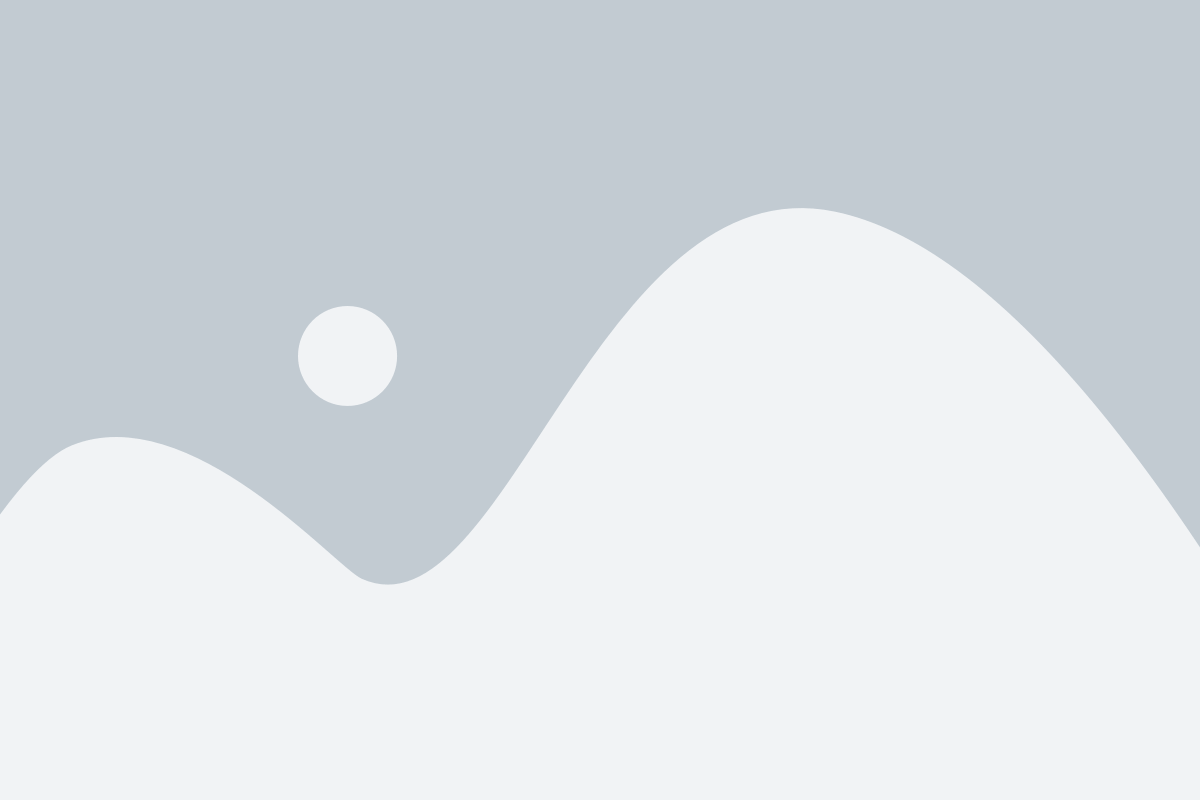
Imagine losing a third of your potential sales because of failure to understand your customers. That is what retailers who ignore data-driven decision-making face.
No wonder organizations that leverage customer insights and behavioral data outperform their peers, experiencing 85 percent higher sales growth and over 25 percent greater gross margins (Source: Forbes). This reinforces a key point: the direct link between analytics and profitability.
Every touchpoint—whether online or in-store—generates valuable insights that can shape marketing strategies, optimize inventory, and increase customer loyalty. Retail marketing analytics is not just an advantage anymore but a necessity.
This article explores retail marketing analytics in depth, highlighting its types, benefits, challenges, best practices, and future trends.
What is Retail Marketing Analytics?
Retail marketing analytics is the process of collecting, analyzing, and interpreting customer data to optimize marketing strategies, improve inventory management, and enhance customer experiences. It helps retailers predict trends, personalize campaigns, and make data-driven decisions that boost sales and profitability.
Picture this: A mid-sized retailer of beauty products was struggling with inconsistent sales. The company implemented retail marketing analytics to transform its operations. The results:
- Enhancement of customer experience: The retailer personalized product recommendations by analyzing customer purchase history and browsing behavior. Shoppers who had abandoned their carts started returning, while repeat customers became more engaged.
