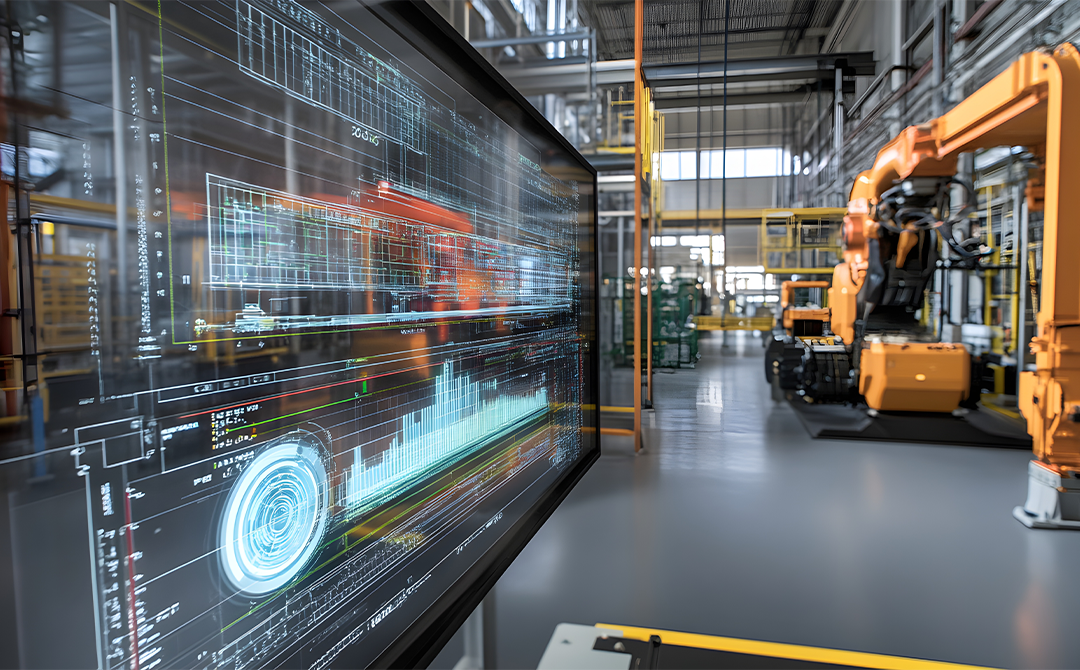
Today's world is exploding with data, and this trend will likely increase significantly in the near future. If not managed well, data will become a liability instead of an asset. As businesses generate 328 million TB of data daily, modernizing data infrastructure is essential to remain competitive and agile (Source: Whatsthebigdata).
Data modernization enables organizations to transform legacy systems into agile, cloud-based platforms that support real-time insights and innovation. By updating data systems, tools, and processes, businesses can improve decision-making, enhance data accessibility, and scale efficiently, ensuring they remain at the forefront of the data-driven age.
In this article, we will explore the need for data modernization, its key components and benefits, common approaches, best practices for success, potential challenges and risks, and real-world case studies of successful transformations.
What is Data Modernization?
Data modernization refers to the process of upgrading an organization's data systems, tools, and workflows to support advanced analytics, and improve scalability and flexibility. This involves moving from outdated, on-premises systems to modern, cloud-based infrastructure that can handle growing data volume and complexity.
The goal of data modernization is to ensure that businesses can not only store and manage data more efficiently but also derive valuable insights in real-time.
By embracing cutting-edge technologies such as cloud computing, artificial intelligence (AI), machine learning (ML), and automation, organizations can achieve a more agile and dynamic data environment. This enables them to access and analyze data faster, drive innovation, and make better-informed decisions.
Data modernization isn't just about technology—it also involves cultural and operational shifts. It requires organizations to adopt new data management practices, rethink their approach to data governance, and implement tools that support data accessibility, security, and collaboration. Ultimately, it empowers businesses to leverage data as a strategic asset, fostering greater agility, competitive advantage, and long-term growth.
