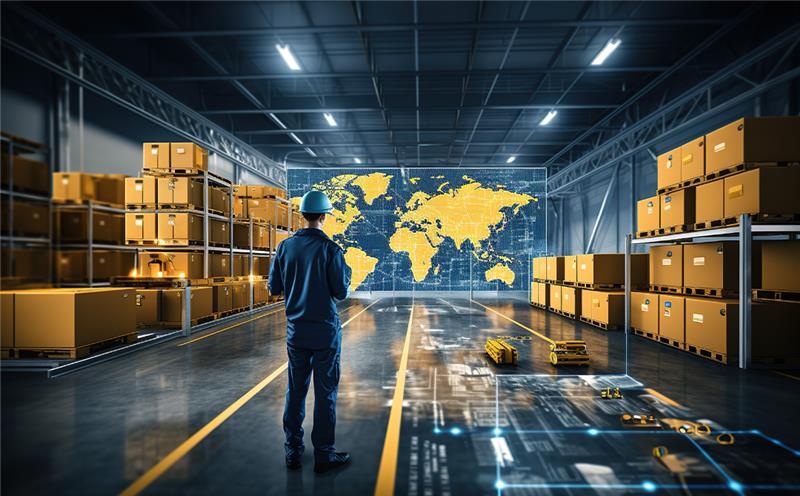
Recent disruptions—manufacturing delays, global conflicts, and extreme weather—have strained supply chains. With rising customer demands for quality and quick delivery, businesses face mounting pressure to enhance efficiency and maintain satisfaction.
According to Tom Bartman, Associate Partner, McKinsey, “Labor shortages, limited equipment availability, and the ripple effect of global bottlenecks are three significant challenges facing global supply chains.” (Source: McKinsey & Company)
Enter predictive analytics—an essential tool for supply chain resilience. By forecasting trends and potential disruptions, predictive analytics enables businesses to streamline operations and stay ahead of challenges, ensuring smoother, more efficient supply chains.
In this article, we’ll explore how predictive analytics transforms supply chain management, highlighting its key benefits, challenges, and real-world applications.
What is Supply Chain Predictive Analytics?
Supply chain predictive analytics combines machine learning and statistical algorithms to analyze historical data and market trends, forecasting future outcomes. By aggregating data from various sources—such as sales figures, inventory levels, purchasing patterns, and transportation metrics—it provides actionable insights that drive accurate decision-making and optimize business processes.
This approach enables businesses to adopt a proactive, data-driven strategy for supply chain management. Predictive analytics not only forecasts demand and identifies potential risks but also uncovers opportunities to enhance efficiency. For example, it helps determine optimal inventory levels, allocate resources effectively, and meet customer demand, ultimately reducing risks and boosting operational performance.
By leveraging predictive analytics, organizations can transform their supply chain operations, making them more responsive and resilient in an increasingly dynamic market landscape.
Role of Predictive Analytics in Supply Chain Management
Predictive analytics plays a key role in optimizing supply chain operations. By predicting potential disruptions and customer demand fluctuations, predictive analytics empowers businesses to stay ahead of challenges and ensure smooth operations.
Key techniques such as data mining, time series forecasting, regression analysis, and optimization algorithms allow for a comprehensive evaluation of the entire supply chain, from procurement to delivery.
In the logistics industry, predictive analytics optimizes delivery routes by factoring in real-time traffic, weather, and road conditions, thus improving delivery speed and reliability. Integration with IoT devices further enhances this by providing real-time shipment monitoring, giving businesses greater control over logistics.
A recent study by Kearney, commissioned by the Council of Supply Chain Management Professionals, found that 77 percent of logistics partners invest in predictive analytics to boost profitability (Source: The 2024 State of Logistics Report).
Benefits of Predictive Analytics in Supply Chain Management
Predictive analytics in supply chain management offers diverse benefits, streamlining supply chain operations. Organizations gain improved visibility, enhance decision-making, reduce costs associated with stockouts or overstock, and boost customer satisfaction by delivering the right product at the right time.
Supply chain predictive analytics also provides numerous strategic advantages. Here are some key benefits of implementing predictive analytics in supply chain management:
Better visibility
Supply chain predictive analytics delivers actionable insights across business operations, enhancing visibility from procurement through last-mile delivery. Such comprehensive oversight enables organizations to prevent delays, identify new business opportunities, and ensure seamless operational efficiency.
