefficiencies can adversely impact profitability and brand reputation.
Manufacturing analytics leverages data to uncover insights that enable decision-making, optimize operations, and create value across the manufacturing lifecycle. This article explores key concepts of manufacturing analytics, highlighting its importance and industrial applications in today's manufacturing landscape.
What is Manufacturing Analytics?
Manufacturing analytics refers to the use of data to optimize production, identify inefficiencies, and predict outcomes. By leveraging technologies such as IoT and big data, it enhances decision-making and operational efficiency, thus helping businesses improve productivity and reduce costs with real-time insights.
Analytical tools detect bottlenecks in the manufacturing process, such as packaging delays and inefficiency in developing raw materials. They can also identify demand spikes in specific areas influenced by holiday events and promotional activities.
Manufacturing analytics caters to consumer demand and reduces overproduction and waste while enhancing operational efficiency. The result is a leaner manufacturing process analytics that adapts quickly to market needs, ensuring consistent product availability and maximizing profitability.
With manufacturing analytics, manufacturers can derive insightful decisions that spur innovation and competitive advantage by offering real-time insights and predictive capabilities.
Importance of Manufacturing Analytics
Manufacturing analytics is crucial for an organization to stay competitive in an increasingly competitive market. Here are the key reasons why it is indispensable:
Increase in Productivity
Manufacturers often struggle with unplanned downtime, inefficient workflows, and underutilized resources that hamper productivity. Manually identifying these inefficiencies is time-consuming and prone to errors.
Manufacturing analytics responds to these challenges by presenting real-time insights into production workflows and underperforming production lines. This enables manufacturers to perform predictive rather than reactive machine maintenance.
Predictive maintenance leads to a 20 percent increase in labor productivity, resulting in higher production output and the ability to meet increasing market demands without compromising quality (Source: Deloitte).
Cost Reduction
Balancing operational costs with profitability remains challenging for manufacturers, primarily due to increased raw material prices and energy costs.
Without granular data, it is difficult to pinpoint wasteful practices or inefficiencies in energy consumption. Manufacturing analysis helps track resource usage, labor efficiency, and operational inefficiencies, thus providing opportunities for reducing costs.
Improvement in Quality Control
Ensuring consistent product quality is challenging, as defects can arise from minor deviations in processes or materials.
Traditional quality control methods often detect issues too late, resulting in high rework costs or potential product recalls. Manufacturing analytics uses predictive algorithms to identify possible defects in real time, ensuring consistent product quality.
Supply Chain Optimization
Manufacturing companies face challenges such as inaccurate demand forecasting, mismatched inventories, and delayed supplies, resulting in supply chain inefficiency. These problems disrupt production schedules and increase costs. Manufacturing analytics smoothens the supply chain by facilitating demand forecasting, tracking inventories, and assessing supplier performance.
Sustainability Goals
Lack of transparency in energy usage and emissions makes it challenging for manufacturers to achieve sustainability targets. For instance, manufacturers may be unable to detect improvement areas or monitor their performance regarding environmental goals.
Manufacturing analytics resolves this issue by monitoring energy consumption, carbon footprints, and material efficiency in real time. This proactive approach also ensures regulatory compliance and positions the manufacturer as an industry leader in sustainability.
How Does Manufacturing Analytics Work?
Manufacturing analytics is a structured approach to extracting actionable insights from vast production data. It enables manufacturers to address inefficiencies and enhance operations.
Through key steps, businesses can transform raw data into actionable insights that drive productivity, cost-efficiency, and quality improvements.
Data Collection
Data collection forms the foundation of manufacturing analytics, gathering information from various sources such as IoT sensors, ERP systems, and MES. IoT sensors capture real-time data on machine performance, temperature, energy consumption, and production rates. ERP systems provide information on inventory, workforce, and financials, while MES platforms monitor the execution of manufacturing orders.