- Improvement in inventory management: The company’s skincare products sold better in winter but sat on shelves in summer. The retailer adjusted its stock levels using predictive analytics, ensuring the right products were available without overstocking.
- Optimization of pricing strategies: Competitor analysis revealed that one of its best-selling moisturizers was priced slightly higher than similar brands. By using dynamic pricing, the company made real-time adjustments that encouraged more purchases without sacrificing profitability.
- Increase in sales conversions: The retailer had adopted a generic email marketing strategy earlier. The company then started sending tailored offers after segmenting customers based on their behavior. New shoppers received welcome discounts, while returning customers got loyalty rewards. This boosted customer engagement and sales.
The retailer’s success demonstrates the value of enterprise retail data analytics, making it clear that understanding its key components is crucial for effectively implementing it in your business.
Key Components of Retail Marketing Analytics
Retail marketing analytics transforms raw data into actionable insights, helping retailers drive sales and customer loyalty.
Primary components of retail marketing analytics include:
- Data Collection and Integration: Retailers collect data from multiple sources—POS systems, e-commerce platforms, and loyalty programs—but siloed data limits visibility.
The retailer of beauty products in the example mentioned above struggled to track the influence of online browsing on in-store purchases. By integrating its data, the company discovered that customers who browsed anti-aging serums online often purchased them in-store within a week, enabling more targeted marketing efforts. - Customer Segmentation: Customer segmentation, based on demographics and buying habits, helps deliver more relevant and personalized messaging.
The relaier’s generic promotions resulted in low customer engagement. By segmenting its audience, the company provided exclusive early access to loyal customers, special offers to first-time buyers, and re-engagement discounts to inactive shoppers—driving higher conversion rates. - Campaign Performance Analysis: Understanding which marketing efforts drive results helps retailers optimize budgets. The retailer used to overspend on social media ads without clear returns. The company increased repeat purchases by tracking its email campaign performance, which prompted a shift toward improving customer engagement.
- Demand Forecasting: Predicting demand helps retailers optimize inventory and reduce waste.
The beauty products retailer faced stock imbalances regularly, with some products selling out too soon while others sat unsold. Using predictive analytics, the company adjusted stock levels based on seasonal trends, ensuring the right products were available at the right time.
Now that we understand the building blocks of retail marketing analytics, let us explore the importance of leveraging these components to drive remarkable results.
Importance of Retail Marketing Analytics
Retailers use analytics to refine customer interactions, streamline inventory management, and maximize marketing impact.
Here is how leading brands apply data-driven strategies:
1. Enhancing Customer Experience
Understanding how customers browse, compare, and purchase helps retailers create seamless shopping experiences. AI-powered personalization engines suggest products based on past behavior, thus simplifying decision-making.
Example: Amazon’s recommendation system drives a significant portion of its revenue by analyzing shoppers’ purchase history, browsing behavior, and similar shoppers’ preferences. This helps customers find relevant products quickly, thus increasing conversions.(Source: newamerica).
2. Optimizing Inventory and Reducing Waste
Overstocking leads to markdowns, while stockouts result in lost sales. Retailers leverage analytics to track demand and adjust stock levels.
Example: Walmart’s inventory system continuously analyzes sales patterns, weather forecasts, and regional demand. This ensures high-demand products are replenished efficiently, reducing waste and keeping shelves stocked (Source: Walmart).
3. Maximizing Marketing ROI
Retailers must invest in campaigns that deliver results. Analytics help optimize budgets by identifying the most effective channels and promotions.
Example: Nike uses digital campaigns to leverage customer data and deliver personalized ads. The company tailors promotions, exclusive offers, and product recommendations by tracking interactions across its website and app. This has helped Nike boost customer engagement and drive sales (Source: consumergoods).
These real-world applications demonstrate the power of analytics. However, retailers need to understand the four distinct types of analytics that work in sync to drive success.
Types of Retail Marketing Analytics
Retail marketing analytics is built on four key types, each serving a specific purpose in transforming data into actionable insights. From understanding past trends to predicting future outcomes, these analytics methods help retailers make smarter decisions.