Improved decision-making
Supply chain predictive analytics enables organizations to generate reliable forecasts, facilitating data-driven and precise decision-making across key areas such as inventory management, logistics, and resource allocation. Enhanced decision-making capabilities ultimately contribute to improved financial performance and sustainable business growth.
Cost savings
Businesses can maintain optimal inventory levels with accurate insights into customer behavior and market trends, thus reducing stockouts, overstocking, and costs such as lost sales.
Supply chain predictive analytics facilitates accurate demand forecasting and data-driven analysis, enabling organizations to meet customer needs while achieving significant cost savings effectively.
Enhanced customer satisfaction
Predictive analytics in supply chain management enables businesses to deliver the right products to the right customers at the right time, significantly enhancing customer satisfaction.
Organizations can effectively meet customer demands and ensure timely deliveries by gaining insights into customer behavior, maintaining optimal inventory levels, improving logistics efficiency, and increasing supply chain visibility.
Challenges in Implementing Predictive Analytics in Supply Chain Management
While predictive analytics offers significant advantages for supply chain management, several challenges can hinder its successful implementation. These include poor data quality, a shortage of skilled resources, data privacy concerns, and difficulties integrating with legacy systems.
Limited availability and poor data quality
Effective predictive analytics relies on vast amounts of data from various sources to make accurate forecasts. However, many organizations face limitations in data availability, leading to incomplete or inaccurate models. More accurate or good-quality data is needed to ensure the reliability of predictions, making it essential for companies to invest in robust data collection and cleaning processes.
Lack of skilled resources
The complexity of predictive analytics often requires specialized expertise. Many organizations lack predictive analysts or data scientists who can extract actionable insights from the data. This skill gap calls for investment in upskilling initiatives to equip existing employees with the necessary skills or for hiring external talent to drive predictive analytics adoption.
Data privacy and security concerns
The data required for predictive analytics in supply chain management often includes sensitive business and customer information, making it vulnerable to security threats. To mitigate risks, companies must ensure compliance with data privacy regulations and implement robust security measures, including encryption and advanced protective systems, to safeguard this valuable data.
Integration with legacy systems
Integrating predictive analytics with existing legacy systems can be a significant hurdle. The complexity of modern predictive algorithms requires consolidating data from across the supply chain into a unified platform. Often fragmented and outdated, legacy systems may not support such seamless integration, requiring extensive system upgrades or replacements.
Case Study: Tredence’s Solution
Tredence’s supply chain solution helped one of the world’s leading water, sanitation, and infection prevention providers modernize its supply chain platform. By migrating from legacy systems to a modern solution, the company saved approximately $50,000 per month, demonstrating how overcoming these challenges can lead to significant cost savings and improved efficiency. Case study- Migrating Legacy Applications to a Modern Supply Chain Platform.
Key Tools and Technologies in Supply Chain Predictive Analytics
Predictive analytics helps identify patterns and utilizes advanced technologies such as machine learning, data mining, statistical methods, and predictive modeling to provide actionable insights. It aims to identify patterns in the historical data and anticipate future outcomes.
Machine Learning: A subset of artificial intelligence, machine learning enables systems to learn from data and make predictions without explicit programming. It continuously adapts to new information, improving predictive accuracy over time.
Data mining: Data mining is one of the key technologies in supply chain predictive analytics. It uncovers hidden patterns by analyzing vast amounts of data.
Predictive modeling: Predictive modeling deploys statistical techniques and takes a mathematical approach to predict future outcomes. It often works with machine learning to respond dynamically to new data inputs, enhancing decision-making capabilities.
Statistical techniques: Regression, decision trees, and neural networks are the most common statistical techniques used in predictive analytics. Beyond forecasting, these techniques identify areas for improvement, assess risks, and reveal growth opportunities.
These tools and technologies analyze structured and unstructured data to drive intelligent decision-making and enhance business operations.
Use Cases of Predictive Analytics in Supply Chain Management
Predictive analytics in supply chain management offers powerful use cases, ranging from demand forecasting and inventory planning to predictive maintenance and risk management. Predictive analytics enhances supply chain efficiency at every stage by reducing costs and optimizing workflows.
Here are key use cases and their associated benefits:
Demand forecasting
Predictive analytics enables businesses to anticipate and accurately forecast customer demand by analyzing historical trends, customer preferences, and external factors. This insight helps businesses plan with precision, allocate resources efficiently, and minimize waste and costs.
