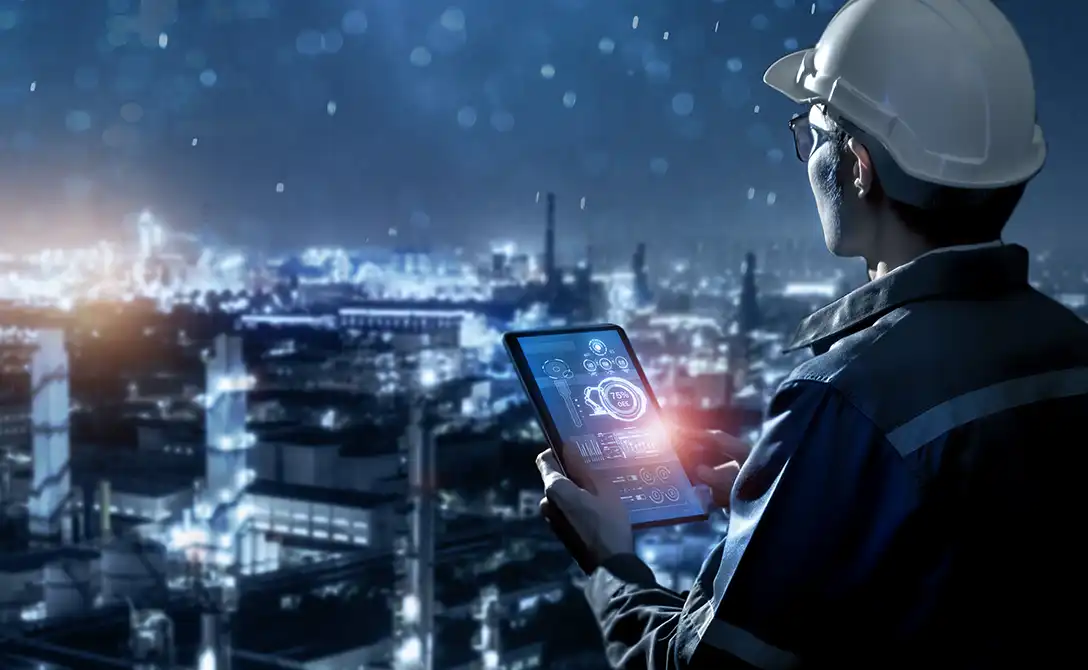
The business world is rapidly shifting towards sustainability as a fundamental requirement, not just a desirable trait. The US Securities and Exchange Commission has mandated that all publicly traded companies disclose their sustainability performance, putting pressure on companies to not only be committed to sustainability but also provide transparent and reliable data on their environmental, social, and economic impact.
This is particularly important for investors, as sustainable investing is becoming more mainstream, with two-thirds of institutional investors, as per a recent study by Morgan Stanley, using ESG data in their investment decision-making process. Studies have shown that companies with strong ESG performance have a greater likelihood of long-term growth and are less likely to face financial risks. Furthermore, according to a report by PwC, many global CEOs believe that their organizations will not be economically viable in the next decade without effective sustainability measurement and reporting.
Therefore, as an ESG leader, if you can effectively manage and communicate your ESG data, you will have a competitive edge in attracting and retaining customers, investors, and top talent.
However, this can be challenging as you may not have the systems or processes to effectively measure and report on their sustainability KPIs. Additionally, reporting practices may lack standardization, making it difficult for your organization to compare and assess sustainability performance. To address these challenges, you must invest in the necessary systems and processes to effectively measure and report on your company’s sustainability performance and work towards standardization in reporting practices.
How to do it?
Leveraging AI and data analytics to address the sustainability data challenge
AI and data analytics-driven sustainability solutions are becoming more critical in addressing organizations' ESG data challenges. Such solutions/tools can assist you in effectively collecting, analyzing, and understanding vast and complex data sets, which are vital for recognizing patterns, trends, and areas for improvement. For example, advanced sustainability analytics and machine learning algorithms can monitor energy consumption, detect energy waste, and forecast energy demand, enabling your organization to reduce energy usage and costs.
Additionally, AI-based systems can automate data collection, analysis, and reporting, reducing the need for human intervention and increasing the accuracy and efficiency of data processing. Furthermore, AI can be used to optimize decision-making. For example, it can assist you in finding the most sustainable solutions in terms of material sourcing, production, and logistics. It can also monitor and predict the impact of different actions on the environment and society.
Reports by McKinsey and PWC suggest that by 2030, AI could contribute $13 trillion to the global economy, with the greatest potential for impact in sectors such as manufacturing, healthcare, and energy. Additionally, AI-driven sustainability solutions could reduce greenhouse gas emissions by 4% by 2030 and save $2 trillion in energy and resource costs.
As we progress, AI and data analytics will play a vital role in addressing the sustainability data challenge, enabling organizations to efficiently collect, analyze, and make sense of large and complex data sets, optimize decision-making, and drive sustainable development.
Before delving deeper into how AI and advanced sustainability solutions can do this, let’s understand the types of sustainability data.
Exploring the various options for collecting and analyzing sustainability data in an organization
Today, organizations have various options to collect and analyze sustainability data. These include:
Current challenges in collecting and analyzing sustainability data
As organizations strive to understand their sustainability performance, it's crucial to consider all types of data holistically and supplement internal data collection efforts with external data sources such as government data and industry benchmarks. However, despite the increasing importance of sustainability data, many companies struggle to effectively measure and report on their performance.
A report by Deloitte found that despite the availability of several reporting standards, methodologies, and management systems, only 3% of CPGs say they produce sustainability data that is as accurate and verifiable as their financial data.