Data Integration
Data integration involves aggregating information from various sources into unified repositories such as data lakes, warehouses, or cloud data platforms. These repositories avoid information silos within departments.
AWS Data Lake and Microsoft Azure Synapse help streamline this process by offering scalable storage and seamless integration. Data integration transforms disparate data into a usable format, enabling cross-functional analysis. The result is a unified dataset prepared for analysis, ensuring no crucial information is missed.
Data Analysis
Data analysis refers to the use of advanced techniques to analyze data with statistical models, ML algorithms, and visualization tools to obtain patterns and trends. Platforms such as Tableau, Power BI, and SAS Analytics are used to establish correlations, predict machine failures, and evaluate production efficiency.
Predictive maintenance uses historical and real-time data to forecast equipment failure and reduce unplanned downtime. It transforms raw data into meaningful insights, offering a clear picture of operational performance.
Generation of Insights
This phase presents actionable insights through dashboards, reports, and alerts. These insights highlight areas requiring immediate attention, such as declining machine performance or an upcoming maintenance need.
Tools such as IBM Watson Analytics and SAP Analytics Cloud provide a user-friendly interface for visualizing data and building predictive models. The goal is to ensure that insights are quickly interpretable by decision-makers, enabling proactive action. The output provides recommendations for process optimization, risk mitigation, and resource allocation.
Acting on the Insights
The generated insights are then put into action; the goal is to identify the pain points and optimize the process by implementing predictive analytics, alerts, etc. When the problem is highlighted before it occurs, the mechanism to address it can be scheduled during non-peak hours, which would not require downtime and would not disrupt in-line operations.
Solutions such as PTC ThingWorx and Oracle Analytics Cloud have simplified the implementation of analytics-driven strategies. This has resulted in a more efficient manufacturing process that boosts productivity, reduces costs, and improves quality control.
General Electric's Predix platform leverages IoT data for real-time monitoring and predictive maintenance. GE's analytics solutions save millions annually by avoiding unplanned downtime (Source: cctech).
Manufacturing analytics transforms data into actionable insights, boosting efficiency and cost savings and increasing product quality. With the help of advanced tools and methodologies, manufacturers can proactively overcome most of the challenges and stay ahead in the competitive market. The journey from data collection to actionable strategies highlights the importance of implementing analytics in manufacturing operations.
Goals of Manufacturing Analytics
Manufacturing analytics is important in transforming the manufacturing process, driving efficiency, decision-making, and overall operational effectiveness with data analytics.
Equipped with data-driven insights, organizations can effectively facilitate workflows, improve quality, inspire innovation, and meet regulatory compliance standards. The following are some goals of manufacturing analytics:
Maximizing Efficiency: Streamlining production flow and reducing downtime are key goals for manufacturers. By monitoring real-time data and utilizing predictive analytics, manufacturers can identify production bottlenecks and detect potential machine failures in advance, thus enabling proactive maintenance.
This reduces downtime due to machine failures and helps allocate the right resources per workload on specific sections in manufacturing plants.
Smarter Decision-making: Manufacturing analytics gives managers real-time, data-driven insights, enabling quick and informed decision-making. With continuous data flow from the production floor, decision-makers can track operational performance across departments in real time, identifying inefficiencies and implementing immediate changes.
Smarter decision-making reduces the risks of reactive decisions while ensuring that operational goals align with the business objectives. Predictive capabilities also allow managers to forecast market trends and inventory and production requirements accurately.
Improving Customer Satisfaction: Manufacturing analytics is key to ensuring the timely delivery of high-quality products. It helps track production performance in real time, enabling the early identification of defects, delays, and quality inconsistencies along the production chain.
This proactive approach ensures timely delivery, consistent quality, and fewer product defects, leading to increased customer satisfaction and higher customer loyalty. As a result, businesses experience improved retention rates and build a strong reputation for timely delivering reliable products.
Cutting-edge Innovation: Manufacturing analytics drives innovation by providing insights that create opportunities to explore new product ideas, materials, and production techniques.
