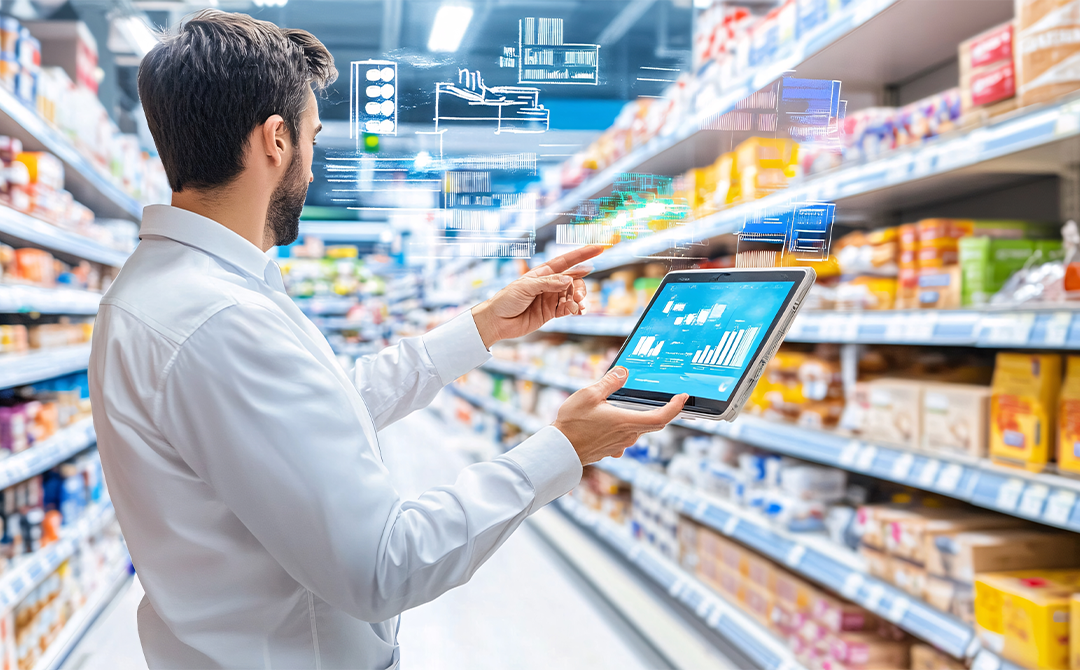
Imagine a retail shop during the holiday, shelves full of goods, customers scanning through the aisles, and a busy staff involved in transactions. How do you stand out in that highly competitive environment? The response: Retail Customer Analytics.
By utilizing customer data, businesses can predict preferences, track trends, and create unique shopping experiences that build loyalty.
78% of consumers become repeat customers if they are given personalized experiences and recommendations. Lack of such strategies may result in customer attrition, poor operations, and stagnation in a dynamic market (Source: McKinsey).
This article discusses retail consumer analytics, the key metrics and tools that drive it, and how it transforms businesses. Whether you are an experienced retailer or a newcomer, this article will help you obtain actionable insights to utilize customer analytics for business growth.
What is Retail Customer Analytics?
Retail customer analytics is the process of analyzing customer data to understand preferences, behaviors, and buying patterns of customers. Retailers can optimize experiences, marketing strategies, and revenue by leveraging data from touchpoints such as in-store visits, online shopping, and loyalty programs.
Target, a U.S.-based retail corporation, uses customer analytics to predict purchasing patterns, such as when a customer is likely to buy baby products. This enables the company to conduct personalized marketing campaigns and improve customer satisfaction, loyalty, and profitability (Source: ResearchGate)
Advancements in AI, predictive analytics, and ML enable retailers to proactively engage customers, thereby giving a competitive edge in the market.
Data management is a precursor to understanding retail customer analytics. A strong data framework is fundamental to providing actionable insights to help make better decisions and engage effectively with customers.
In this light, let’s walk through the role of data in driving retail customer analytics.
Role of Data in Retail Customer Analytics
Data forms the basis of retail customer analytics; however, its worth depends on how it is collected, processed, and analyzed. About half of organizations, or 46 percent, do not use data to gain insights or make decisions.
Poor data management or underutilized data can leave businesses with blind spots. Without robust data practices, retailers may not understand customers' behavior, hence missing profits and losing customer satisfaction (Source: Dataversity)
Untapped Power of Data
Data that seems insignificant can reveal powerful insights when analyzed correctly.
With the right approach, even small details can provide valuable information. These may include:
- Purchase Frequency and Basket Size: Businesses can predict when customers will likely require replenishment by analyzing how often a specific product is bought. This enables targeted marketing.