Need for Data Modernization
Organizations with legacy systems often face challenges such as limited scalability, high maintenance costs, and poor data accessibility. Traditional infrastructure usually fails to meet modern business needs due to the surge in demand for real-time analytics and big data capabilities.
Big data refers to extremely large and diverse collections of structured, unstructured, and semi-structured data that grow exponentially over time. These datasets are vast and complex in volume, velocity, and variety. They require specialized tools, as traditional data management systems cannot store, process, and analyze them effectively.
Data modernization is necessary to streamline data processes, improve operational efficiency, and empower businesses to leverage data for strategic advantage. It enables companies to break free from the limitations of legacy systems, ensuring they can manage and analyze complex data at scale. As the demand for more sophisticated data insights grows, modernized systems allow businesses to remain agile, innovate faster, and make better-informed decisions.
According to Gartner, more than 50% of enterprises will use industry cloud platforms for business initiatives by 2028, indicating a growing trend toward adoption of cloud based solutions for data modernization. This shift highlights the need for organizations to adapt to modern, scalable solutions to stay competitive and future-proof their operations.
Key Components of Data Modernization
Data modernization revamps and enhances an organization's data infrastructure for greater efficiency and insights. The following elements form the backbone of a successful data modernization framework:
-
Data integration: Data integration centralizes and consolidates data from various sources, ensuring seamless access and providing a unified view across the organization. By integrating data from disparate systems, businesses can improve operational efficiency and enhance the quality of insights derived from their data.
-
Data cleansing and quality: This improves data accuracy, completeness, and consistency by identifying and resolving errors or inconsistencies. This ensures that the data used for analysis is trustworthy, which in turn leads to reliable insights and more informed decision-making.
-
Data warehousing: Data warehouse stores integrated data in a modern, scalable repository. It supports advanced analysis by providing a single, accessible location for all the organization's data. A well-structured data warehouse enables faster data retrieval, making it easier for teams to access critical information on demand.
-
Advanced analytics and Business Intelligence (BI): These tools enable data-driven decision-making by analyzing real-time and predictive data, helping organizations improve ROI and make timely business decisions.
-
Cloud computing and big data technologies: This enables organizations to manage large volumes of data efficiently. Scalable cloud solutions enhance processing power and flexibility, allowing businesses to store, process, and analyze vast datasets without being constrained by traditional infrastructure.
-
Data security: Data security ensures the protection of data through robust policies and controls, safeguarding against breaches and ensuring compliance with privacy regulations. This instills trust in the organization’s data management practices and mitigates risks related to data vulnerabilities.
These key components lay the foundation for a robust data modernization strategy, enabling organizations to harness the full potential of their data. In the next section, we'll explore the significant benefits that data modernization can bring to your organization, including enhanced decision-making and operational efficiency.
Benefits of Data Modernization
Data modernization brings a host of advantages that not only streamline IT infrastructure but also drive tangible improvements in overall business performance.
-
Enhanced decision-making: Access to real-time insights empowers teams to make predictive analytics and data-driven decisions. This allows businesses to act faster and more effectively, improving operational outcomes and aligning strategies with market needs.
-
Cost efficiency: Businesses can significantly lower maintenance costs by reducing their reliance on legacy systems. Modern infrastructures are more cost-effective to manage, freeing up resources for innovation and growth while improving financial and human capital allocation.
-
Improved data security and compliance: Modern systems offer enhanced security features, ensuring sensitive data is protected from breaches. Additionally, they help organizations comply with regulatory requirements, reducing legal risks and maintaining customer trust.
-
Scalability and flexibility: Modern data systems are designed to grow with the business. As data volumes and business needs evolve, these systems can easily scale to accommodate future demands, ensuring businesses remain adaptable and future-ready.
-
Increased innovation: With access to advanced analytics and big data tools, businesses can uncover insights that drive innovation, product development, and customer engagement. This enables companies to maintain a competitive edge in a rapidly changing market.