Such monitoring and analysis reveal patterns and trends in the data, highlighting areas where changes can be made to a product's design or manufacturing process.
Analytics also allows new production methods to be tested to assess their impact on efficiency, quality, and cost. Additionally, manufacturers can gauge a market's emerging demands and quickly adapt strategies to stay competitive.
This innovative approach enables companies to bring new products to market faster while staying aligned with evolving customer needs and dynamic industry trends.
Regulatory Compliance: Compliance with industry standards and regulations is paramount for manufacturers, with legal challenges and reputation at stake. Manufacturing analytics provides tools to track and report essential metrics related to quality control, environmental impact, and safety regulations.
It helps manufacturers comply with environmental standards through real-time energy consumption, emissions, and waste generation monitoring. Furthermore, data-driven insights enable manufacturers to maintain transparency in processes, providing traceability audits with minimal chances of error.
The ability to stay aligned with regulatory requirements through continuous monitoring helps manufacturers build a strong reputation for being responsible and compliant.
Thus, manufacturing analytics empowers organizations to maintain competitiveness through effective data insights that drive productivity, quality, and customer satisfaction.
Clear goals around efficiency, decision-making, innovation, and compliance enable manufacturers to leverage analytics to enhance operations, mitigate risks, and maintain a leading position in a dynamic market.
Real-life Manufacturing Analytics Use Cases and Examples
Manufacturing analytics is revolutionizing industries by providing actionable insights that drive efficiency, innovation, and cost-effectiveness. Its applications range from enhancing production workflow to ensuring optimized resource utilization.
Industrial Use Cases of Manufacturing Analytics
Key industrial use cases that demonstrate the transformative potential of manufacturing analytics are outlined below:
1. Predictive Maintenance
Unplanned equipment downtime can significantly disrupt operations and inflate costs. Powered by manufacturing analytics, predictive maintenance tools monitor equipment health based on historical and real-time data from sensors and IoT devices.
This helps detect anomalies or impending failures, thus giving businesses time to schedule maintenance activities and avoid disruptions.
2. Process Optimization
Manufacturers strive to achieve operational efficiency with minimum waste. Analytics tools help analyze production data, identify bottlenecks, optimize workflows, and balance machine loads.
For instance, real-time analytics can optimize variables such as temperature and pressure during complex chemical reactions, ensuring the plant operates safely and securely while maximizing output. This significantly increases productivity and minimizes resource wastage.
3. Quality Control and Defect Reduction
Quality assurance is vital in maintaining customer trust and minimizing warranty claims. Manufacturing analytics uses machine learning algorithms to identify patterns and factors that contribute to product defects.
In electronics, analytics tools can trace defects to specific components or processes, enabling root cause analysis and targeted fixes. Addressing these issues in real time would help manufacturers achieve consistent output and reduce rework costs.
4. Energy Efficiency
Energy costs are a major expense in manufacturing. Analytics helps keep track of consumption patterns across machines and facilities for energy use, highlighting losses.
In the steel industry, energy analytics tools monitor furnace usage to optimize power consumption, reduce carbon emissions, and lower operational costs, all while supporting sustainability goals.
5. Supply Chain Optimization
A strong supply chain is essential for on-time production and delivery. Manufacturing analytics equip supply chains with real-time visibility into inventory, supplier performance, and logistics.
In the food and beverages industry, analytics tools predict demand fluctuations to ensure optimal stock levels and minimal spoilage. This increases customer satisfaction while reducing inventory carrying costs.
6. Workforce Productivity
Analytics tools help manufacturers optimize labor allocation by analyzing workforce data, such as shift patterns and task performance, to boost productivity.
In the textile industry, analytics tools track employee efficiency and recommend scheduling adjustments to meet production targets while preventing employee overburden. This ensures a balance between productivity and employee well-being.
