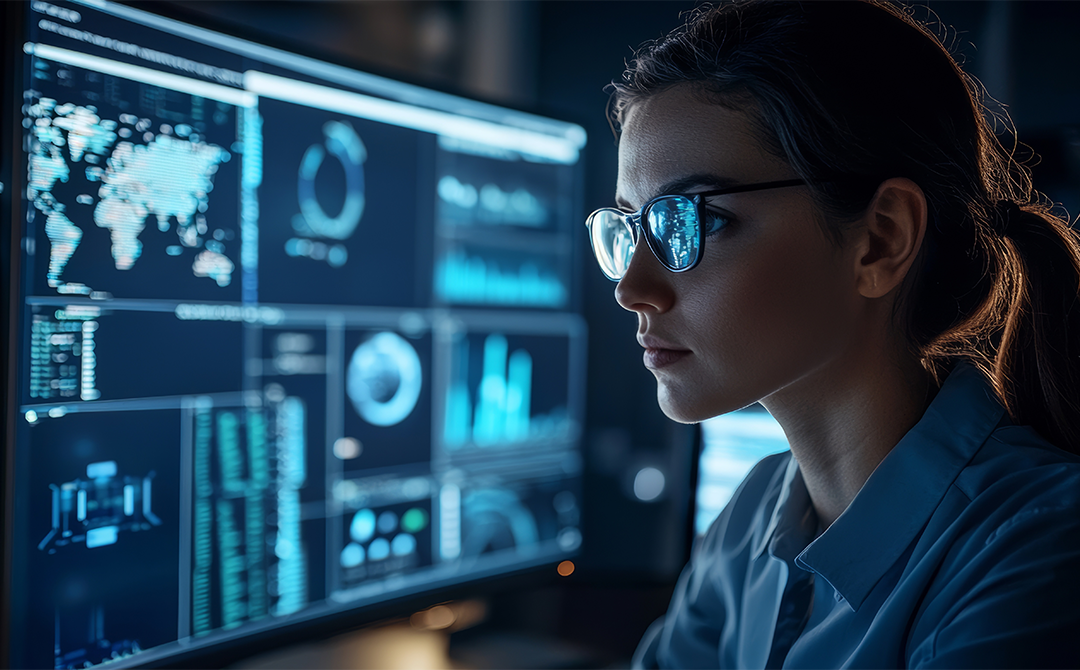
Introduction
The rapid growth of global e-commerce has transformed the retail industry, intensifying competition and raising customer expectations. To stay profitable and satisfy customers, retailers must ensure the right products are available at the right time, avoiding overstocking and empty shelves through effective inventory management. This is where predictive analytics in retail helps optimize inventory levels.
Retail inventories grew by 12% in the United States alone, reaching $740 billion in 2022 (Source: McKinsey & Company). Predictive analytics leverages data-driven insights, and retailers can improve inventory planning, ensuring optimal on-shelf availability and reducing costly out-of-stocks. By using demand forecasting, predictive analytics can align stock levels with customer demand to manage retail supply chains efficiently.
As retailers adopt predictive analytics, they can better navigate the complexities of inventory management, ultimately minimizing excess stock and associated holding costs. This article explores how predictive analytics can significantly optimize retail inventory costs while improving service levels and operational efficiency.
What is Retail Predictive Analytics?
Predictive analytics in retail uses historical and real-time data, machine learning, and statistical algorithms to predict future inventory trends accurately. Simply put, predictive analytics recommends accurate inventory planning based on data from retail operations.
By getting insights into past trends, customer buying patterns, sales figures, and market factors, retailers can make informed decisions on inventory levels, minimize carrying costs, and improve overall retail operations.
Instead of relying on multiple spreadsheets, data-driven inventory planning eliminates guesswork, determines optimal stock levels, and optimizes retail inventory to avoid excess stock and stockouts of essential products.
Macy’s uses predictive analytics in retail to ensure healthy stock levels, with only a 7% increase in inventory in Q2 of 2022. (Source: SupplyChainInDive)
The Cost of Poor Inventory Management
Poor inventory management leads to overstock or out of stocks, high holding costs, wasted resources, and negatively impacts the bottom line.
Inventory planning and management issues are critical challenges every retailer must address. Inefficient inventory management invites many problems that ultimately affect the bottom line. Overstock, out-of-stock, lack of real-time visibility, demand volatility, and high holding costs lead to wasted resources and tied-up capital.
For example, overstocking increases storage costs, and retailers may have to clear excess inventory at discounted prices. Conversely, stockouts result in costly emergency replenishments or customer losses to competitors. Ultimately, poor inventory management causes significant revenue loss and profit erosion.
According to a Harvard study, 72% of stockouts resulted from erroneous replenishing practices or inventory mismanagement. Further, around 30% of customers purchase the product from another store in understock situations, with abandoned sales resulting in a 4% revenue loss for retailers (Source: Harvard Business Review)
Additionally, inaccurate inventory management negatively impacts the efficiency of the entire retail supply chain, resulting in delays and increasing lead times.
