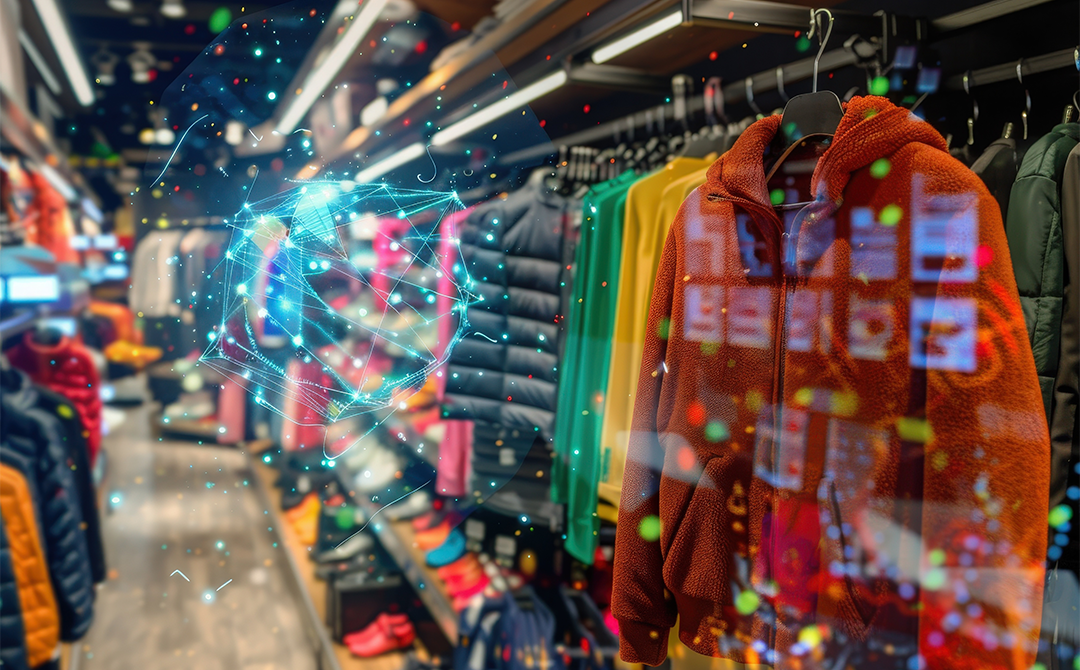
Imagine walking into a retail store where every shelf is tailored to your preferences and every interaction feels personal. Such precision is not a distant dream; it is a reality modern businesses are achieving through retail analytics.
Retail analytics enables businesses to identify customer preferences, optimize operations, and maximize profitability. The lack of it presents challenges for businesses. They struggle to understand evolving consumer behavior, leading to ineffective marketing campaigns, unsold inventory, and missed revenue opportunities.
According to customer loyalty expert Fred Reichheld, a 5 percent improvement in customer retention yields between 20 percent and 100 percent increase in profits across a wide range of industries. Without data-driven insights, businesses struggle to understand their customer base, risking churn and stagnation (Source: Wasp Barcode).
Retail analytics equips businesses with actionable insights to make informed decisions. It allows companies to anticipate trends, personalize customer experiences, and streamline operations. The result? Enhanced customer satisfaction, increased profitability, and the agility to adapt to ever-changing market dynamics.
This article explores the fundamentals of retail analytics, its evolution, and how it empowers businesses to thrive in today’s dynamic market.
What is Retail Analytics?
Retail analytics refers to the usage of data-driven insights to optimize retail operations. It includes analyzing customer behavior, enhancing inventory management, forecasting sales trends, and improving operational efficiency. This approach helps retailers make informed decisions that boost performance and profitability.
By leveraging technologies such as Artificial Intelligence (AI) and Machine Learning (ML), retail analysis empowers businesses to deliver better customer experiences, increase profitability, and stay competitive.