With these benefits in mind, businesses can explore the most effective approaches to data modernization, ensuring they stay ahead in a rapidly evolving digital landscape.
Common Data Modernization Approaches
Data modernization offers various approaches tailored to organizational goals, resources, and technical landscapes. Choosing the right approach ensures a seamless transition to efficient and scalable data ecosystems. Here’s a closer look at each method, when and why to use it, and how it supports modernization.
1. Lift-and-shift migration
This approach involves relocating legacy data and applications to a modern environment, such as the cloud, without altering the existing architecture or code.
When and why to use:
-
Suitable for organizations seeking a quick and cost-effective migration.
-
Ideal for businesses that need to minimize downtime and disruption.
-
Often used as a stepping stone for further optimization after migration.
How it helps: Lift-and-shift ensures data and workloads are securely moved to modern platforms, allowing organizations to benefit from the scalability and reliability of cloud infrastructure. However, to maximize the advantages of modernization, additional optimizations—such as adopting cloud-native features—may be required.
2. Replatforming
Replatforming involves making selective adjustments to applications and data to leverage cloud-native capabilities while maintaining core functionality.
When and why to use:
-
Ideal for organizations aiming to balance performance improvement with cost management.
-
Suitable for those wanting to modernize critical systems without a complete overhaul.
How it helps: By enabling the use of cloud-native services, re-platforming enhances application performance, reduces operational costs, and prepares organizations for more advanced data use cases, such as real-time analytics. It strikes a balance between innovation and operational continuity.
3. Data re-architecting
This comprehensive approach involves redesigning the data architecture to fully embrace modern technologies.
When and why to use:
-
Best for organizations with long-term goals that require scalability, flexibility, and advanced data capabilities.
-
Suitable for businesses aiming to future-proof their data environments or adopt cutting-edge analytics and AI tools.
How it helps: Re-architecting builds a robust, modern data framework that supports complex workloads, real-time analytics, and seamless integration with emerging technologies. While resource-intensive, it provides the foundation for sustained innovation and business growth.
4. Automation-driven modernization
This approach uses automated tools to modernize data systems, streamlining processes such as data migration, integration, and analytics.
When and why to use:
-
Suitable for organizations seeking efficiency and accuracy in their modernization efforts.
-
Ideal for those with extensive manual processes prone to errors or delays.
How it helps: Automation minimizes human error, reduces operational overhead, and accelerates data transformation. By automating repetitive tasks, businesses can focus on strategic objectives while ensuring consistency and scalability in their modernization initiatives.
Choosing the right approach
Selecting the right data modernization strategy requires a thorough evaluation of an organization’s existing infrastructure, business objectives, and resource availability. For example:
-
Lift-and-shift suits businesses looking for immediate scalability.
-
Replatforming is effective for leveraging cloud-native capabilities with minimal disruption.
-
Re-architecting is essential for forward-thinking organizations seeking a robust, future-ready framework.
-
Automation benefits those aiming for efficiency and accuracy in large-scale projects.
By aligning the approach with strategic priorities, organizations can unlock the full potential of data modernization, ensuring a smoother transition to modern data environments and sustainable growth.
Best Practices for Successful Data Modernization
Building a resilient organization through data modernization requires adherence to best practices that streamline processes and boost efficiency, enabling data-driven decision-making.
Organizations should consider the following best practices to maximize the value of a data modernization strategy:
-
Define clear objectives: Establish specific goals for data modernization, such as improving analytics capabilities, reducing operational costs, or enhancing customer experiences. Clear objectives guide the strategy and help prioritize initiatives.
-
Engage stakeholders early: Collaborate with stakeholders across departments to ensure alignment and buy-in. By engaging leaders and users early in the process, organizations can ensure the modernization strategy meets organizational goals and addresses key pain points.