Type of Analytics |
Purpose |
How Retailers Use It |
Pros |
Cons |
Descriptive Analytics |
Analyzes historical data to answer "what happened?" |
Identifies sales trends, customer demographics, and campaign performance. |
Helps identify patterns and track performance. |
Does not predict future outcomes or explain why trends occur. |
Diagnostic Analytics |
Explores "why" certain trends occur. |
Investigates sales drops, customer churn, and marketing performance. |
Pinpoints issues affecting sales and engagement. |
Requires advanced analysis and can be time-consuming. |
Predictive Analytics |
Forecasts future trends using AI and ML. |
Predicts demand, optimizes stock, and personalizes marketing. |
Enables proactive decision-making and inventory management. |
Accuracy depends on data quality; incorrect predictions can mislead strategy. |
Recommends actions based on predictive insights. |
Adjusts pricing, inventory, and marketing strategies. |
Provides data-driven decisions for efficiency and profitability. |
Requires complex AI models and continuous optimization. |
Understanding these analytics types is just the first step—the real impact comes from applying them to enhance customer experience, streamline operations, and maximize profitability. Let us explore these benefits through real-world scenarios.
Benefits of Retail Marketing Analytics
Retailers must balance personalization, efficiency, and security to stay competitive. Here is how data-driven strategies deliver tangible benefits:
1. Customer Personalization
Retailers can create personalized shopping experiences that boost engagement and loyalty by analyzing purchase history and browsing behavior. Instead of generic promotions, they deliver targeted recommendations that resonate with individual customers.
Scenario: A fashion retailer notices high cart abandonment rates for premium handbags. Rather than sending blanket discounts, it identifies and targets returning browsers with personalized styling guides or exclusive, time-limited offers, thus boosting conversions while maintaining product value.
2. Improved Inventory Management
Smart inventory management prevents both stockouts and overstocking. Analytics helps retailers monitor trends, predict demand, and optimize stock levels based on real data.
Scenario: A grocery store uses predictive analytics to address seasonal challenges. It can adjust orders to match demand by analyzing past sales patterns and weather forecasts. Thus, the store can reduce waste while keeping shelves stocked.
3. Smarter Pricing Strategies
Pricing impacts both sales volume and profit margins. Static pricing does not account for demand fluctuations, competitor strategies, or external influences. Retailers use dynamic pricing models to adjust prices in real time, ensuring they remain competitive while maximizing revenue.
Scenario: A hotel chain uses data to adjust room prices based on demand. The prices remain stable on weekdays. The hotel chain analyzes booking trends, local events, and competitor rates as the weekend approaches.
If demand spikes, prices automatically increase to maximize revenue. Conversely, if bookings slow down, limited-time discounts attract last-minute guests, ensuring higher occupancy rates without heavily discounting rooms in advance.
4. Fraud Prevention
Retailers handle thousands of transactions daily, making fraud detection critical. Advanced analytics monitors transaction patterns, identifying suspicious behavior before fraud occurs. By detecting anomalies in real time, businesses reduce chargebacks and financial losses while protecting legitimate customers.
Scenario: An e-commerce platform's system flags an unusual activity: multiple high-value purchases from different locations using one account. By automatically holding suspicious transactions for verification, it prevented fraud while protecting legitimate customers.
Incorporating retail marketing analytics enables retailers to make informed decisions that not only improve operational efficiency but also enhance customer experiences, ultimately driving profitability and long-term success.
Challenges in Retail Marketing Analytics
Retail marketing analytics offers immense benefits, but implementing it comes with challenges that retailers must navigate carefully. From fragmented data to compliance concerns, businesses need the right strategies to overcome these obstacles and maximize the value of their data.
Here are some common challenges faced by retailers and their solutions:
1. Data Silos
Challenge: Retailers collect data from multiple sources such as POS systems, e-commerce platforms, loyalty programs, and third-party marketplaces, These systems often do not communicate. This fragmentation makes it difficult to obtain a complete view of customer behavior and business performance.
Solution: Centralizing data through a unified analytics platform allows retailers to integrate information from all channels, creating a single source of truth. This enables better decision-making and more accurate insights.
Implementation Tips
-
- Invest in a cloud-based data warehouse that consolidates all customer interactions.
- Use API integrations to connect different platforms, such as CRM, e-commerce, and in-store systems.
- Standardize data formats across systems to ensure seamless merging and analysis.
2. Privacy and Compliance Issues
Challenge: Retailers must ensure responsible customer data handling due to the enactment of regulations such as GDPR and CCPA. Data mismanagement can result in hefty fines, legal risks, and loss of customer trust.
Solution: Implementing a strong data governance framework ensures compliance while maintaining transparency with customers about how their data is used.