The world's largest retailer, Walmart transformed its business by adopting a data-driven approach and leveraging big data analytics, the company optimized its supply chain and implemented real-time inventory tracking.
Walmart uses predictive analytics to anticipate demand spikes and minimize stockout and overstocking issues (source: Walmart)
Modern businesses face fierce competition, ever-evolving customer preferences, and increasing market complexities. Without tapping into retail data insights, companies risk making decisions based on intuition rather than insights, leading to missed opportunities, inventory mismanagement, and poor customer experiences.
For example:
- Without retail analytics, retailers may fail to stock trending items, resulting in revenue losses.
- With analytics, they can predict trends, personalize marketing efforts, and ensure optimal product availability, driving higher sales and customer loyalty.
91 percent of business leaders agree that data analysis is key to business strategies. This highlights the value of analytics in customer-facing businesses.
As businesses use analytics for retailers for a competitive edge, it is important to understand its foundations, from simple sales tracking to an advanced system that guides decision-making for leaders in the retail industry (Source: Google).
Evolution of Retail Analytics
Retail analytics has evolved significantly over the past few decades. Initially focused on manually tracking sales and inventory—a process prone to errors and inefficiencies—it has advanced with the advent of point-of-sale (POS) systems, enabling retailers to efficiently capture and store transactional data.
Digital Shift
The rise of e-commerce in the 1990s and early 2000s marked a turning point. Retailers began collecting vast digital customer data, including browsing behaviors, purchase histories, and preferences.
Google Analytics and Customer Relationship Management (CRM) systems became essential for analyzing this data and tailoring marketing efforts.
Big Data Era
The 2010s ushered in the era of big data and advanced analytics. With technologies like cloud computing, ML, and AI, retailers could analyze enormous datasets in real time. Amazon pioneered predictive analytics to anticipate customer needs, automate inventory replenishment, and optimize pricing strategies.
Modern Landscape
Today, retail analytics encompasses omnichannel data integration, leveraging in-store and online data to provide seamless customer experiences. AI-driven personalization, supply chain optimization, and real-time insights have become standard practices among leading retailers.
Target’s Predictive Analytics
Predictive analytics leverages historical data, statistical algorithms, and machine learning to identify patterns and forecast future trends or behaviors. It helps organizations anticipate customer needs, improve decision-making, and optimize marketing, risk management, and supply chain efficiency operations.
Target, one of America’s retail giants, showcases the transformative potential of modern retail analytics. By leveraging predictive analytics to analyze customer purchase patterns, Target anticipates shopper needs with remarkable accuracy. This insight enables the company to deliver highly personalized marketing campaigns, increasing customer engagement and significantly boosting sales.
The transformation of retail analytics over the decades paints a vivid picture of progress—from rudimentary data collection to dynamic, AI-driven insights. But what powers this evolution, and what components make up this thriving ecosystem? To fully grasp its impact, let us break down the key building blocks that define retail analytics today (Source: Driveresearch).
Key Components of Retail Analytics
Retail analytics relies on several interconnected components that enable businesses to unlock valuable insights and drive decision-making. Each plays a distinct role in transforming raw data into actionable strategies.
1. Data Collection and Integration
The foundation of retail analytics lies in the collection of data from diverse sources, including:
- In-store systems such as POS terminals and inventory management software.
- Online platforms, including e-commerce sites, mobile apps, and social media.
- IoT devices such as smart shelves and in-store cameras.
- Third-party data sources include demographic databases, location-based information, and market trends from external providers.
Seamless integration across these channels ensures a unified view of customer behavior and operational metrics.
2. Data Warehousing and Management
Raw data needs to be stored and organized effectively. Modern retailers use cloud-based data warehouses such as Snowflake and Amazon Redshift to centralize massive datasets. These platforms ensure scalability, security, and quick access to critical data.
3. Advanced Analytics and ML
Advanced analytics tools identify patterns and trends from vast datasets. ML models predict customer behavior, optimize pricing strategies, and automate supply chain operations.