-
Prioritize data quality: Data integrity is critical for a successful modernization process. Regular data cleansing and validation should be incorporated into the migration process to ensure high-quality data that can generate actionable insights.
-
Invest in scalable solutions: Select modern data tools and platforms that can evolve with your organization’s needs. Scalable solutions are essential, especially when dealing with big data and complex analytics workloads that will only grow over time.
-
Monitor progress continuously: Use metrics to evaluate the success of modernization efforts. Ongoing monitoring allows organizations to assess whether they are meeting objectives, identify areas for improvement, and adapt the strategy as needed to meet future challenges.
By following these best practices, organizations can successfully navigate the data modernization journey and unlock the full potential of their data. However, it is important to remain mindful of the potential risks and challenges that may arise along the way.
Data Modernization: Potential Challenges, Risks, and Overcoming Them
Data modernization is a cornerstone of digital transformation, enabling organizations to unlock new opportunities, enhance operational efficiency, and remain competitive. However, it is not without challenges. Understanding these hurdles and implementing strategies to address them is crucial for a smooth and successful transition.
1. Data Security Concerns
Migrating sensitive data to modern systems, especially cloud platforms, exposes organizations to potential security vulnerabilities. Cyber threats, unauthorized access, and non-compliance with data protection regulations are significant risks. Implementing robust encryption, multi-factor authentication, and access control mechanisms can mitigate these concerns.
Additionally, organizations should invest in continuous monitoring and threat detection tools to safeguard their data environments. Adhering to compliance standards such as GDPR (General Data Protection Regulation) or HIPAA (Health Insurance Portability and Accountability Act) is also vital to prevent regulatory penalties.
2. Data Compatibility Issues
Integrating legacy data with advanced systems often poses compatibility challenges. Legacy systems may use outdated formats or lack standardization, complicating the transition to modern platforms. This can lead to data inconsistencies or inaccuracies that hinder decision-making.
To address this, organizations can employ advanced data transformation and validation tools, which standardize data formats and ensure seamless integration. Collaboration between IT teams and data professionals is essential to resolve compatibility issues without disrupting ongoing operations.
3. Change Management
Introducing modern systems necessitates significant changes in workflows, tools, and organizational culture. Resistance from employees, unfamiliarity with new processes, and a lack of technical expertise can slow adoption. A comprehensive change management strategy is essential to address these challenges.
Organizations should provide extensive training programs tailored to different roles, ensuring employees are equipped to use new tools effectively. Clear communication about the benefits of modernization and how it aligns with organizational goals can foster buy-in and reduce resistance. Establishing dedicated support teams to guide employees during the transition can further smooth the process.
4. High Initial Costs
Data modernization involves substantial upfront investment in infrastructure, software, and expertise. For many organizations, these costs may seem prohibitive, particularly in industries with tight budgets. However, the long-term benefits of modernization—such as improved scalability, enhanced analytics capabilities, and reduced operational costs—often justify the investment.
Organizations can adopt a phased implementation approach to manage these expenses, prioritizing critical areas with the highest ROI potential. Cloud-based solutions and pay-as-you-go models can also help reduce the financial burden by aligning costs with usage.
A proactive and strategic approach is crucial for addressing the risks of data modernization. Conducting a thorough risk assessment, developing a robust roadmap, and involving stakeholders at all levels can significantly reduce potential pitfalls. Partnering with experienced data modernization providers can provide access to proven methodologies, ensuring a smoother transition.
Organizations can mitigate risks and create a resilient, future-ready data ecosystem by addressing these challenges head-on. This enables them to harness the full potential of their data, driving innovation, scalability, and sustained growth in an increasingly data-driven world.
Case Studies of Successful Data Modernization
Data modernization has fueled groundbreaking innovations and applications, empowering organizations across industries to unlock new possibilities and drive greater efficiency. The following case studies highlight some of these innovations:
Streamlining Data Migration for a Multinational Food and Beverage Giant
A prominent U.S.-based multinational food and beverage company operating in over 200 countries encountered difficulties due to its dependence on outdated systems and on-premises databases. These legacy systems were costly to maintain and impeded performance enhancements. In pursuit of cost efficiency and modernized operations, the company opted to transition its on-premises databases to a cloud-based architecture.
