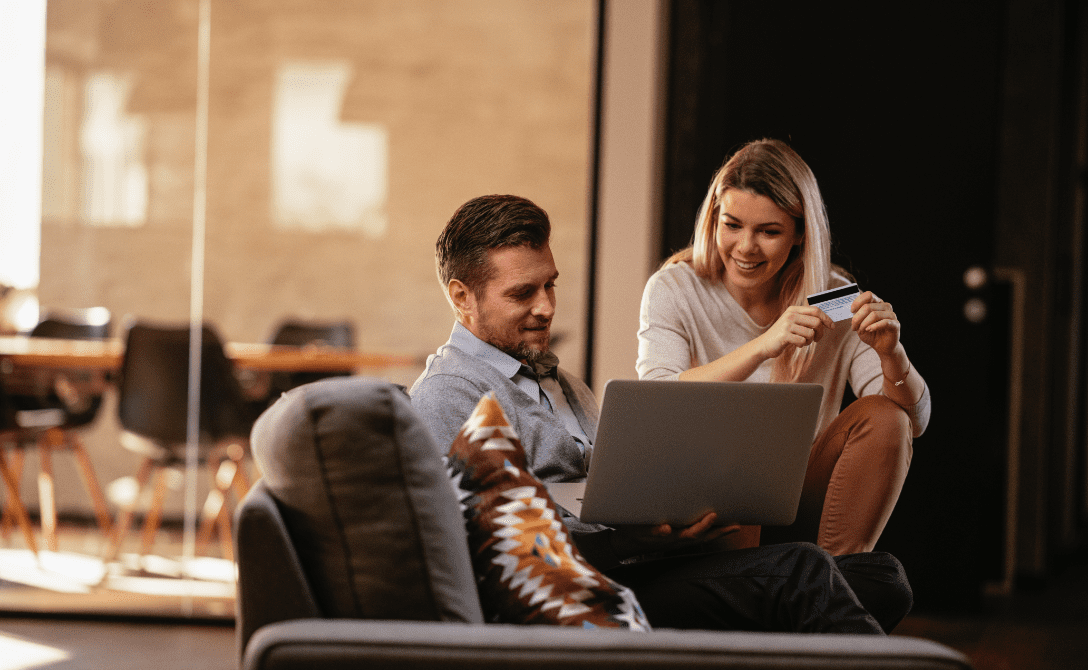
‘Efficiency’ roots from processes, solutions, and people. It is one of the main driving forces leading to significant changes in the way companies work in the first decade of the 21st century. The following decennary further accelerated this dynamic. Now, post-COVID, it is vital for us to become efficient, productive, and environmentally friendly.
One of our clients manufactures and sells precast concrete solutions that improve their customers’ building efficiency, reduce costs, increase productivity on construction sites, and reduce carbon footprints. They provide higher quality, consistency, and reliability while maintaining excellent mechanical properties to meet customers’ most stringent requirements. The customers rely on their quality service and punctual delivery to receive products. This is possible because their supply chain model is simple. They prepare the order by date, call the driver the day before, and load the concrete the next morning. The driver delivers the exact specific product to the specified address.
However, a large percentage of customers cancel orders. One of the main reasons for the cancellation is the weather.
The client turned to Tredence to provide an analytical solution for flagging such orders so that their employees do not have to prepare for such deliveries.
I’ll abridge the journey so far that it led to creating a promising solution.
How it all started?
One of the business units of the client suffered huge operational losses due to the cancellation of orders. Although the causes were(are) beyond their control, they always had(have) to compensate truck drivers and concrete workers. To improve the demand and supply planning process’s efficiency, they had to encounter order cancellation risks. Though they might have increased their resource capacity by adding more people or working in shifts, this option may not have paved well in the long run. Apart from this, the risks may not have been mitigated as anticipated, which might have further reduced the RoI.
Although they put forward various innovative ideas, the results did not reflect the expectations, resulting in the loss of thousands of drivers’ hours. Before deciding to use an analytical solution, they discovered that their existing system has two main shortcomings.
- Extensive reliance on conventional methods for dispatch
- Absence of a data-driven approach
Thus, they wanted to leverage a powerful ML-enabled solution to empower ‘order dispatching’ to effectively get ahead of order cancellation and minimize high labor costs.