- Browsing and Abandonment Data: A customer repeatedly viewing a particular item online but not purchasing it might indicate hesitation due to pricing. This insight can trigger personalized discounts or payment plan options to close the sale.
- In-store Foot Traffic: Sensors tracking how customers move through a store can help optimize product placement and enhance overall store layouts, driving better sales performance and in-store customer analytics.
Lululemon uses weather data to optimize inventory management. When a cold snap is expected, it increases stocks of jackets and cold-weather gear, thus ensuring products match customer needs (Source: Ambee).
This approach helps drive sales by aligning supply with demand. When analyzed strategically, it demonstrates how unconventional data sources can become a competitive advantage.
Harnessing diverse data sources is critical for actionable insights. The real value lies in understanding which metrics matter the most.
Let us delve into the key metrics that drive impactful retail customer analytics.
Key Metrics in Retail Customer Analytics
Retailers monitor and track a broad list of KPIs to be competitive in the market. Retail customer analytics is beyond mere data harvesting; it is the extraction of actionable retail consumer insights from data that directs a decision.
Retailers use the following essential metrics to understand customer behavior:
1. Customer Lifetime Value (CLV)
CLV measures the total revenue a customer is expected to bring while being associated with a brand. By analyzing CLV, retailers can identify the most profitable customer segments and adjust their marketing strategies to target them effectively.
2. Customer Retention Rate
This calculates the percentage of customers who continue purchasing from a retailer over some time. High retention rates are often indicative of customer satisfaction and personalized experiences.
3. Average Order Value (AOV)
AOV measures the average amount spent per customer in a single transaction. Increasing AOV enables retailers to generate more revenue without necessarily increasing traffic. It also gives insight into the efficiency of upselling, cross-selling, and promotional offers.
4. Purchase Frequency
This metric tracks how often a customer purchases a given time frame. Higher purchase frequency generally reflects stronger loyalty and higher engagement.
5. Conversion Rate
The conversion rate measures the percentage of website visitors or store visitors who purchase. It helps understand how well a business is turning leads into actual sales.
6. Customer Satisfaction and Net Promoter Score (NPS)
This indicates customer satisfaction levels and the likelihood of the customer recommending the retailer to others. High satisfaction scores correlate with positive customer experiences and directly influence loyalty and retention.
7. Churn Rate
This indicates the percentage of customers who stop buying from a retailer over a period. Churn monitoring helps determine whether there is a problem with customer satisfaction. It could be an early warning signal of deteriorating business performance.
8. Basket Size
Basket size is the total number of items purchased by a customer in a single transaction. Retailers use this metric to assess the effectiveness of their product bundling strategies, promotions, and cross-selling tactics.
Understanding and acting upon these key metrics is an ongoing process. Retailers need to keep refining their strategy through advanced technologies to stay ahead of the curve from a competitive perspective.
The next step is to examine the tools and technologies that help retailers utilize these metrics.
Tools and Technologies for Customer Analytics
The right tools can transform raw data into actionable insights. Retailers can leverage various technologies to understand their customers better and optimize their strategies.
Below are some of the primary tools and technologies used in retail customer analytics:
-
Customer Relationship Management (CRM) Systems
CRMs are used to interact with current potential customers. They gather, store, and analyze customer data, allowing retailers to interpret customer preferences, behaviors, and purchases.
Leading CRM platforms such as Salesforce, HubSpot, and Microsoft Dynamics enable personalized communication and targeted marketing campaigns based on customer insights.
-
Big Data and Cloud Analytics
Big data processes large, structured, and unstructured datasets from various sources, such as social media sites, online shopping portals, and customer feedback.
Tools like Hadoop, Spark, and AWS (Amazon Web Services) allow retailers to store large datasets, uncover hidden information from various data, and execute predictive analytics models that forecast customer behavior.
-
Predictive Analytics Tools
Retailers can use IBM Watson, SAS Predictive Analytics, and Microsoft Azure Machine Learning to forecast customer needs and optimize inventory and marketing efforts, making the right decisions.
These tools help businesses make informed decisions by predicting purchases and churn rates.
-
Customer Data Platforms (CDPs)
CDP helps retailers create a 360-degree profile of each customer, facilitating personalized marketing and improved customer engagement.
CDPs such as Segment, BlueConic, and Tealium allow businesses to track individual customer behaviors across channels, providing valuable insights into their preferences and buying patterns.
