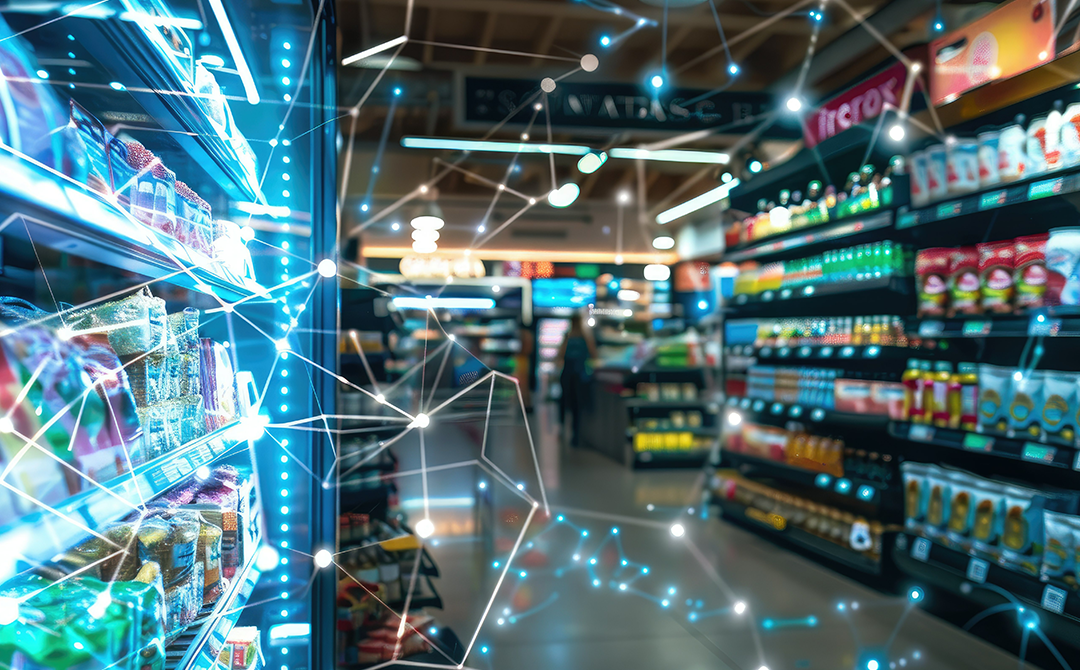
In today's globalized marketplace, the diversity of consumer preferences and buying habits has rendered the "one-size-fits-all" approach to product offerings obsolete. Localization has emerged as a critical strategy for businesses aiming to meet the unique demands of different markets, supporting the evolution of the approach from "We cater to everyone " to "We understand what each customer needs.”
This blog delves into the significance of localized assortment planning, its benefits, challenges, and solutions. Moreover, it highlights how localization will be the foundation for next-generation AI-driven assortment planning in retail, propelling the industry forward in 2024 and beyond.
Why Localized Assortment Planning Matters
Localized assortment planning offers myriad benefits that directly impact retailers' financial performance and long-term viability. By adopting a more analytical approach to assortment management, retailers can unlock significant improvements across multiple areas.
- Understanding Local Demand
Consumers’ preferences are shaped by demographics, cultural nuances, and purchasing habits. According to the Forbes 40 Stats On Digital Transformation And Customer Experience report, 75% of consumers are more likely to buy from retailers that offer personalized product recommendations. Localized assortments ensure products resonate with local customers, fostering a deeper connection and higher satisfaction. Moreover, 76% of global consumers indicate that they prefer to buy from brands that source local ingredients, underlining the importance of tailoring product offerings to local markets
- Sustainable Competitive Edge
Contemporary consumers prioritize choice, opting to patronize multiple stores rather than adhering to loyalty to a single outlet. On average, individuals frequent 2.9 distinct stores weekly to fulfill their shopping needs. However, amidst this inclination for variety, accessibility, convenience, and the allure of comprehensive one-stop shopping experiences reign supreme. Given different markets experience seasons, holidays, and events at varying times, a localized approach allows retailers to adapt product offerings efficiently, ensuring relevance and timeliness. Gartner predicts that by 2025, retailers that adapt their product assortments to local events will see a 20% increase in conversion rates.