A roadmap that led to the solution’s development
The analytics team from Tredence pitched the idea of developing a pilot solution and executing it in the decided test market and then creating a full-blown working solution.
We used retrospective data in the sterile concept (the idea was to solve as many challenges as possible for POC (Proof of Concept)). Later, when the field team gave positive feedback, we planned to deploy a cloud-based working model with a real-time front-end. Next, measure its benefits in terms of hours saved in the next 12 to 24 months.
Proof of Concept (POC)
To reap the maximum benefits and minimize risks on the analytical initiative, we opted to start with the proof of concept (POC) and execute a lightweight version of the ML tool. We developed a predictive model to flag orders at risk of cancellation and simulated operational savings based on the weather and previous years’ data. We found that:
- 50% of orders were canceled each year
- A staggering percentage of orders were canceled after a specific time the day before the scheduled delivery – ‘Last-minute cancellations.’
- Because of these last-minute cancellations, hundreds of thousands of driving hours were lost
Creating the Most Viable Product (MVP)
Before we could go any further or zero down to the solution deployment, we had to understand the cancellation’s levers. And once the POC was ready, we decided to evaluate the results based on the baselines and expectations and compare them with the original goals. Next, we decided to proceed with the pilot test and modify the solution based on its result. Therefore, we selected a location and deployed some field representatives to provide real-time feedback and relied on our research for this purpose. The results (savings potential) were as follows:
- Fewer large orders canceled
- More orders canceled on Monday
- When the temperature dropped to certain degrees, the number of cancellations increased
- More than half of the last-minute cancellations were from the same customers
- If a certain proportion of the orders were canceled at least one day in advance, the remaining orders were canceled at the last minute
- On days with rain, the number of cancellations increased
Overall, order quantity, project, and customer behavior were the essential variables.
The MVP stage provided a staggering number, representing the associated monetary loss (in millions) due to the last-minute cancellations. The reasons behind such a grim figure were the lack of a data-oriented approach and prioritization method.
The deployed MVP helped reduce the idle hours. It helped flag the cancellations that we usually would have missed with our heuristic model. It also provided the market-wise potential, which we ultimately decided to roll out.
Significant findings (and refinements) in the ML model based on pilot test
Labor planning is a holistic process
An effective labor plan must deliberate factors other than the quantity (orders), such as the distribution of orders throughout the day, the value of the relationship with customers, and so on.
Therefore, the model output was modified to predict the quantity based on the hourly forecast.
Order quantity may vary with resource plan
‘Order quantity’ shows a considerable variation between the forward order book and the tickets, making it impossible to use it as a predictor variable.
Resources are reasonably fixed during the day
This contradicts one of the POC’s assumptions that resources will be concentrated in the market on a given day. This has led to corresponding changes in forecast reports, accuracy calculations, etc.
Building and Deploying a Full-blown ML-model at Scale
At this stage, we had the cancelation metrics, levers that worked, and exact variables to use in the solution. Now, the team has enough data to build an end-to-end solution comprising intuitive UI screens & functions, automated data flows, and model runs. And finally, measure the impact in monetary equivalent.
Benefits’ (Impact) Measurement
To turn the wheel and get it on track, we have to extract the model’s maximum value and evaluate it over time. We decided on two evaluation time metrics for measuring the impact.
- Year-on-Year
- Month-on-Month
The following is a summary table of improvements to key operational KPIs. Based on TPD change, the estimated savings are calculated based on the annual business volume.
TPD | Location-specific | US | |
---|---|---|---|
Metric value (YoY) | 30% (up) | >$350k | >$3M |
Metric value (MoM) | 12% (up) | >$150k | >$3M |
*data is speculative and based on the pilot run.
Predictive Model’s Key Features
- Visual Insights
- Weekly Model Refresh
- Modular Architecture for seamless maintenance
Results
- Reduced Deadheading
- Streamlined dispatch planning
- Higher Labor Utilization
- Greater Revenue Capture
Why should you consider Tredence’s ML/AI solutions?
We have successfully tested the pilot solution, and the model has shown annual savings of more than $3 million. Now, we will build and deploy the full version of the model.
Tredence is one of the top analytics and data engineering companies in the US and APAC regions. If you need to make multi-faceted changes in your business operations, let us understand your top-of-mind concerns and help you with our unique analytics services. Reach out to us at info@tredence.com
Topic Tags