7. Custom Production and Mass Personalization
Agility in manufacturing processes is required to meet the rising demand for personalized products. Analytics enables producers to customize production lines according to customer preferences and market trends.
For instance, consumer electronics companies leverage analytics to predict customer preferences for features and design, enabling them to align products with market demand. This improves time-to-market and customer satisfaction.
8. Inventory Management
Optimum inventory levels prevent production delays and overstocking. Manufacturing analytics enables accurate demand forecasting by analyzing past trends, seasonality, and market conditions, helping optimize inventory management.
In the pharmaceutical industry, analytics helps ensure that life-saving drugs are always available but not overproduced, as this may result in losses due to drug expiration.
9. Sustainability and Waste Reduction
Modern manufacturers are under pressure to adopt sustainable production methods. Analytics tools track the generation of waste at each stage of the production process and suggest steps to minimize it.
This could involve identifying excessive material usage and recommending design optimizations within the packaging industry, which would reduce costs and environmental impact.
10. Product Lifecycle Management
Analytics is important in product lifecycle management, from design to disposal. Integrating data across all stages of a product's lifecycle allows manufacturers to make informed decisions on design improvements, material selection, and end-of-life recyclability.
In the aerospace industry, product lifecycle management analytics ensures that aircraft components are designed for maximum durability while meeting regulatory standards.
The use cases above demonstrate how manufacturing analytics helps businesses achieve operational excellence and competitive advantage. By using data-driven insights, manufacturers can proactively resolve challenges, innovate processes, and align with evolving market demands.
Advanced analytics and strategic interventions empower businesses to achieve outstanding outcomes, from cost savings in transportation to improved product quality in manufacturing. This demonstrates the value of leveraging data and analytics to drive operational excellence and sustain growth.
Optimizing Transportation Costs Through Load Consolidation Post-merger
A leading North America-based manufacturer sought Tredence’s expertise to identify synergies in outbound transportation following a merger. The objective was to optimize load consolidation opportunities and reduce costs across priority lanes.
To address this, Tredence broke down the business goals into actionable problem statements, focusing on feasible consolidation modes, identifying high-priority lanes with savings potential, and developing a reusable tool for ongoing cost evaluations.
It analyzed data and devised scenarios to realize load consolidation opportunities. These scenarios were rigorously validated on destination clusters to ensure their feasibility. A prioritization framework was then developed to identify high-saving lanes and quantify the savings potential for each mode of load consolidation.
Tredence created customized roadmaps for each lane for effective implementation, factoring in savings potential, IT systems, and location-specific constraints.
The initiative delivered multiple benefits. Tredence improved load consolidation by introducing scheduled two-day and three-day shipments, significantly enhancing efficiency compared to reactive processes.
For Phase II optimizations, it proposed additional cost-saving strategies, including multi-pick-multi-drop routes and cross-docking. Furthermore, Tredence developed a simulation tool that modeled annual operations, enabling the client to make data-driven decisions and expand into other geographies.
Tredence’s comprehensive 12-month execution plan reduced overall outbound transportation costs by 13 percent, optimizing operations while providing a scalable framework for future growth (Source: Tredence).
Optimizing Product Yield Through Analytics in Manufacturing Process
Nestlé, a global leader in food and beverages, faced challenges regarding the product agglomeration process, leading to inconsistent moisture and density in its powder. This variability resulted in low product yield, wasted materials, and inconsistencies in packaging.
To address these issues, the company implemented real-time data analytics to predict product quality and recommend optimal process setpoints, thus improving consistency and reducing waste.
It used advanced analytics to predict density and moisture levels in the product in real time during the manufacturing process. Nestlé also aimed to provide operators with suggestions for manipulating variables, allowing them to produce the desired powder independently and consistently.
In a trial, Nestlé achieved 10 percent savings in product yield—saving 101 grams of powder per kilogram—as higher consistency led to less powder wastage in product lines. This resulted in a more consistent jar-to-jar weight variation.