4. Visualization and Reporting
Insights become actionable only when presented clearly. Tools such as Tableau and Power BI enable retailers to create interactive dashboards, helping teams monitor KPIs such as sales performance, customer acquisition costs, and inventory turnover.
5. Real-time Insights
Retailers are increasingly leveraging real-time data processing to respond swiftly to market trends. Stream processing tools like Apache Kafka allow businesses to adjust pricing, promotions, and stock levels instantly.
6. Customer Segmentation and Personalization
Retail analytics leverages customer segmentation to target specific groups with tailored messaging. Personalization engines use analytics to recommend products, thus improving customer satisfaction and boosting sales.
By understanding and leveraging these components, businesses can create a comprehensive analytics strategy that enhances operational efficiency, improves customer experiences, and fuels growth.
Amazon’s recommendation engine generates 35 percent of its revenue by providing highly personalized product suggestions. It implements retail analytics to browse the history and purchase behavior of customers (Source: evdelo).
These components lay the groundwork for retail analytics. However, the true power of retail analytics emerges when applied to address specific business challenges.
What benefits do retailers gain when these elements work in harmony? How does this translate into tangible growth, enhanced decision-making, and operational excellence? Let us delve into the advantages of implementing retail analytics.
Benefits of Implementing Retail Analytics
Success in a highly competitive market depends upon agility, precision, and foresight. Analytics for retailers empowers businesses to achieve all three, delivering measurable outcomes across multiple dimensions. Here are the key benefits of retail analytics:
1. Enhanced Customer Experience
Personalization has become the holy grail of customer experience. Retail analytics provides deep insights into consumer behavior, enabling tailored experiences that increase satisfaction and drive loyalty.
2. Optimized Inventory Management
Retail analytics provides predictive insights into demand trends, ensuring inventory is optimized, and operations remain efficient.
3. Improved Pricing Strategies
Price is often the tipping point between conversion and abandonment. Advanced analytics enables dynamic pricing, allowing retailers to adjust prices based on demand elasticity, competitor prices, and customer behavior.
4. Streamlined Operations
Analytics uncovers inefficiencies across supply chains, staffing, and energy consumption. Retailers can streamline their operations to cut costs and improve sustainability, all while enhancing their bottom line.
5. Informed Decision Making
With data-driven decision-making, retail leaders can act on actionable insights rather than instincts. Analytics clarifies everything, from sales performance to supply chain bottlenecks, helping businesses make swift, informed choices.
6. Competitive Advantage
By leveraging analytics, retailers can better understand emerging trends, consumer behaviors, and market dynamics. This provides them with a distinct competitive edge.
Implementing retail analytics has tangible benefits beyond operational improvements. It creates a customer-centric, agile business model capable of responding to market dynamics in real time.
However, retailers often face obstacles that can hinder the full realization of analytics benefits. Addressing these challenges head-on is critical to success.
Challenges in Retail Analytics and How to Overcome Them
Retail analytics offers immense opportunities, but implementing it successfully has its share of challenges. Given below are some of the common obstacles retailers face, along with strategies to tackle them:
1. Data Quality and Consistency
Retailers rely on diverse data sources. However, poor data quality can lead to flawed insights, undermining decision-making. This inconsistency often results from siloed systems or inaccurate data inputs.
Retailers can address this by investing in data governance frameworks that ensure accuracy, consistency, and completeness.
Data validation protocols, real-time monitoring, and automated tools to clean and standardize data can significantly improve quality. Leveraging AI and ML can also help identify anomalies and improve data accuracy over time.
2. Integration of Legacy Systems
Retailers often rely on outdated legacy systems not designed for modern analytics, creating integration issues when consolidating data into advanced analytics platforms. This fragmented infrastructure hampers the ability to derive meaningful insights.
Retailers should integrate new analytics tools with legacy systems using middleware or API layers, enabling data to flow seamlessly between old and new systems. Investing in cloud-based platforms such as AWS, which offer flexibility and scalability, can ease integration while reducing operational costs.