- Driving Revenue Growth
One of the primary advantages of localized assortment planning lies in its ability to drive revenue growth through strategic product selection. By tailoring assortments to match local demand, retailers can attract a broader customer base and stimulate higher sales volumes. According to McKinsey’s report, retailers could realize an additional 2 to 4% increase in sales through a more customer-centric product portfolio. Furthermore, localized assortment planning fosters deeper customer engagement and loyalty. By curating product offerings that resonate with local preferences, retailers can demonstrate an understanding of their customers' needs, fostering stronger emotional connections and brand loyalty. It can also aid in systematic delisting, allowing retailers to guide customers towards higher-margin products, potentially boosting gross margins by up to 0.5 %.
- Optimizing Inventory Management
By aligning inventory levels with local demand patterns, retailers can ensure product availability while reducing excess inventory holding costs. This optimization of inventory levels not only enhances operational efficiency but also mitigates the risk of lost sales due to stockouts and minimizes the costs associated with excess inventory. Furthermore, improved procurement conditions result from understanding a product's uniqueness and its potential impact on customer loyalty. This knowledge empowers retailers to make informed decisions regarding product delisting or brand removal, enhancing bargaining power with suppliers and potentially lowering procurement costs by up to 3%.
How AI/ML Reduces the Complexity of Localized Assortment Planning
Localized assortment planning is a crucial strategy for retail chains aiming to meet the diverse demands of different markets. However, the journey to achieving effective localized planning while maintaining the benefits of standardized assortments and store layouts is complex and fraught with challenges. Striking a balance between these two approaches is essential to avoid issues such as higher costs, logistical complexities, and disruptions in store layouts while preventing costly out-of-stock and overstocks. In this context, AI and machine learning (ML) are powerful tools to overcome these challenges and optimize retail operations.
Navigating Data Challenges
- Data Integration and Management
The first significant challenge in localized assortment planning is integrating and managing diverse data sources. Retailers need to gather and process vast amounts of data, including internal sales history, inventory levels, product attributes, customer demographics, and external market trends. This process is time-consuming and requires advanced data management capabilities. AI and ML platforms can automate data cleaning, normalization, and integration, creating a comprehensive and actionable data foundation of golden records.
- Data Anonymization
Handling sensitive customer data ethically and in compliance with regulations is another critical challenge. By leveraging cutting-edge techniques such as the implementation of differential privacy within platforms like Databricks Confidential Compute, Google Cloud Confidential space, and Snowflake data clean rooms, retailers can effectively anonymize sensitive customer data. It enables retailers to harness the power of data-driven insights while demonstrating a commitment to safeguarding individual privacy rights.
Effective Planning and Execution
- Tailored Assortments
Creating assortments that cater to the unique preferences of different regions demands flexibility and agility. Retailers must thoroughly understand local needs and trends to avoid overstocking unpopular items and ensure sufficient stock for high-demand products. AI-driven recommendation systems analyze sales history, customer demographics, and external factors like weather to suggest optimal product mixes for each location.
- Supply Chain Adaptation
Adapting the supply chain to respond quickly to local market dynamics is essential. Collaboration with various stakeholders and efficient logistics networks are crucial. AI-driven supply chain control towers provide end-to-end visibility, enhancing resilience and enabling swift adaptation to changes in local demand patterns.
Overcoming Organizational Challenges
- Cultural Shifts and Investment
Transitioning from standardized approaches to localized strategies often requires significant cultural shifts within the organization. This shift entails embracing data-driven decision-making and investing in advanced analytics, planning, and fulfillment systems. The integration of AI and ML can streamline this process by providing clear, actionable insights that guide strategic decisions.
