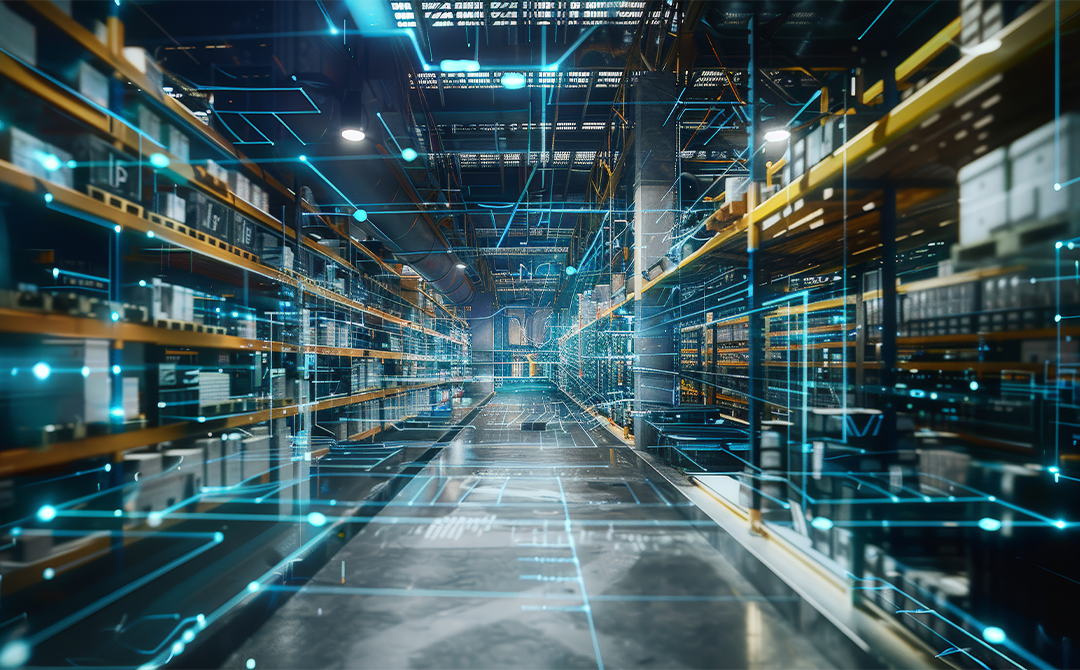
As data generation accelerates across all sectors, the retail industry stands out as one of the biggest contributors. Every interaction—from online browsing and loyalty programs to in-store purchases—creates vast data, resulting in an ecosystem that grows more complex daily.
Yet, as this data grows, so do the challenges of handling it. Traditional systems often struggle to keep pace, creating bottlenecks that limit how effectively an enterprise can tap into its data’s potential. Companies using data-driven B2B sales-growth engines report above-market growth, and EBITDA increases by 15 to 25 percent. (Source: McKinsey)
Relying on outdated systems can hinder retailers' efforts to make timely, data-driven decisions. They need a way to streamline data flow, make it accessible across teams, and unlock real-time insights seamlessly.
This is where data modernization comes into play.
“Data modernization is a multi-step process of transforming access to data to radically improve business intelligence and decision-making. Organizations modernize by shedding data silos and the complexity of legacy systems in favor of a cloud operational model across edge to cloud.” ~ Hewlett Packard
In the sections ahead, we’ll explore why retail data modernization is crucial and examine how outdated systems limit growth and insights. We’ll cover the core elements—retail data migration, integration, and governance—that enable a 360° customer view.
Alongside the benefits, we’ll consider the challenges, like data silos and privacy, and how emerging trends like AI make modernization a strategic must-have in today’s competitive retail landscape.
The Need for Retail Data Modernization
Retail data modernization is the transformation of a retailer's data systems from fragmented, legacy infrastructures to unified, agile platforms. Unlike other industries, retail relies on immediate data processing to understand and respond to customer behaviors, which fluctuate with trends, seasons, and shopping patterns.
Reliance on outdated systems creates serious inefficiencies in the retail industry. Crucial insights—like demand signals, shelf availability, and seasonal purchasing patterns—aren’t processed in real time, leading to disconnected decision-making.
Modernized data in retail unifies previously isolated sources, allowing deep customer insights by connecting online, in-store, and social data. This integration helps retailers predict demand, refine inventory, and deliver personalized experiences that enhance loyalty and responsiveness.
Data Modernization: The Enterprise Journey to 360° Customer View
A 360° customer view is a comprehensive, unified profile of a customer that integrates data from every interaction with a brand. It consolidates details from various sources—such as purchase history, browsing behavior, loyalty programs, customer service interactions, and even social media engagements—into a single, accessible profile.
For example, imagine a customer who provides his name as "John Smith" during in-store purchases but uses "J Smith" for online shopping.
In traditional legacy systems, these entries may be stored as separate customers due to minor inconsistencies in data entry. This disconnect could mean the retailer cannot track John's complete journey and preferences.
With 360° customer view, particularly through AI-driven enterprise customer data platforms, these discrepancies can be resolved by automatically identifying and merging duplicate records, creating a unified, accurate customer profile.
Now, John’s in-store and online purchases are connected, providing customer service reps and marketing teams with a clear view of his preferences and shopping patterns. This unified view allows the brand to tailor promotions, address service needs more effectively, and deliver a more cohesive, personalized experience across all touchpoints.
The Path to Achieving a True 360° Customer View for Enterprises
To achieve a robust, data-driven retail ecosystem, organizations must modernize their data management processes across three primary areas: data migration, integration, and retail data governance.