Cloud-based analytics also allowed for rapid scaling and easy updates, ensuring continuous improvements. This project's success helped Nestlé enhance efficiency and consistency across its manufacturing operations (Source: Aveva).
Whether it is enhancing production quality, cutting costs, or driving sustainability, the applications of manufacturing analytics are vast and varied across industries. Companies that leverage these tools are better equipped to thrive in today's highly competitive manufacturing landscape.
Challenges in Manufacturing Analytics
Manufacturing analytics helps boost efficiency, reduce costs, and enhance product quality. However, implementing and scaling analytics solutions in manufacturing presents challenges across various areas.
The common challenges include:
1. Data Silos and Integration Complexities
Most manufacturing companies obtain data from various sources, such as IoT devices, ERP systems, MES platforms, and SCADA systems. However, this information tends to remain fragmented among business units.
Such a condition makes integration one of the critical challenges. This dispersed data can be unified to allow for cross-functional analysis. Consolidating these various data formats and converting them into something usable requires heavy lifting.
2. Data Quality Issues
Analytics insights are only as effective as the quality of input data. Inconsistent formats, missing values, duplicate records, and incorrect sensor readings lead to imperfect analytics and poor decisions.
Ensuring the quality and consistency of data from multiple systems is an ongoing challenge that requires robust governance frameworks and continuous monitoring.
3. Limited Technical Expertise
Manufacturing operations are becoming increasingly digitized; however, many organizations still face skill-based gaps in data analytics and data science.
The inability to implement advanced techniques such as predictive analytics, machine learning, and real-time monitoring due to a lack of in-house expertise may prove to be a serious disadvantage. Recruiting and retaining skilled professionals or collaborating with external partners is essential but costly.
4. Resistance to Change
Employees, particularly in traditional manufacturing environments, often resist new technology due to a lack of familiarity with its use or a fear that it may replace their jobs. Overcoming resistance requires creating trust in the analytics tools and proper training to help them understand the benefits.
5. High Implementation Costs
Implementing analytics technologies in manufacturing requires significant investment in infrastructure, software tools, and human resources from the beginning.
These costs can exceed small and medium-sized manufacturers' budgets, slowing the adoption of analytics technologies. Additionally, companies must balance the immediate expenses with the long-term returns on investment, which can further complicate decision-making.
6. Cybersecurity Risks
The further integration of IoT devices and cloud platforms in manufacturing analytics introduces increased risks of cyber threats. Additionally, securing sensitive production data and preventing unauthorized access to critical systems adds complexity to implementing analytics solutions.
Overcoming these challenges requires well-defined and planned actions: strong leadership commitment, investment in technology and skills, and fostering a habit of fact-based decision-making. Removing these barriers will allow manufacturers to unleash the full potential of analytics in changing their operations.
Best Practices for Manufacturing Analytics
Industry best practices for manufacturing analytics focus on optimizing processes to enhance effectiveness and efficiency, ensuring that analytics initiatives align with the organization’s broader business objectives to drive maximum value.
Here are some key best practices related to manufacturing analytics:
Start Small: Begin with pilot projects to test the viability of analytics initiatives. This provides manufacturers with an opportunity to experiment on a small scale.
Pilot projects bring up potential challenges, test how effective an analytics approach may be, and allow one to change strategies where necessary. This approach helps organizations minimize risks and gain insights from real-world data before making large-scale commitments. As a result, it ensures smoother transitions and more favorable outcomes in the long term.
Focus on ROI: Prioritize use cases that provide higher expected returns on investment. Analyze high-priority areas or specific production processes to pinpoint where insights can drive significant improvements.
Focusing on high-priority use cases delivers early wins that showcase the value of analytics, building confidence for further investment. This, in turn, drives greater financial benefits and enhances operational efficiency.