- Continuous Monitoring and Adaptation
Local preferences and market trends evolve rapidly, necessitating continuous monitoring and adjustment of assortments. Competitors' strategies also impact market dynamics, requiring retailers to innovate continuously. AI and ML technologies enable real-time analysis of sales data, customer behavior, and external factors, helping retailers stay ahead of market trends and make proactive adjustments.
Building a Best-in-Class Solution
- Leveraging Market Intelligence
Retailers can enhance their localized assortment planning by leveraging granular market intelligence from providers like NielsenIQ, Kantar, and GWI. These insights help model hyperlocal buying patterns and trends, providing a foundation for informed decision-making.
- Smart Playbooks and AI Recommendations
Smart playbooks offer structured guidance for decision-makers, helping them adjust product mix, pricing, and timing based on algorithmic insights. AI-driven recommendation systems further refine product offerings by suggesting items tailored to individual customer preferences, ensuring shelves are stocked with the right products in the right quantities.
In addition to segmentation and personalization, AI optimization algorithms aid retailers in identifying the optimal assortment mix for each location. By balancing factors such as sales potential, profitability, and inventory constraints, these algorithms ensure that retailers maintain a competitive edge in the market. Furthermore, AI and ML enhance supply chain responsiveness by predicting demand fluctuations, optimizing inventory distribution, minimizing logistics costs, and ensuring timely product availability.
Collaboration in the Age of Gen-AI
As businesses progress through the stages of AI maturity, collaboration becomes increasingly vital. It’s about striking the right balance between hyper-localized and standard assortments. By leveraging secure multi-party computation, retailers can collaborate with external partners, such as suppliers and marketing agencies, without directly sharing sensitive data. This collaborative approach allows for insights generation while ensuring confidentiality and privacy, enabling retailers to make informed decisions together.
At the genesis of this journey lies data mastery, where Tredence’s partnership with Databricks helps leverage Lakehouse and Unity Catalog. Businesses can lay the foundation for advanced AI applications by centralizing and orchestrating diverse data sources. This data mastery sets the stage for the exploration of AI's potential, from prompt engineering with off-the-shelf LLMs to the customization of bespoke models tailored to strategic goals.
Furthermore, collaboration extends to the deployment and operationalization of AI models. After developing and refining assortment planning models, retailers must deploy them within a secure production environment to ensure operational excellence. By democratizing advanced AI through LLMOps, organizations can scale and refine their models, driving operational efficiencies and enhancing the overall shopping experience.
The Way Forward for Localized Assortment Planning
In today's intensely competitive retail environment, relying solely on generic solutions for assortment planning is no longer sufficient. Supply chain leaders and retailers must instead prioritize the development of customized strategies that capitalize on the potential of Gen AI while ensuring robust data security measures to safeguard sensitive customer information.
The current generation of consumers, often called "Green spenders," places a premium on sustainability, significantly influencing their purchasing decisions. McKinsey's report underscores this trend, revealing that products with ESG-related claims have been responsible for 56% of all growth in the past five years, underscoring the increasing demand for sustainable products.
Localized assortment planning, coupled with local procurement, not only caters to regional tastes but also aligns with local sustainability standards. By sourcing products locally, retailers mitigate the carbon footprint linked with long-distance transportation and bolster local economies and producers. As consumers increasingly prioritize environmental responsibility, investing in the integration of art, technology, and social science in retail becomes paramount. Meeting local demand is no longer just a strategic consideration; it's imperative for success.
Therefore, AI and machine learning will only continue to grow in importance for driving localized assortment planning. Leveraging advanced technologies, such as LLMs, will hold immense potential to revolutionize how retailers understand and respond to local preferences, leading to more effective strategies. By harnessing data-driven insights and embracing agile methodologies, retailers can create personalized experiences that deeply resonate with consumers, ultimately driving increased sales and securing long-term success in the market.
Topic Tags