Implementation Tips
-
- Use privacy-first data collection methods, such as anonymization and opt-in consent.
- Regularly audit data storage and security practices to comply with regulations.
- Train employees on data protection policies to prevent unintentional breaches.
3. High Implementation Costs
Challenge: Advanced analytics tools and skilled data professionals come at a high cost, making it difficult for smaller retailers to compete with industry giants.
Solution: Retailers can start with scalable analytics solutions, prioritizing high-impact areas such as customer segmentation and demand forecasting before expanding.
Implementation Tips
-
- Use SaaS-based analytics tools that offer flexible pricing instead of investing in costly in-house systems.
- Focus on quick wins, such as improving email marketing through data insights, before committing to large-scale infrastructure changes.
- Collaborate with external data analytics firms to reduce the need for an in-house team in the early stages.
4. Accuracy of Predictions
Challenge: Analytics is only as good as the data it relies on. Inaccurate or incomplete data can lead to misleading insights, resulting in poor business decisions.
Solution: Data quality management through rigorous validation and continuous monitoring improves the accuracy of predictive models.
Implementation Tips
-
-
- Regularly clean and standardize data to remove inconsistencies.
- Cross-check analytics outputs with actual sales and customer feedback to refine models.
- Use AI-powered anomaly detection to identify and correct discrepancies before they impact decision-making.
-
Overcoming these challenges allows retailers to fully leverage retail marketing analytics, enabling smarter decisions, improved efficiency, and a stronger connection with customers.
Best Practices for Retail Marketing Analytics
Retailers must balance personalization, inventory efficiency, pricing optimization, and fraud prevention to stay competitive. Data-driven strategies help businesses anticipate customer needs, stock the right products, adjust prices dynamically, and detect fraud before losses occur.
Here is how these best practices play out in real-world applications:
1. Centralize Your Data
Integrating data from all sales channels—online, in-store, and third-party platforms—provides a unified view of customer behavior. Insights remain fragmented without a centralized system, resulting in missed opportunities.
Example: Sephora consolidates customer data across its mobile app, website, and physical stores. This allows seamless omnichannel personalization, such as reminding online shoppers of items tested in-store, leading to increased conversions (Source:etailwest).
2. Leverage AI and ML
AI-driven insights help retailers forecast demand, personalize marketing, and automate decision-making. ML models continuously refine predictions, enabling retailers to adapt in real time.
Example: Zara, a fashion retailer, uses AI to analyze sales data daily and adjust production accordingly. Instead of overproducing seasonal stock, Zara responds to demand shifts quickly, ensuring stores are stocked with high-demand items (Source: digitaldefynd).
3. Focus on Customer Segmentation
Not all customers engage with a brand in the same way. Segmentation based on purchase history, browsing behavior, and demographics allows retailers to create highly targeted marketing campaigns.
Example: Starbucks segments customers based on buying patterns. For instance, regular morning coffee buyers receive exclusive discounts on breakfast items, while occasional visitors get loyalty-based incentives. This drives engagement and encourages repeat purchases (Source: businessmodelanalyst).
4. Monitor Key Performance Indicators (KPIs)
Tracking essential KPIs, such as conversion rates, average order value, and customer retention, helps retailers identify what’s working and where improvements are needed. Retailers that regularly analyze these metrics can optimize marketing spend and refine customer engagement strategies.
Example: Amazon tracks customer lifetime value (CLV) and purchase frequency to refine its recommendation engine. The company ensures a higher likelihood of repeat purchases by personalizing offers based on past behavior (Source: premierecreative).
These best practices offer a strong starting point; however, real-world examples of successful retailers put them into perspective and provide valuable inspiration. Let us explore some standout cases.
Examples of Retail Marketing Analytics
Retailers no longer rely on intuition alone to drive sales. Advanced analytics help track shopper behavior, personalize marketing, predict demand, and optimize product placement.
Here is how these strategies play out in real-world retail environments:
1. In-store Customer Tracking
Understanding customer movement helps retailers design layouts that drive sales. Heatmaps and sensor-based tracking reveal high-traffic areas and shopper behavior, enabling optimized shelf placement.
Real-world application: IKEA places its most in-demand products deeper inside stores. By analyzing foot traffic, it has designed a winding layout that exposes shoppers to more products before making their intended purchase. This has helped the company boost impulse buying and increase average order value (Source: thehustle.co).
