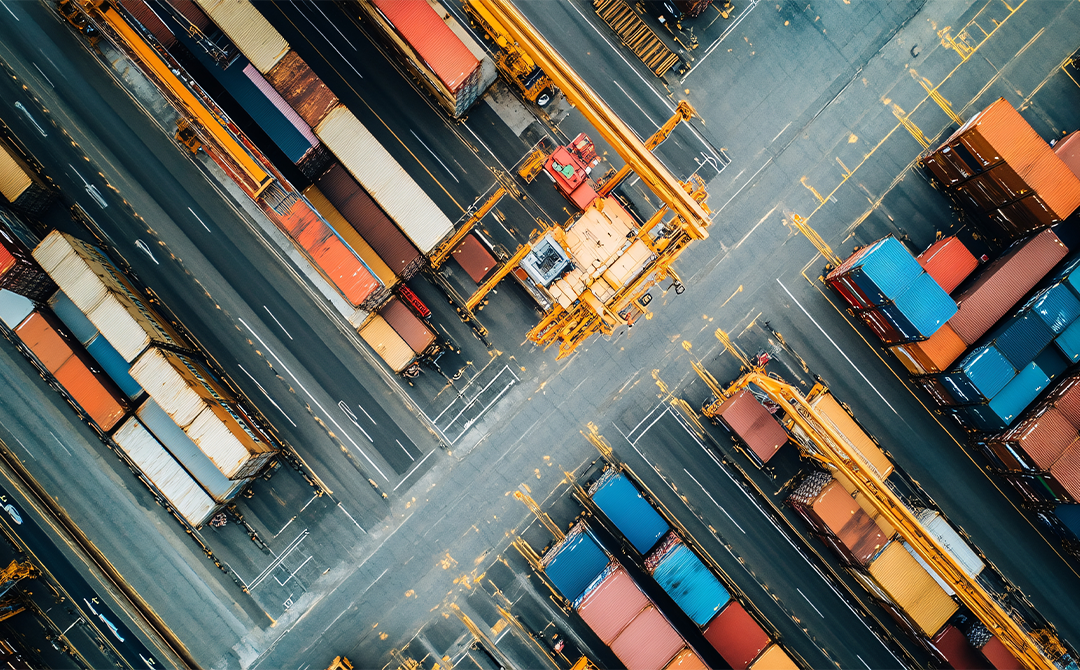
Imagine foreseeing supply chain disruptions before they occur, ensuring your products reach the right place at the right time, every time. With supply chain analytics, this vision becomes a reality, empowering enterprises to anticipate challenges and optimize operations for unmatched efficiency.
Supply chain analytics, or SCM analytics, involves leveraging data-driven insights to transform data into actionable strategies. The global supply chain analytics market is projected to reach $33.45 billion by 2032, expanding at a CAGR of 17.1% from 2024 to 2032.
Supply chain disruptions directly impact profitability of organizations. Therefore, mastering supply chain data analytics is not just an operational upgrade, but a key requisite for driving sustainable growth.
What is Supply Chain Analytics?
Supply chain analytics involves using advanced data analysis techniques to monitor, optimize, and forecast supply chain activities. It helps businesses improve efficiency, reduce costs, and make data-driven decisions by providing insights into inventory, logistics, demand planning, and overall supply chain performance.
Businesses leverage supply chain management analytics to collect data across the supply chain and apply methods such as statistical analysis, predictive modeling, and machine learning to uncover insights.
Supply chain analytics offers the following benefits:
- Reduced costs: Businesses can identify inefficiencies and reduce operational costs by analyzing supply chain data.
- Improved efficiency: Supply chain analytics provides insights into inventory management and demand forecasting, leading to optimized operations.
- Enhanced decision-making: With access to real-time data and predictive analytics, decision-makers can make informed choices swiftly.
According to a study, a 50% reduction in the cost of the supply chain leads to doubling of profits.
Supply chain analytics comprises different types, with each playing a unique role in transforming data into actionable insights.
- Descriptive analytics provides insights into historical data, helping organizations understand past performance.
- Predictive analytics uses statistical models to forecast trends and demands, enabling proactive decision-making.
- Prescriptive analytics recommends actions based on data analysis to optimize supply chain processes.
- Diagnostic analytics investigates data to uncover the reasons behind past outcomes, providing insights that inform future strategies.
How Supply Chain Analytics Works
By transforming the supply chain into data-driven steps, companies gain real-time insights and leverage predictive models to enhance efficiency. This approach enables proactive decision-making, reduces operational risks, and drives continuous improvements across supply chain processes.
Here’s how supply chain analytics typically works:
- Data collection: Data is gathered from various sources within the supply chain, including inventory systems, sales records, supplier databases, and customer orders.