-
Heatmaps and In-store Analytics Tools
Understanding how customers move through physical spaces is essential for brick-and-mortar stores. Heatmap tools such as RetailNext and Trax track a customer's movement using sensors, cameras, and data analytics about interactions with in-store displays.
-
Social Media Listening and Analytics
Tools such as Hootsuite, Brandwatch, and Sprout Social enable retailers to track social media trends and analyze customer feedback. Businesses obtain insights about customer perception, emerging trends, and potential marketing opportunities from real-time social media data.
-
A/B Testing and Personalization Engines
Functional testing tools, Optimizely and VWO, help retailers test websites and marketing strategies. Personalization engines, such as Dynamic Yield, customize the shopping experience according to customer behavior, increasing conversion rates and customer satisfaction.
-
Data Visualization Tools
Tableau, Power BI, and QlikView transform complex data into clear, actionable visualizations. These systems enable retailers to track key performance metrics of sales and customer satisfaction more accurately, resulting in quicker, data-driven decisions.
-
Sentiment Analysis Tools
Sentiment analysis tools help retailers understand customers' emotions regarding their products and services.
Retailers can use products such as Lexalytics to analyze customer reviews and social media comments, determining whether the sentiment is positive, negative, or neutral. This helps them identify areas for improvement and pinpoint opportunities to engage their customer base more effectively.
Adopting the abovementioned tools allows retailers to make data-driven decisions, improving customer experiences and operational efficiency. However, selecting the right technology stack can be challenging, as it must align with business objectives and customer needs.
Let us now discuss how customer segmentation can enhance actionable insights.
Segmenting Customers for Better Insights
Customer segmentation is a powerful strategy in retail customer analysis. By dividing customers into distinct groups based on shared characteristics, retailers can tailor their offerings, marketing efforts, and customer experiences to meet specific needs better.
Retailers must consider the following major points when implementing customer market segmentation for better insights:
-
Demographic Segmentation
This segmentation classifies customers based on measurable traits such as age, gender, income, and occupation. Retailers can utilize this information to design relevant marketing campaigns, such as targeting young professionals with products that fit their lifestyles or promoting items specific to a particular gender.
Key factors: Age, gender, income, occupation, and education.
Nike uses demographic data to create personalized offers. It targets young adults with trendy, limited-edition releases and promotes products based on seasonal needs or sports interests (Source: Edrawmind).
-
Behavioral Segmentation
Customers are categorized according to their interactions with the brand, including purchase history, browsing behavior, and loyalty. This method enables retailers to anticipate future actions and provide personalized experiences, thus enhancing customer retention and sales through targeted recommendations.
Key factors: Purchase history, browsing patterns, brand loyalty, and product usage.
Amazon excels at behavioral segmentation by recommending products based on customer personality and analyzing their buying behavior within the app. Its personalized recommendation engine contributes significantly to repeat customer sales (Source: BRM).
-
Geographic Segmentation
Retailers can tailor their product offerings to meet regional demands by concentrating on customers' locations and cultural preferences. For example, they might sell winter coats in colder areas or adjust their inventory to reflect local tastes and trends.
Key factors: Location-based preferences and cultural differences.
A fashion brand may offer different clothing collections in regions with varying climate conditions or local cultural preferences.
-
Psychographic Segmentation
Psychographic segmentation focuses on customers' lifestyles, values, interests, and personality traits. It allows retailers to connect more emotionally, offering products or campaigns that resonate with customer values, such as promoting eco-friendly products to environmentally conscious consumers.
Key factors: Lifestyle, values, interests, and personality traits.
Retailers targeting eco-conscious customers may focus on sustainable product lines that align with customers' values and prioritize sustainability.
-
Transactional Segmentation
This segmentation is based on purchase frequency, order value, and recency metrics. Retailers can enhance loyalty by providing customers with VIP programs, exclusive offers, or rewards, thus ensuring ongoing engagement and profitability.
Key factors: Frequency of purchases, average order value, and recency of purchase.
High-value customers who frequent large purchases can be targeted with VIP programs or exclusive offers. A retail organization used transactional segmentation to calculate their CLV scores.
It used the Recency, Frequency, Monetary (RFM) model to create clusters of similar CLV scores, allowing it to rank clusters by highest CLV and design targeted strategies for each cluster individually (Source: Kaizen Institute).
Analyzing Customer Behavior
Customer behavior analytics is at the core of effective retail customer insights. By analyzing how customers engage with a brand, retailers can anticipate needs, improve experiences, and drive sales.
Here's how a deeper understanding of behavior can lead to actionable strategies:
-
Purchase Patterns
Retailers can study purchase frequency, volume, and recency to predict future buying behavior. This helps increase conversion rates and ensure inventory is appropriately stocked for anticipated demand.