3. Shortage of Skilled Workforce
Demand for skilled data scientists and analysts in retail is outpacing supply. According to ZDNet, a global shortage of data talent is hampering retailers' ability to fully leverage advanced analytics (Source: ZDNet).
Retailers should invest in employee training programs to upskill current staff in data analysis and data science or partner with universities and tech boot camps to bridge the talent gap. Additionally, using no-code or low-code analytics platforms can empower non-technical staff to engage with data more effectively, thus reducing the reliance on specialized professionals.
4. Data Security And Privacy Risks
Increase in the amount of personal data collected by retailers, especially in the wake of stricter data privacy regulations such as GDPR, poses a significant challenge. Failure to protect sensitive customer information can lead to fines, reputational damage, and loss of customer trust.
Retailers must prioritize data security by implementing robust encryption techniques, conducting regular audits, and educating employees about the best security practices. A transparent data-use policy and customer consent mechanism can help mitigate privacy concerns.
5. Resistance to Change
Change management is often the most difficult challenge in any digital transformation. Employees may be reluctant to adopt new tools, especially if they do not see immediate benefits or fear job displacement.
Building a culture of data-driven decision-making is crucial. Retailers should educate employees on the value of analytics in improving business outcomes. Leadership should actively promote analytics success stories within the company and provide hands-on training to build confidence.
Retailers that recognize and address these challenges head-on can unlock the full potential of their data, resulting in better decision-making, improved customer experiences, and enhanced operational efficiency.
Case Studies of Successful Retail Analytics Implementation
Retail analytics has proven invaluable for businesses seeking to optimize operations, improve customer satisfaction, and drive profitability. Some examples of how retail analytics has transformed leading companies include:
1. Walmart
Walmart, the world’s largest retailer, uses predictive analytics to optimize store inventory. The company can predict demand fluctuations by leveraging data from its vast network of locations, reducing stockouts and overstocking.
Walmart's predictive models analyze various factors, such as weather, regional events, and local customer behavior. The company reduced out-of-stock instances by 10 percent to 15 percent, significantly improving customer satisfaction.
It also reported a $1 billion cost reduction through data-driven inventory management (Source: ProjectPro).
2. Sephora
Sephora's Beauty Insider program, powered by retail analytics, provides personalized recommendations that boost customer engagement and sales. The company analyzes vast customer data to offer tailored promotions and experiences.
This approach helped Sephora increase customer loyalty, with Beauty Insider membership accounting for over 80 percent of total sales. Furthermore, Sephora’s data-driven insights help it predict product demand precisely, optimize inventory and reduce waste (Source: Renascence).
3. Amazon
The e-commerce giant collects vast amounts of data from user behavior to refine its pricing models and personalize recommendations. Due to its highly personalized customer recommendations, Amazon increased its sales conversion rate by up to 30 percent through advanced algorithms and ML.
Amazon's ability to optimize its supply chain with predictive analytics contributes to its operational efficiency, reducing delivery times and lowering costs (Source: Rejoiner).
These case studies indicate the critical role of retail analysis in various industries. Companies that invest in robust analytic capabilities experience a competitive edge by improving efficiency, making informed decisions, and enhancing customer experiences.
Future Trends in Retail Analytics
The retail industry is entering a new era where data-driven strategies are more crucial than ever. Retailers who embrace emerging technologies and stay ahead of trends will be poised for success in an increasingly competitive marketplace.
Here are the key trends that are shaping the future of retail analytics:
AI- and ML-driven Insights
AI and ML are transforming retail analytics in the CGP industry by unlocking deeper insights from vast data sets. These technologies enable retailers to predict trends, personalize customer experiences, and optimize inventory in real time.
AI's ability to analyze patterns and forecast demand more accurately will reduce costs and improve operational efficiency.