2. Personalized Email Marketing
Generic email blasts fail to engage customers. By leveraging purchase history and browsing behavior, retailers send targeted emails that improve engagement and conversions.
Real-world application: Nordstrom engages shoppers by sending personalized emails based on browsing history. Customers receive recommendations or sale alerts if they view designer handbags but do not buy. This increases the likelihood of a purchase (Source: Nordstrom).
3. AI-powered Demand Forecasting
Excess inventory ties up capital and leads to discounts, while running out of popular items results in missed revenue and frustrated customers. AI-driven demand forecasting helps retailers adjust inventory based on seasonality, trends, and external factors.
Real-world application: H&M uses AI to analyze sales data and optimize inventory, ensuring high-demand items are restocked while reducing overstock and markdowns. This data-driven approach improves product availability, boosts sales, and enhances customer satisfaction (Source: The Wall Street Journal).
4. Optimized Product Placement
Strategic product placement boosts visibility and sales. Retailers analyze buying behavior to determine which products to feature at entrances, checkout counters, and high-traffic areas.
Real-world application: Retailers often employ strategic store layouts to encourage impulse purchases. For example, placing high-demand items at the back of the store compels customers to traverse multiple aisles, increasing exposure to other products and prompting unplanned purchases. This layout strategy is commonly used in supermarkets and other retail environments to boost sales.
These real-world examples highlight that retail marketing analytics is not just about numbers. It is about understanding the customer journey on a deeper level and making smarter, more intuitive decisions that ultimately shape the shopping experience and drive long-term loyalty.
Let us now look at the innovative trends shaping the future of retail analytics.
Future Trends in Retail Marketing Analytics
Retail technology is evolving rapidly, and retailers are embracing innovations to enhance customer experiences, streamline operations, and improve security. Industry leaders have already started adopting these emerging trends, setting new standards for the future of retail.
1. AI-powered Hyper-personalization
Retailers are using real-time data to create ultra-personalized customer experiences. AI-driven personalization analyzes real-time behavior, past purchases, and customer preferences to deliver tailored product recommendations, promotions, and shopping experiences.
Leadership position: Amazon uses AI-powered personalization to customize product recommendations, dynamically adjust pricing, and tailor homepage content based on each shopper’s unique browsing and purchasing history (Source: aboutamazon).
2. Augmented Reality (AR) Shopping
Retailers have started to integrate AR for virtual try-ons and immersive experiences. AR allows customers to visualize products in their environment before making a purchase, reducing uncertainty and boosting confidence in online shopping.
Leadership position: IKEA enables customers to view how furniture would look in their homes through its IKEA Place AR app. This allows users to scale and position products virtually before buying (Source: IKEA).
3. Voice Commerce and Chatbots
AI assistants have started helping customers make purchases via voice commands. Conversational AI is transforming retail by enabling hands-free shopping and instant customer support, making transactions faster and more convenient.
Leadership position: Walmart has integrated Google Assistant for voice shopping, allowing customers to add items to their cart and place orders using simple voice commands (Source: Walmart).
4. Blockchain for Data Security
Retailers are using blockchain to enhance data security and transparency. Blockchain provides a tamper-proof system for tracking transactions, preventing fraud, and ensuring the authenticity of products.
Leadership position: Walmart has implemented blockchain to track its food supply chain, ensuring product authenticity and reducing contamination risks by providing full transparency from farm to shelf (Source: Walmart)
By leveraging these cutting-edge technologies, leading retailers are shaping the future of shopping, offering smarter, more engaging, and more secure experiences for consumers.
Transforming Retail with Tredence’s Data-driven Insights
The retail landscape is rapidly evolving, with shifting customer expectations and advancing technology transforming the way we approach shopping experiences. Retail marketing analytics has become indispensable for staying competitive in this dynamic environment.
From enhancing personalization and optimizing inventory to preventing fraud and predicting future trends, data-driven decision-making is no longer optional—it is essential for survival and growth.
Retailers who embrace analytics will be better equipped to meet customer needs, streamline operations, and drive profitability. However, the journey from data to insights is not always straightforward. It requires the right combination of technology, expertise, and strategic vision.
This is where Tredence steps in. Its cutting-edge analytics solutions empower retailers with:
- Real-time data integration across all channels
- AI-driven insights that drive actionable results
- Scalable predictive models tailored to your business
- End-to-end implementation support
Whether you're just starting your analytics journey or looking to enhance your existing capabilities, Tredence has the expertise to help you transform your retail operations. Its proven track record in delivering hyper-personalized customer experiences, accurate demand forecasting, and optimized marketing strategies has helped retailers across the globe stay ahead of the competition.