-
Browsing Behavior
Tracking customer interaction with products online helps retailers understand preferences, interests, and purchasing triggers.
eBay leverages browsing behavior data to suggest complementary products based on previous searches, increasing cross-selling opportunities and overall sales (Source: ebay).
-
Sentiment Analysis
Monitoring customer feedback, reviews, and social media sentiment offers valuable insights into their opinions and emotional connection with the brand.
Walmart developed Social Genome, a big data analytics solution that analyzes customers' social media mentions to identify positive and negative sentiments, drive purchase decisions and provide customers with personalized offers and discounts. (Source: ProjectPro).
-
Customer Journey Mapping
Analyzing the touchpoints a customer engages with before purchasing can highlight key moments that influence decision-making.
The Home Depot uses customer journey mapping to identify in-store and online interactions, tailoring marketing strategies to each touchpoint. This holistic view of the customer journey leads to more personalized experiences and improves conversion rates (Source: Renascence).
-
Predictive Analytics
By applying ML algorithms, retailers can predict future customer behavior, including potential churn, repeat purchases, and changes in preferences.
Carrefour, a France-based retailer, employs AI predictive analytics to enhance its business operations. It utilizes Viya, an AI-driven software developed by SAS, to improve inventory management.
This platform gathers data from warehouses, stores, and online channels to effectively forecast demand and manage supply orders. As a result, Carrefour has minimized stock shortages and prevented overstocking in stores and warehouses (Source: InData Labs).
Power of Behavioral Insights
Behavioral insights focus on understanding what customers do, what they prefer, and what motivates them. By examining these patterns, retailers can gather important information to develop strategies that improve customer experiences and promote business growth. Here’s how behavioral insights benefit retailers:
-
Customer Retention: By analyzing customer engagement, retailers can create targeted retention strategies. These may include offering discounts, loyalty rewards, or personalized experiences to encourage repeat business.
-
Sales Growth: Behavioral insights help identify the best times to target customers with special offers, improving the chances of conversion.
-
Enhanced Personalization: Retailers can create highly personalized experiences that resonate with customers based on their unique behaviors, fostering stronger emotional connections.
Understanding these behaviors helps retailers better serve customers; it also enables them to anticipate future needs and offer proactive solutions.
The next section will explore how implementing these insights through customer analytics strategies can help retailers gain a competitive edge in today’s rapidly changing market.
Implementing Customer Analytics Strategies
Retailers can address specific operational, marketing and customer experience challenges through key customer analytics strategies.
Here’s how each strategy addresses specific challenges and how to implement them effectively:
-
Personalized Marketing Campaigns
Generic campaigns often fail to engage customers. Retailers must personalize marketing messages based on customer demographics, purchase history, and browsing behavior.
They must leverage customer segmentation tools and automation platforms to send personalized emails, ads, and offers tailored to individual preferences. Amazon enhances customer experience by recommending products based on purchase and browsing behavior, increasing sales (Source: mana-analytics).
-
Dynamic Pricing
Static pricing models may not help retailers with missed opportunities or product over/underpricing. Real-time dynamic pricing addresses fluctuations in demand, competition, and customer behavior to ensure competitive prices and optimized revenue.
Retailers must use pricing optimization tools such as Prisync or Omnia Retail to monitor competitor prices and demand fluctuations and adjust prices accordingly. Amazon employs dynamic pricing to adjust fares based on real-time demand, balancing revenue generation with customer satisfaction (Source: Pricefy).
-
Inventory Optimization
Overstocking and stockouts result in lost sales and inefficient operations. Retailers must manage their inventory levels as per customer insights to predict demand, thus ensuring optimal inventory levels and reducing waste.
Walmart uses advanced analytics to optimize inventory levels and improve product availability, thus reducing overstocking while meeting customer needs (Source: Walmart).
-
Customer Retention Programs
The revenue spent on retaining an existing customer is less than that spent on attracting a new one. Retailers can check customer behavior patterns to detect high-value customers and develop customized retention strategies.
They can use CRM platforms such as Salesforce or Zoho to analyze customer behavior and loyalty and then formulate customized retention strategies.
-
Customer Feedback Loop
Feedback should be collected at regular intervals to understand new customer needs and pain points. This will help businesses improve their offerings, rectify dissatisfaction, and fine-tune the customer experience.
They can use tools like SurveyMonkey or Qualtrics to collect feedback through surveys and sentiment analysis platforms like Zendesk to measure customer satisfaction and sentiment.