Real-time Analytics
Real-time data is becoming a vital tool for decision-making in retail. With the rise of IoT devices and connected systems, retailers can now analyze data as it happens, enabling immediate responses to customer behaviors and market changes.
This capability is critical for adapting to shifting trends, such as inventory adjustments or personalized promotions.
Augmented Reality (AR) and Virtual Reality (VR)
IKEA and Nike leverage AR and VR to enhance customers’ shopping experience. These technologies improve engagement and provide valuable data on consumer preferences and behavior.
Retailers are using AR and VR to offer virtual try-ons, allowing them to capture data on customer interactions and refine marketing strategies (Source: Linvelo).
Voice Commerce
Retailers are capturing data from voice searches and commands to improve the shopping experience. Walmart has been developing and utilizing Conversational AI assistants to enhance the shopping experience and save customers’ time.
This data allows for better-targeted promotions, optimized product listings, and seamless transactions, ensuring customers can easily access products through voice-enabled devices (Source: Walmart).
Hyper-personalization
AI-driven hyper-personalization is taking customer engagement to the next level. Retailers use data from various sources, such as browsing behavior, purchase history, and social media activity, to create highly tailored offers.
This level of personalization fosters stronger customer loyalty and drives higher sales.
Blockchain for transparency
Blockchain is gaining traction to enhance transparency and security in the retail supply chain. By providing an immutable ledger of product origins and transactions, blockchain allows customers to verify product authenticity, ensuring higher trust. This also helps retailers manage compliance and reduce fraud.
The future of retail analytics is focused on harnessing advanced technologies to deliver real-time, personalized, and data-driven experiences.
Let's explore the best practices that retailers can implement to capitalize on these innovations and stay ahead of the competition.
Best Practices for Retailers in Retail Analytics
Implementing retail analytics effectively requires more than just collecting data; it is about leveraging insights to drive strategic decisions. Retailers need to follow best practices that enhance their analytical capabilities and align with their business goals.
Here are the best practices that will help retailers harness the full potential of retail analytics:
1. Clearly Define Objectives
Before diving into retail analysis, it is crucial to define specific business objectives. Retailers must understand what they want to achieve, whether improving inventory management, increasing customer loyalty or optimizing marketing efforts.
By setting clear goals, analytics efforts become focused, measurable, and aligned with overall business strategies.
2. Integrate Data Across Channels
Retailers must consolidate data from various touchpoints like in-store, e-commerce, and mobile platforms to get a complete view of customer behavior.
Omnichannel data integration enables more accurate insights into customer preferences, purchase patterns, and overall business performance. This integrated data approach drives informed decision-making across all customer interactions.
3. Leverage Predictive Analytics
Predictive analytics analyzes historical data to help retailers anticipate customer needs and market trends. Using predictive models, retailers can forecast demand, personalize customer experiences, and optimize pricing strategies. This foresight empowers retailers to stay ahead of market shifts and enhance operational efficiency.
4. Invest in real-time data analytics
Real-time analytics enables retailers to respond quickly to changes in customer behavior, inventory levels, or sales trends. By utilizing real-time data, businesses can instantly adjust marketing campaigns, pricing strategies, and product offerings.
5. Adopt Advanced ML and AI Techniques
AI and ML techniques can unlock deeper insights into customer data. By automating data analysis, retailers can uncover hidden patterns and trends that would be impossible to detect manually.
These technologies can drive advanced personalization, customer segmentation, and demand forecasting, providing retailers a competitive edge.
6. Prioritize Data Privacy and Security
Customer data has become a central component of retail analytics. Therefore, it is essential to prioritize data security and privacy.
Retailers must comply with regulations such as GDPR and CCPA and ensure customers' personal data is handled responsibly. Building trust through transparent data usage enhances customer loyalty and protects brand reputation.
7. Create a Data-driven Culture
Retailers should foster a culture where data-driven decision-making is embedded in every aspect of the business. This includes training employees on data tools, promoting data literacy, and ensuring leaders at all levels have access to the insights they need to make informed decisions.