Inventory management and production planning
With precise demand forecasting, predictive analytics helps maintain optimal inventory levels, reducing the risk of stockouts or overstocking. This minimizes waste and lowers carrying costs while ensuring businesses meet customer demand without surplus inventory.
Predictive maintenance
Predictive analytics analyze production logs and equipment sensor data to identify potential bottlenecks and anticipate costly breakdowns. This proactive approach to maintenance ensures smoother operations, reduces downtime, and boosts overall manufacturing efficiency.
Supply chain risk management
Predictive analytics can foresee risks and improve supplier performance management by providing insights into potential disruptions. By acting on these predictions, businesses can mitigate risks before they escalate, ensuring a more resilient supply chain.
Case Study: Walmart
Walmart is an excellent example of leveraging predictive analytics in its supply chain. The company uses AI and ML models to analyze data from sales, online searches, and page views to identify inefficiencies and proactively address supply chain issues.
By harnessing these predictive analytics use cases, businesses can strengthen their supply chain management and drive greater customer satisfaction.
Best Practices for Effective Implementation of Supply Chain Predictive Analytics
Implementing supply chain predictive analytics requires a strategic and scalable approach. Best practices for effective implementation include setting clear objectives, building a robust team, and choosing the right platform.
Organizations should adopt a planned and scalable approach while implementing supply chain predictive analytics. Here are some best practices for effective implementation of supply chain predictive analytics:
Set clear objectives
Evaluate your scope of work and establish clear goals for using supply chain predictive analytics. Analyze existing systems, data sources, and infrastructure to avoid disruptions when integrating predictive analytics with the current systems.
Build a robust team
A team with expertise in predictive analytics for the supply chain is crucial for successful implementation. This team usually includes data scientists and analysts who monitor performance and ensure smooth operations during deployment.
Adopt the right tools and platform
Choosing an advanced, feature-rich platform is critical for staying competitive. A robust platform simplifies the predictive analytics process, enabling more accurate insights and driving desired outcomes.
Future Trends in Supply Chain Predictive Analytics
As supply chains continue to evolve, future trends in predictive analytics will be shaped by the increasing adoption of emerging technologies like blockchain and the Internet of Things (IoT), which will play a crucial role in managing supply chain disruptions.
Blockchain for Transparency and Traceability
Blockchain technology offers enhanced transparency and traceability, providing deeper insights into the supply chain and strengthening data integrity across the entire value chain. By ensuring that data is tamper-proof and accessible to all stakeholders, blockchain can improve decision-making, reduce fraud, and increase trust between partners.
Advancements in AI and Machine Learning
Artificial intelligence (AI) and machine learning (ML) are advancing rapidly, further improving the predictive accuracy of analytics. These technologies drive automation across the supply chain, helping organizations forecast demand better, identify risks, and optimize processes in real time. As AI and ML models become more sophisticated, businesses will gain even greater agility to respond to disruptions and enhance operational efficiency.
Together, these technologies set the stage for a more resilient, transparent, and efficient supply chain, allowing organizations to proactively manage disruptions and stay competitive in an increasingly dynamic market.
Conclusion
Predictive analytics in supply chain management is a vital tool for organizations looking to navigate the complexities of modern supply chain dynamics. Businesses can unlock significant cost savings and boost efficiency by enhancing visibility, optimizing resource allocation, and streamlining operations.
To elevate your value chain, Tredence’s advanced supply chain analytics solutions provide a comprehensive, AI-enabled view of your operations, delivering actionable insights that reduce costs and maximize performance.
Partner with Tredence’s experts today to build intelligent, resilient, and agile supply chain operations that keep pace with an ever-changing market.
FAQs
- How predictive analytics can predict supply chain success?
Predictive analytics analyzes data from various sources, such as historical data, customer buying behavior, and market trends, to identify potential issues and address them proactively, thus optimizing supply chain operations. - What are the major aspects of a supply chain that could use predictive analytics?
Key aspects of a supply chain that could use predictive analytics include demand forecasting, inventory optimization, route planning, predictive maintenance, and last-mile delivery. - What are the key tools and technologies used in supply chain predictive analytics?
Predictive analytics in supply chains leverage advanced techniques such as machine learning, regression analysis, and time series forecasting to predict events.
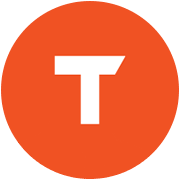
AUTHOR - FOLLOW
Editorial Team
Tredence