As an ESG leader, you may have faced hurdles in pursuing better sustainability data management. One major challenge you may have encountered is the lack of standardization in data collection and reporting methods, making it difficult to compare data across organizations and industries and identify best practices. Additionally, you may have found the complexity and diversity of sustainability data difficult to integrate and analyze meaningfully. Furthermore, you may have witnessed that your organization lacks the resources to properly address data quality and accuracy.
Therefore, making sustainability data transparent and accessible to stakeholders, including customers, investors, and regulatory bodies, is essential to ensuring accountability but can be challenging.
Identifying the right AI and data analytics solutions for your organization
Effective sustainability data management and reporting begin with thoroughly assessing your organization's current data collection and analysis processes. By identifying where AI and data analytics can improve efficiency and accuracy, you can ensure that you select the right solutions for your specific needs.
When selecting sustainability solutions, it's important to consider your company's technical capabilities and resources. For example, you should choose solutions that seamlessly integrate with your existing systems and be implemented within your organization's current technological capabilities. Additionally, it's important to select scalable solutions that can adapt and grow with your organization's evolving data collection and analysis needs.
Cost-effectiveness is also a key consideration when evaluating sustainability solutions. Look for solutions that can provide measurable returns on investment in increased efficiency, cost savings, and improved sustainability performance.
Lastly, it's essential to consider the ethical implications of using AI and data analytics for sustainability data collection and analysis. You should choose transparent, explainable, and sustainability solutions that align with your organization's values and commitment to sustainability. By taking a thoughtful and strategic approach to selecting AI and data analytics solutions, you can gain valuable insights and drive meaningful action on sustainability.
You can turn sustainability data into actionable insights that drive positive change and business success with the right AI and data analytics ESG partner.
Overcoming common implementation challenges in sustainability solutions
Once you have identified the right sustainability solution, you should overcome the implementation challenges using AI and data analytics.
One common challenge is a need for buy-in from stakeholders, mainly if they are unfamiliar with the benefits of AI and data analytics. It's essential to communicate the new sustainability solutions' help and involve stakeholders in the implementation process to gain support.
Another common challenge is a need for more data quality and accuracy. To overcome this, you can implement data validation and quality control processes to ensure the data is accurate and reliable. Additionally, you need to ensure that the data is properly formatted and structured.
A third common challenge is more resources and expertise to implement and maintain the new solutions. For example, you should consider partnering with the right sustainability analytics solution provider to ensure that your sustainability solutions are implemented correctly, and your organization has the necessary support and guidance to maintain them.
Lastly, you may face challenges in integrating the new ESG solution with existing systems and processes. So, having a clear testing and integration plan before deployment is essential to ensure they work seamlessly with existing systems.
Best practices for successfully implementing sustainability solutions
There are several best practices that you can follow to ensure a successful implementation of sustainability solutions for data collection and analysis:
- Start with a clear understanding of your organization’s sustainability data needs and goals, and select solutions tailored to meet those needs
- Involve stakeholders in the implementation process to gain buy-in and ensure that the solutions align with their needs and expectations
- Ensure that data quality and accuracy are a top priority, and implement data validation and quality control processes to ensure that the data is reliable
- Partner with an advanced ESG analytics expert to ensure that the solutions are implemented correctly and that your organization has the necessary support and guidance to maintain them
- Have a clear plan for integration with existing systems and processes, and test the solutions thoroughly before deployment to ensure that they work seamlessly with existing systems
- Continuously monitor and evaluate the performance of the sustainability solutions and make adjustments as needed to ensure that they continue to meet your organization's needs and goals
- Communicate the benefits and progress of the implementation to all stakeholders to ensure transparency and accountability
By following these best practices, organizations can increase the chances of a successful implementation and make the most of the benefits that AI and data analytics can bring.
The future of sustainability data management with AI and data analytics
In the future, AI and data analytics are expected to play an increasingly important role in sustainability data management. As the volume and complexity of sustainability data continue to grow, AI and data analytics will be crucial for organizations to effectively collect, analyze, and make sense of the data.
One prediction is that AI-powered systems will become increasingly sophisticated and will be able to analyze a broader range of data, including unstructured data such as text and images. This will enable organizations to gain a more comprehensive understanding of their sustainability performance and identify new opportunities for improvement.
Another prediction is that AI and data analytics will enable organizations to monitor and predict the impact of their actions on the environment and society. This will allow organizations to make more informed and sustainable decisions and to set more ambitious sustainability goals.
Additionally, AI and data analytics are expected to enable organizations to communicate their sustainability solutions more effectively to stakeholders, including customers, investors, and regulatory bodies. This will increase transparency and accountability and help organizations build trust with stakeholders.
Overall, AI and data analytics have the potential to revolutionize the way organizations approach sustainability data management and will be essential for organizations to effectively address the sustainability data challenge and promote sustainable development in the future.
Bottom line: AI and data analytics have already started playing a major role in addressing the sustainability data challenge, and in the future, it will become indispensable
In conclusion, AI and data analytis have the potential to play a significant role in addressing the sustainability data challenge. Additionally, it can optimize decision-making; for example, it can help your organization find the most sustainable solutions regarding material sourcing, production, and logistics. It can also monitor and predict the impact of different actions on the environment and society. However, you must be aware of the challenges of implementing AI and data analytics solutions and follow best practices for successful implementation. Finally, the future looks bright for AI and data analytics-driven sustainability solutions. As these technologies continue to evolve and improve, they will become an essential tool for organizations to address the sustainability data challenge and promote sustainable development effectively.
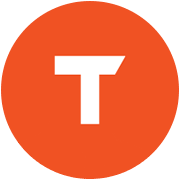
AUTHOR - FOLLOW
Editorial Team
Tredence
Topic Tags