Ready to harness the full potential of your retail data? Contact Tredence today to discover how its analytics solutions can empower your business to make smarter decisions, drive growth, and stay ahead of industry trends.
FAQs
1. How can retailers start using marketing analytics without heavy investment?
Retailers can begin by leveraging existing data from POS systems, e-commerce platforms, and loyalty programs. Cloud-based analytics tools offer affordable, scalable solutions that help track customer behavior and optimize marketing efforts without large upfront costs.
2. How does AI improve retail marketing analytics?
AI automates data analysis, predicts trends, and personalizes marketing efforts. ML models identify customer patterns, adjust pricing dynamically, and optimize inventory, enabling retailers to make real-time, data-driven decisions.
3. What are the biggest challenges in retail marketing analytics?
Retailers often face data silos, privacy concerns, and inaccurate predictions while adopting retail marketing analytics. Solutions to these challenges include integrating data across all sales channels, ensuring GDPR and CCPA compliance, and continuously refining data quality for better insights.
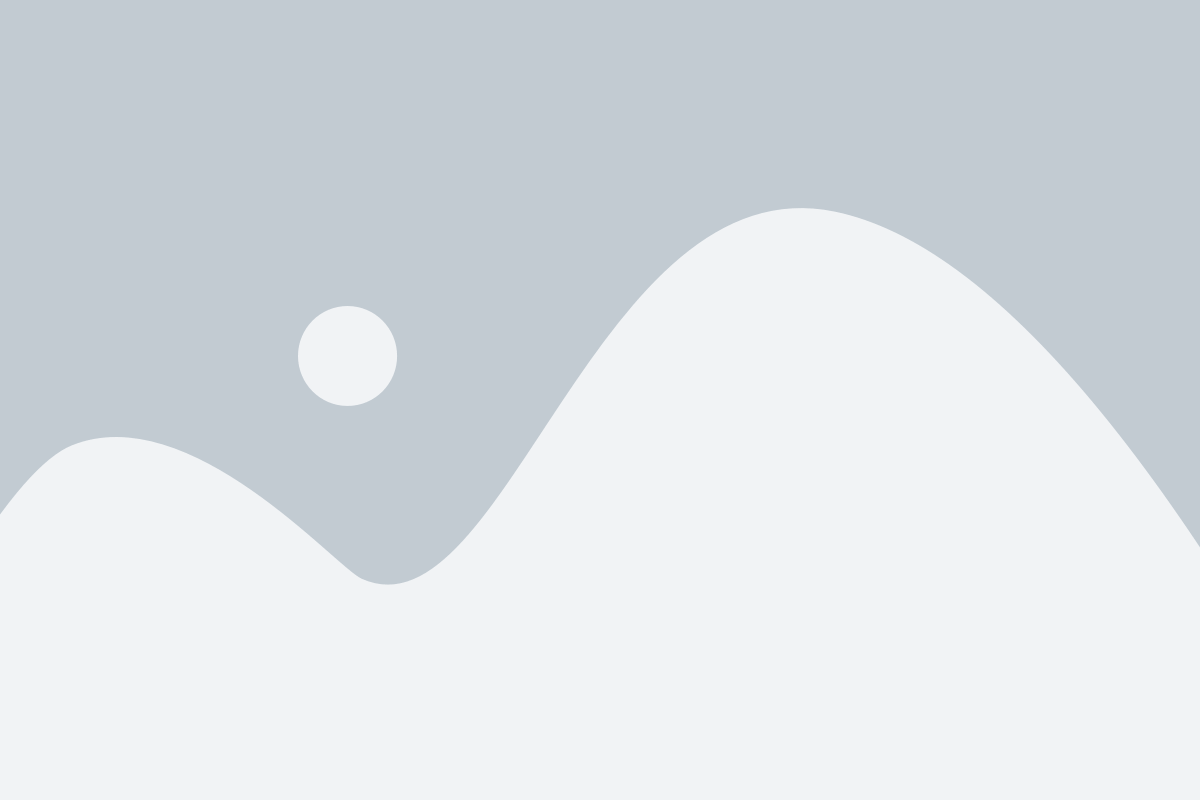
AUTHOR - FOLLOW
Editorial Team
Tredence