Leverage Partnerships: Collaborate with analytics experts to accelerate the implementation process and achieve the best results. Analytics experts are equipped with specialized knowledge, tools, and resources that accelerate the adoption of advanced techniques.
Partnering with established analytics providers or consultants can help manufacturing companies avoid common pitfalls and accelerate the process of unlocking the full potential of their data. This ensures that analytics is integrated faster and more efficiently.
Ensure Data Accuracy: Provide appropriate data governance policies to ensure the accuracy and reliability of collected data. Strong governance, such as regular data audits, validation checks, and standardized processes, ensures high-quality data.
Reliable data forms the foundation for informed decision-making, and meaningful analytics can only be derived from accurate data. Manufacturers can trust the insights generated by ensuring data accuracy, leading to better decision-making and improved operational outcomes.
Embrace Agility in Best Practices: Regularly revising and updating analytics strategies is essential to address the evolving needs of both the business and the market. In an agile approach, manufacturers can swiftly change analytics processes, establish new priorities, and sharpen existing strategies with real-world insights.
This approach ensures the relevance and impact of analytics efforts. Agility allows organizations to stay aligned with industry trends, improve performance, and make quicker, data-driven decisions that reflect shifting priorities.
By following these best practices, manufacturers can ensure their analytics initiatives are cost-effective and scalable. Implementing these practices results in more efficient processes, greater ROI, and a data-driven culture that drives continuous improvement and innovation.
Optimizing Manufacturing Analytics with Tredence’s AI-enabled Solutions
Manufacturing analytics helps optimize operations, enhance efficiency, and reduce costs. Through data-driven insights, manufacturers can streamline production, minimize downtime, and improve overall productivity.
Successful implementation of manufacturing analytics goes beyond adopting the latest technologies such as AI, ML, and IoT; it also involves fostering a culture of continuous improvement and ensuring strategic alignment across the organization. These tools drive innovation and operational excellence by enabling predictive maintenance, supply chain optimization, and advanced process control.
Real-time data insights help manufacturers make informed decisions, predict challenges, and adapt to market trends. Cloud computing advances have made it possible even for small-scale enterprises to find scalable and affordable analytics solutions.
As manufacturing becomes increasingly data-driven, investment in analytics technologies and talent is no longer a choice but an absolute necessity to stay competitive in this fast-moving environment. However, manufacturers need advanced tools and specialized expertise to effectively apply analytics across their processes and fully harness the potential of these technologies.
Tredence, one of the leading data analytics and AI-driven solution providers, is leading the charge in enabling manufacturers to achieve these transformative goals. With deep expertise in advanced analytics, AI, and data engineering, Tredence provides tailored solutions that empower manufacturers to unlock the full potential of their data.
Tredence’s solutions include predictive maintenance, production optimization, and supply chain analytics. Its proven methodologies enable manufacturing enterprises to unlock innovation-driven, long-term growth while enhancing operational efficiency.
As manufacturing companies continue to adopt analytics, the need for a partner who understands the industry's intricacies is paramount. Tredence's expertise ensures manufacturers can seamlessly integrate analytics into their existing processes.
Get in touch with Tredence today to discover how its solutions can transform your manufacturing operations.
FAQs
What is the role of predictive analytics in manufacturing?
Predictive analytics helps manufacturers optimize production, forecast demand, reduce downtime, and improve quality by using data-driven insights to anticipate issues before they occur.
How is manufacturing analytics different from traditional data analysis?
Manufacturing analytics focuses on real-time data from IoT sensors and machines to drive operational efficiency, while traditional data analysis often deals with static datasets and broader business trends.
What is the future of manufacturing analytics?
The future lies in AI-driven analytics, real-time monitoring, autonomous decision-making, and enhanced supply chain optimization, leading to smarter, more agile manufacturing processes.
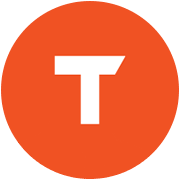
AUTHOR - FOLLOW
Editorial Team
Tredence