Tredence’s data migration and engineering team developed a three-phase strategy utilizing Azure. The initial phase focused on re-coding UNIX scripts into Azure Synapse to facilitate seamless data ingestion. The next step involved creating reusable frameworks and a comprehensive data testing solution, which cut QA efforts by 90%, ensuring a swift and accurate migration process. Finally, Power BI dashboards were created on the Synapse layer, providing actionable insights for business operations.
This comprehensive solution standardized processes minimized data flow time and enhanced overall performance, equipping the client with a modern, scalable data platform.
Accelerating Data Migration for Global Consumer Products Leader
A leading manufacturer and marketer of cleaning, disinfectant, and personal care products in Oakland, California, sought to modernize its data infrastructure.
With operations in over 20 countries, the company initiated a project to consolidate its enterprise data lake, focusing on enhancing supply chain and retail operations. It aimed to migrate essential enterprise data to the Azure platform, phasing out legacy systems and minimizing reliance on manual processes to accelerate dashboard turnaround times.
Tredence assessed the client’s data landscape and implemented a Delta Lake architecture for batch and near-real-time data migration on Azure. This automated approach streamlined three key data systems: manual data sources, SAP data, and the Azure Synapse database.
The transformation reduced business report execution time by 30 percent and eliminated dependencies on outdated systems. The solution enabled the client to integrate and process data more effectively, allowing it to make well-informed decisions across supply chain, retail, and R&D functions. This improved the company’s operational efficiency and agility.
Enhancing Data Modernization with Tredence
Data modernization is vital for organizations aiming to achieve agility, foster innovation, and gain a competitive advantage. It enables businesses to grow by effectively leveraging data.
Businesses can adopt a comprehensive data modernization strategy and leverage tools for automated data modernization and analytics. This can unlock new insights, reduce operational costs, and help them stay ahead of the competitive curve.
Tredence experts, services, and accelerators enable you to achieve your goals with data migration and modernization programs faster.
Leverage Tredence’s services to address complex issues, gain fresh insights into your business, and generate new cost savings and revenue opportunities. Harness Tredence’s expertise in migrating and modernizing your data and creating new analytical capabilities.
Contact Tredence today to unlock actionable insights and gain a competitive edge for your business.
FAQs
Q1: What are the common challenges that businesses face during data modernization?
A: Major challenges include data security concerns, compatibility issues with legacy systems, high initial costs, and resistance to change.
Q2: Can data modernization improve regulatory compliance?
A: Yes, implementing a robust data governance framework helps maintain data integrity, security, and compliance with industry regulations. Automated data workflows and regular audits ensure data practices align with legal and regulatory standards.
Q3: How does data modernization support advanced analytics and AI?
A: Data modernization provides the foundation to harness advanced analytics and AI by organizing and optimizing data for machine learning models and predictive insights.
Q4. Why is data modernization important for businesses?
Data modernization is crucial because it enables businesses to move from outdated legacy systems to scalable, cloud-based infrastructures. This transformation enhances data accessibility, supports real-time insights, and improves decision-making, helping companies stay competitive and agile in an increasingly data-driven world.
Q5. What are the key components of a successful data modernization strategy?
A successful data modernization strategy includes upgrading data systems to cloud platforms, improving data management and governance practices, implementing automation tools, and leveraging technologies like AI and machine learning. This multi-step process helps businesses optimize data usage, enhance analytics capabilities, and ensure scalability for future growth.
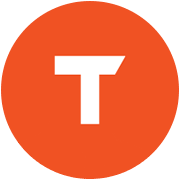
AUTHOR - FOLLOW
Editorial Team
Tredence