- Data cleansing and integration: Data cleansing ensures accuracy by removing duplication and errors, while integration allows a comprehensive view of supply chain activities.
- Data analysis: In this step, data scientists and analysts apply statistical models, machine learning algorithms, and other analytical techniques to interpret the data.
- Predictive modeling: Predictive modeling helps forecast supply chain scenarios using historical data and advanced algorithms.
- Visualization and reporting: The results from data analysis and modeling are presented through dashboards and reports, simplifying complex insights for key decision-makers.
- Actionable insights and implementation: Insights derived from analytics are used to develop strategic plans and operational adjustments.
Role of Data in Supply Chain Analytics
Data quality refers to measuring data’s accuracy, completeness, and relevance for its purpose. In supply chain analytics, high-quality data ensures reliable insights, helping organizations make informed decisions, optimize operations, and reduce costs. Data is the backbone of supply chain analytics, providing critical insights to streamline operations and drive strategic decisions.
It encompasses information from various sources, such as inventory levels, customer demand, supplier lead times, production rates, and transportation metrics, with each contributing to a detailed view of the supply chain. Advanced tools like AI and machine learning enable real-time data processing, ensuring swift responses to changing conditions.
Additionally, predictive analytics relies on historical and real-time data to forecast demand patterns, prevent disruptions, and improve supply chain resilience. Data integration across systems ensures a unified approach, breaking down silos for better collaboration and visibility.
Effective data usage in supply chain analytics translates to reduced costs, increased efficiency, and a more resilient, responsive supply chain capable of adapting to market changes. According to a Gartner report, 50% of organizations will adopt modern data quality solutions by 2024 to better support their digital business initiatives.
By investing in robust data management practices and leveraging advanced analytics, companies can unlock the full potential of their supply chains, achieving both operational excellence and strategic growth.
4 Types of Supply Chain Analytics
Supply chain analytics is a cornerstone of modern supply chain management, enabling businesses to unlock insights and drive efficiency. Here are the four key types of supply chain analytics and how they support decision-making:
1. Descriptive Analytics
Descriptive analytics answers the question, "What happened?" It gathers historical and real-time data to capture supply chain performance. Metrics like inventory levels, order accuracy, and delivery timelines are analyzed to identify patterns and trends. This type of analysis helps companies understand their current state, monitor KPIs, and detect anomalies that may require immediate attention.
2. Diagnostic Analytics
This type goes further by addressing, "Why did it happen?" Diagnostic analytics dives deeper into the root causes of supply chain issues, such as delays, cost overruns, or inventory shortages. Using data mining and statistical analysis, companies can uncover hidden inefficiencies and bottlenecks, enabling targeted interventions to resolve them.
3. Predictive Analytics
Predictive analytics forecasts future outcomes by answering the question, "What might happen?" Businesses can anticipate demand fluctuations, identify potential disruptions, and predict inventory needs using machine learning and AI algorithms. This helps minimize risks, optimize production schedules, and improve demand planning, leading to a more resilient supply chain.
4. Prescriptive Analytics
Prescriptive analytics provides actionable recommendations by answering the question, "What should we do?" This advanced form of analytics combines predictive insights with optimization algorithms to suggest the best course of action. For instance, it can recommend optimal inventory levels, logistics routes, or supplier relationships to maximize efficiency and minimize costs.
Each type of supply chain analytics plays a critical role in enabling data-driven decision-making. Together, they empower companies to build smarter, more responsive supply chains that align with dynamic market demands and customer expectations.
Benefits and Challenges of Implementing Supply Chain Analytics
Supply chain analytics offers significant advantages, empowering organizations to enhance their supply chain operations and drive better business outcomes.
- Cost savings: Analytics can identify areas where costs can be minimized, resulting in significant savings.
- Optimized inventory: Companies can reduce excess inventory and associated holding costs with better demand forecasting.
- Improved supplier relationships: Analytics fosters better supplier communication and collaboration, enhancing supply chain resilience.
According to a McKinsey report, companies that fully leverage supply chain analytics can reduce errors by 20% to 50% and automate up to 50% of workforce management tasks.
Supply chain analytics offers transformative potential; however, organizations often face challenges that complicate effective implementation.
- Data silos: Disparate data sources or data silos can hinder comprehensive analysis and insights.
- Data quality issues: Poor data quality can lead to inaccurate forecasts and misguided decisions.
- Resistance to change: Employees may hesitate to adopt new technologies and processes.
Best Practices for Effective Supply Chain Analytics
Effective implementation of supply chain analytics demands a strong focus on best practices that improve data accuracy, usability, and overall impact throughout the organization.