Retail Data Migration
Data migration involves transferring data from outdated, siloed legacy databases to modern cloud-based platforms. This transition is crucial as it allows retailers to utilize advanced retail analytics and business intelligence tools to process large volumes of structured and unstructured data more efficiently.
Key Steps in Data Migration
- Assessment of Current Data Landscape: Before migration, retailers must evaluate their existing data systems to identify which data is most valuable and frequently used. This assessment helps prioritize what needs to be migrated first.
- Planning the Migration Strategy: A well-defined migration strategy should include:
- Data Mapping: Understanding how existing data will fit into the new architecture.
- Choosing the Right Tools: Selecting appropriate migration tools that facilitate smooth transitions without data loss.
- Impact Analysis: Assessing dependencies and potential impacts on operations during migration.
- Execution of Migration: This phase involves extracting data from legacy systems, transforming it to meet new standards, and loading it into modern databases. It is essential to ensure that data integrity is maintained throughout this process.
- Post-Migration Validation: After migration, retailers need to validate the accuracy and completeness of the migrated data through reconciliation processes. This step is critical to ensure no valuable information is lost during the transition.
Integrating Data
Data integration combines information from various sources—such as CRM systems, ERP systems, supply chain databases, and external APIs—to create a unified view of customer interactions. This integration is vital for enabling comprehensive analysis and reporting, which is essential for informed decision-making.
Steps for Effective Data Integration
- Identifying Data Sources: Retailers should identify all relevant internal and external data sources contributing to customer insights. This includes transactional data, customer feedback, and social media interactions.
- Implementing Integration Tools: Utilizing modern integration platforms can facilitate seamless connections between disparate systems. These tools help automate data flows and ensure consistency across various datasets.
- Data Cleansing and Quality Assurance: Before integrating, it is crucial to cleanse the data by removing duplicates, correcting errors, and standardizing formats. High-quality data is essential for generating reliable insights.
- Creating a Unified Data Repository: Establishing a centralized data warehouse or lake allows easier access to integrated data. This repository serves as a single source of truth for analytics and reporting purposes.
Retail Data Governance
Data governance is the framework that ensures the quality, security, and compliance of data within an organization. It plays a critical role in managing how data is accessed, used, and maintained throughout its lifecycle.
Key Components of Retail Data Governance
- Defining Roles and Responsibilities: Clear definitions of who owns what data and who has access are crucial for effective governance. Establishing a governance team can help oversee these responsibilities.
- Implementing Policies and Standards: Organizations should develop policies regarding data usage, privacy compliance (such as GDPR), and security measures (like encryption and access controls). These policies should guide employees in handling sensitive information responsibly.
- Monitoring Data Usage: Continuous monitoring helps ensure compliance with established policies while identifying unauthorized access or anomalies in data usage patterns.
- Fostering a Culture of Data Literacy: Educating employees about the importance of data governance fosters a culture where everyone understands their role in maintaining data integrity and security. Training programs can enhance skills related to analytics tools, compliance requirements, and best practices in data management.
Key Challenges & Considerations for Retail Data Modernization
Data modernization in retail faces significant challenges, such as managing data quality during migration from legacy systems to cloud-based platforms. This transition requires meticulous planning to prevent data loss and ensure accuracy.
Another major consideration is data integration across diverse sources, including CRM, ERP, and external APIs, which can lead to compatibility issues. Retailers must choose tools that enable seamless integration to build a unified data view.
Security and compliance also pose critical challenges. With large volumes of sensitive customer data, retailers need robust governance frameworks to meet regulatory requirements like GDPR and protect data integrity.
The Convergence of Data Modernization and AI
The advancements in AI have become foundational to retail data modernization, allowing retailers to maximize the value they derive from data across all touchpoints. For instance, according to a recent survey, 64% of large retailers plan to increase investments in AI infrastructure over the next 18 months, reflecting the industry’s recognition of AI’s transformative potential in operational efficiency and customer engagement. (Source: NVIDIA)
McKinsey reports that IoT and robotics are now being deployed in manufacturing and retail operations to track products, streamline logistics, and even support in-store experiences like personalized promotions and automated checkouts.
A well-executed, expert-led migration minimizes delays and ensures data quality and system alignment, so retailers can swiftly move from siloed to integrated data insights.
By expediting this process, retailers can access and act on a complete customer view sooner, driving more immediate personalization, engagement, and loyalty-building initiatives.
Connect with us for highly customized retail data analytics solutions that will fast-track your migration journey and bring a unified customer view within close reach.
FAQs
- What are the key challenges for data migration in retail?
Key challenges in retail data migration include maintaining data integrity and compatibility between legacy and new systems and ensuring compliance with data security regulations. Successful migration also requires careful planning to avoid operational disruptions and provide accurate, consistent data across all platforms. - What is an enterprise customer data platform, and how does it benefit retailers?
An enterprise customer data platform (CDP) centralizes customer data from multiple sources into a single profile, giving retailers a complete view of each customer. This enables personalized marketing, omnichannel experiences, and better customer engagement, ultimately improving satisfaction and loyalty. - Why is retail data analytics important for modern businesses?
Retail data analytics provides insights that guide inventory management, pricing, and personalized marketing, helping retailers adapt quickly to customer behaviors and market changes. It is a critical driver of competitive advantage, enabling data-driven decisions that increase efficiency and profitability.
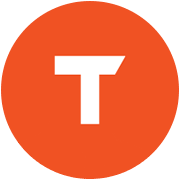
AUTHOR - FOLLOW
Editorial Team
Tredence
Next Topic
Customer Market Segmentation: A Key to Successful Targeted Marketing Campaigns
Next Topic