8. Continuously Monitor And Optimize Analytics Efforts
Retail analytics is not a one-time initiative but an ongoing process. Retailers need to continuously monitor the effectiveness of their analytics programs and optimize them based on performance.
Regular reviews, testing new approaches, and integrating feedback ensure that analytics efforts align with business goals and market dynamics.
By adopting these best practices, retailers can better understand their customers, optimize operations, and make data-driven decisions.
The next section explores the tools and technologies available for retailers to implement and scale their analytics programs effectively.
Tools and Technologies for Retail Analytics
Retailers are increasingly using advanced tools and technologies to unlock the full potential of their data. The following key solutions can empower retailers to improve decision-making and optimize operations:
- Cloud-based Analytics Platforms: AWS, Google Cloud, and Microsoft Azure offer scalable solutions for real-time processing of large datasets. This facilitates advanced analytics for retailers and seamless integration with ML-driven solutions.
- Business Intelligence (BI) Tools: Tableau, Power BI, and Looker provide interactive dashboards and reports to track performance and customer trends, helping retailers make data-driven decisions quickly.
- Predictive Analytics and ML: Tools such as SAS, DataRobot, and Alteryx use historical data to predict future trends, optimize inventory, and personalize customer experiences, providing retailers with a competitive edge.
- CRM Systems: Platforms such as Salesforce and HubSpot enable retailers to analyze customer behavior, tailor marketing campaigns, and enhance customer relationships.
- Retail-specific Analytics Tools: Retail-focused tools like RetailNext analyze in-store performance, foot traffic, and inventory, providing retail data insights for operational optimization.
- E-commerce Analytics: Google Analytics and Adobe Analytics offer deep insights into online shopping behavior, helping retailers optimize user experiences and boost conversions.
- Inventory Management Systems: NetSuite and Quickbooks enable real-time inventory tracking and demand forecasting, reducing the risk of stockouts and overstocking.
By combining these technologies, retailers can gain valuable insights, enhance operational efficiency, and drive profitability.
Embracing Retail Analytics with Tredence
Integrating retail analytics into modern strategies is no longer optional—it is necessary for growth, innovation, and sustained success in an increasingly competitive market.
With businesses now more equipped than ever to convert vast amounts of data into actionable insights, the potential for transformation is immense. From improving operational efficiency and enhancing customer experiences to making data-driven decisions in real time, the opportunities are limitless.
Retailers must embrace advanced analytics tools and adapt to future trends such as AI and ML. These technologies are shaping the next frontier of retail, allowing businesses to stay agile, predictive, and customer-centric.
For executives looking to future-proof their businesses, leveraging retail analytics is the key to surviving and thriving in a rapidly evolving retail landscape.
Tredence understands that the journey toward data-driven retail analytics is complex and multifaceted. Its expertise in advanced analytics solutions empowers retailers to harness the full potential of their data and drive tangible business outcomes.
Contact Tredence today to enhance your retail analytics and stay ahead in the competitive retail landscape.
FAQs
- How can small to medium-sized retailers benefit from retail analytics with limited budgets?
Retailers with limited budgets can use cloud-based tools or platforms with pay-as-you-go models that scale according to requirements and demand instead of high fixed-cost or subscription models.
- What challenges do retailers face when implementing retail analytics, and how can they overcome them?
Common challenges include data silos, outdated systems, and a lack of skilled personnel. Retailers can address these by integrating centralized data platforms, modernizing legacy systems with cloud-based technologies, and investing in employee training or outsourcing expertise.
- How do retailers measure the ROI of retail analytics implementation?
ROI can be measured by tracking specific metrics such as sales, inventory turnover, customer satisfaction score, and cost savings in operations.
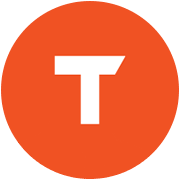
AUTHOR - FOLLOW
Editorial Team
Tredence