- High data quality: Regularly cleanse and validate data to eliminate errors and inconsistencies. High-quality data is the foundation of accurate analysis and reliable insights, driving better decision-making.
- Scalable analytics solutions: Select tools that can expand alongside the company’s evolving needs. Scalable solutions allow an organization to grow its analytics capabilities without costly overhauls.
- Data-driven culture: Encourage employees at all levels to embrace data in their daily decisions. A culture prioritizing data-driven insights fosters more informed and strategic actions across the organization.
Tools and Technologies for Supply Chain Analytics
Various tools and technologies empower supply chain advanced analytics by automating data collection, analysis, and forecasting. Leveraging these resources enhances an organization's capacity to effectively perform supply chain advanced analytics, driving improved operational performance and strategic alignment.
- Machine Learning (ML): ML enables predictive modeling and demand forecasting, allowing organizations to anticipate market trends.
- Artificial Intelligence (AI): AI-powered tools can detect patterns and anomalies, enabling quick adjustments in supply chain operations by modernizing and optimizing the supply chain through connected intelligence and data analytics.
- IoT sensors: IoT sensors collect real-time data from various points across the supply chain. This continuous flow of data supports timely adjustments to avoid disruptions.
- ERP software: This software integrates and manages data across the supply chain processes, enhancing visibility and coordination.
- Supply Chain Control Tower: Supply Chain Control Tower (SCCT) provides all stakeholders with real-time visualizations of various supply chain functions and their overall health through tailored interfaces and automated alerts
Use Cases of Supply Chain Analytics
SCM analytics can be applied across various industries, with notable use cases, including:
- Consumer Packaged Goods (CPG): CPG companies utilize supply chain analytics to handle changing demand, monitor distribution networks, and improve inventory replenishment.
- Manufacturing: Manufacturers use supply chain analytics to optimize production, reduce waste, and improve efficiency. Analyzing demand, logistics, and supplier performance ensures just-in-time delivery, quality control, and cost efficiency.
- Retail: Retailers use supply chain analytics to optimize inventory management and enhance customer experiences through demand forecasting, especially during festive sales, to manage seasonal supply change crises with analytics and data science.
- Healthcare: Healthcare organizations use AI-driven supply chain transformation to streamline their supply chains for pharmaceuticals and medical devices and ensure timely delivery.
Thus, businesses in diverse industries can leverage supply chain analytics to drive efficiencies, adapt to market changes, and enhance their competitive advantage.
Boosting Supply Chain Efficiency and Profitability with Tredence
Supply chain analytics is an indispensable tool for organizations aiming to improve their supply chain performance. By harnessing the power of data and advanced analytics, businesses can reduce costs, enhance efficiency, and drive innovation. Beyond operational benefits, it enables companies to anticipate market changes, enhance customer experiences, and foster resilience in the face of disruptions.
As the supply chain management landscape evolves, companies adopting supply chain analytics and related methodologies will set themselves up for sustained success. Those who integrate advanced analytics into their strategies will gain a competitive edge, turning their supply chains into value-generating assets.
To boost profitability with insight-driven, agile supply chain analytics services, contact Tredence and discover how it can help your organization in the data-driven age.
FAQs
Q1: ) What is the primary goal of supply chain analytics?
The main aim of supply chain analytics is to enhance operations by boosting efficiency, cutting costs, and improving decision-making throughout the supply chain. It does this by utilizing data-driven insights to forecast demand, manage inventory effectively, and optimize logistics.
Q2: ) What is the difference between supply chain management and supply chain analytics?
Supply chain management is centered on the strategic and operational aspects of transporting goods and services from their source to the end consumer. On the other hand, supply chain analytics employs data and advanced technologies to evaluate these processes, leading to improved decision-making and operational efficiency.
Q3: ) In what ways can supply chain analytics increase business revenue?
Supply chain analytics helps pinpoint inefficiencies, lower operational costs, and maintain optimal inventory levels, all of which have a direct effect on profitability. Additionally, it boosts customer satisfaction by enhancing delivery times and aligning production with market demand.
Q4: ) What does the future hold for supply chain analytics?
The future of supply chain analytics is set to be shaped by advanced technologies such as AI, IoT, and blockchain, which facilitate real-time tracking, predictive analytics, and greater transparency. These advancements will contribute to more agile, resilient, and customer-focused supply chains.
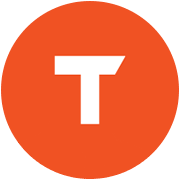
AUTHOR - FOLLOW
Editorial Team
Tredence
Next Topic
From Design to Production: How Generative AI is Reshaping Smart Manufacturing
Next Topic