-
Omnichannel Integration
Customers get frustrated when their experiences are inconsistent across online and offline channels. Retailers need to integrate these experiences to create a seamless, personalized customer journey across all areas.
They can invest in integrating omnichannel platforms such as Shopify Plus or Magento to unify inventory, data, and interactions across various sales channels. Retailers can also track cross-channel behavior and personalize the experience accordingly.
-
Predictive Analytics for Churn Prevention
Customer churn is inimical to business growth. Retailers need to resort to predictive analytics to predict when customers may churn. Retailers can use tools, such as IBM Watson or SAS, to develop predictive models that use historical data and customer behavior to identify a churn risk and then take action in that direction.
Implementing the strategies outlined above requires technical and market expertise. Tredence is one of the key leaders providing AI, ML, and customer analytics services and solutions to retail businesses.
Its customer analytics strategies help resolve critical issues in marketing, inventory management, customer retention, and overall customer experience, all of which contribute to sustainable business growth.
In the next section, we’ll explore some of the challenges retailers face when implementing these strategies and how to overcome them effectively.
Challenges in Retail Customer Analytics and How to Address Them
Implementing retail customer analytics comes with various challenges that, if not addressed properly, can reduce effectiveness.
Below are key hurdles businesses face when adopting customer analytics and ways to overcome them:
1. Data Quality and Consistency
Inaccurate data can distort insights and consequently lead to poor decision-making. Businesses should use data cleansing tools like Alteryx or Talend to maintain data integrity. Regular data audits and clear data governance policies are essential to maintaining data quality across platforms.
2. Integration of Data Sources
Data from diverse sources, such as in-store, online, and CRM systems, is often tedious. Customer Data Platforms (CDPs) can consolidate data into a unified customer view, increasing accuracy. API integrations streamline data flows, making it easier to gain comprehensive interpretations.
3. Customer Privacy and Data Security
Retailers must comply with privacy regulations such as GDPR and CCPA. Non-adherence can result in fines and loss of trust. This can be ensured through data encryption and the use of consent management platforms.
4. Unavailability of Skilled Human Resources
Retailers may need help finding good data analysts or data scientists. Analytics consulting firms like Tredence can help fill this gap, making sure that the tools and insights of customer analytics are used properly.
5. Resistance to Change
Employee or management resistance is another setback to analytics tools adoption. This can be overcome by laying a data-driven culture with training, effective communication, and early wins in clear visible benefits, increased sales or better customers' retention.
6. Real-time Data Processing
Real-time processing of enormous data volumes is quite challenging. Retailers use technologies like Apache, Kafka and AWS Kinesis to process and act on data in real-time, thus enhancing the online and in-store experience.
Thus, businesses can implement retail customer analytics successfully to make better data-informed decisions by settling the above challenges.
Let us conclude by discussing how such an implementation yields considerable results.
Driving Retail Growth with Tredence’s Customer Analytics
Retail customer analytics is crucial in understanding consumer behavior, enhancing personalization, and driving business growth. By utilizing accurate data and advanced analytics, businesses can improve customer experiences, streamline operations, and maintain a competitive edge in the market.
Implementing effective data quality and integration strategies allows companies to leverage customer insights fully.
As AI and machine learning evolve, businesses that adopt retail customer analytics are more likely to achieve long-term success, fostering customer loyalty and anticipating future demands.
With Tredence’s retail analytics solutions, you can improve your inventory planning and management. Leverage the power of AI-driven insights for accurate demand forecasting. Connect with Tredence today to elevate your retail strategy.
FAQs
1. How can retailers use customer data to improve their inventory management?
By analyzing past purchase patterns and external factors, retailers can leverage retail customer insights to predict customer demand.
2. What tools should retailers use to gather actionable retail customer insights?
Key tools for collecting and analyzing retail customer insights include Customer Relationship Management (CRM) systems, predictive analytics software, and Customer Data Platforms (CDPs). Platforms like Salesforce, IBM Watson, and Segment help retailers consolidate customer data from various sources to drive more personalized marketing and optimize sales strategies.
3. How can customer segmentation lead to better retail customer insights?
Segmenting customers into specific groups based on behaviors, preferences, and demographics enables retailers to gain more accurate and targeted insights. By using segmentation techniques, businesses can craft personalized marketing strategies, predict customer behaviors more accurately, and increase customer loyalty.
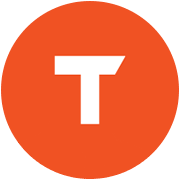
AUTHOR - FOLLOW
Editorial Team
Tredence