How Predictive Analytics Reduces Retail Inventory Costs
Predictive analytics in retail addresses inventory management challenges by optimizing stock levels and keeping costs low. It enables accurate demand forecasting, efficient resource allocation, and improved supply chain efficiency. Here’s how predictive analytics can help reduce your inventory costs:
Improving Demand Forecasting
One way of using predictive analytics in retail is demand forecasting. Machine learning and statistical algorithms dissect insights from historical sales data, customer behavior patterns, market trends, and more to forecast demand accurately.
Predictive analytics helps retailers maintain inventory levels per market demand, eliminating the risks of overstock or stockout situations.
Learn how Tredence’s retail data analytics solution helped a top 10 retailer achieve demand forecasting with 600 basis point accuracy and a 6% improvement in inventory costs.
With accurate demand forecasting, retailers can boost customer satisfaction and reduce inventory management costs.
Optimizing Retail Inventory
By leveraging predictive analytics, retailers can maintain optimal stock levels through machine learning models that analyze real-time sales data, market trends and other key factors. This helps retailers adjust inventory in response to changing conditions, enabling them to meet seasonal demands and deliver the right product at the right time.
Hence, predictive analytics in retail supports precise inventory planning, reducing the risks associated with fluctuating market demands and enhancing overall inventory optimization.
Improving Supply Chain Management
Predictive analytics in retail improves retail supply chain management by providing accurate customer demand forecasts and efficient inventory management. Retailers can make informed inventory adjustments, optimally allocate resources, reduce lead times, enhance procurement cycles, and manage operations smoothly.
Walmart, for example, uses data and analytics to manage inventory levels and improve supply chain efficiency. It accurately predicts demand and ensures products are available to customers at the right time and price. (Source: SupplyChainDive)
Predictive analytics reduce inventory costs by aligning stock levels with customer demand and enhancing operational efficiency. By anticipating needs and minimizing excess inventory, retailers can avoid overstocking and stockouts, ensuring smoother operations. Next, let's explore how real-time insights play a pivotal role in maintaining on-shelf availability and boosting customer satisfaction.
Leveraging Predictive Analytics: Real-Time Insights and On-Shelf Availability
Predictive analytics in retail help with real-time inventory monitoring for on-shelf availability, minimizing out-of-stock situations. It also reduces the time spent on manually checking inventory levels.
Beyond inventory and supply chain management, retail inventory optimization delivers real-time insights into on-shelf availability, empowering retailers to make faster, data-driven decisions.
- Streamlining Real-Time Inventory Monitoring: With the help of IoT devices and sensors, machine learning and artificial models in predictive analytics provide real-time insights into on-shelf availability. This allows retailers to identify low-stock products and replenish them promptly and quickly.
- Reducing Out-of-Stock Situations: Out-of-stock scenarios directly impact customer satisfaction and business sales. Predictive analytics' demand forecasting capabilities enable accurate replenishments, allowing retailers to set up automated inventory orders to meet customer demand. This is especially crucial for high-demand products that tend to sell out quickly.
- Enhancing Chain Efficiency: Accurate demand forecasting and automated inventory ordering reduce the time retailers spend manually checking inventory levels. Predictive analytics in retail enhance overall chain efficiency, giving retailers more time to focus on strategies that enhance customer satisfaction.
Harnessing predictive analytics for real-time insights and on-shelf availability allows retailers to deliver a seamless shopping experience and reduce costs. This helps them enhance customer satisfaction and stay ahead of market demands.
The Future of Predictive Analytics in Retail Supply Chains
The future of predictive analytics in retail hinges on intelligent data utilization, the integration of advanced AI and ML models, and the provision of scalable solutions. By enhancing retail operations and personalizing customer experiences, predictive analytics will continue to transform the industry.
- Smarter, Data-Driven Inventory Strategies: With enhanced precision in machine learning models, predictive analytics will deliver granular insights into operations. Access to these deeper insights will empower retailers to refine their inventory strategies and better meet customer demands.
- Integrated AI and Machine Learning Models: The advent of Generative AI (GenAI) will further advance predictive analytics. For example, GenAI models can analyze turnover data and suggest fine-tuned remedies to improve stock efficiency. AI-empowered demand forecasting will enhance the accuracy and overall efficiency of inventory planning.
- Scalability and Flexibility for Retailers: Scalable predictive analytics is the future in retail. Such solutions can process increasing data volumes while maintaining high accuracy and performance. This scalability empowers retailers to adapt easily to market demands and ensures continuous improvement.
Predictive analytics in retail is not just about retail inventory optimization but also about keeping costs low and increasing profitability. It is a powerful tool for retailers to make data-driven decision-making on inventory planning and predictive perceptions, improving overall operational efficiency.
When we look at the future of predictive analytics in retail supply chains, its potential to transform operations is readily apparent. Retailers can use advanced data analytics to boost demand forecasting, inventory planning, and overall supply chain management. This proactive strategy not only reduces out-of-stocks but also ensures that products are available when and where customers need them, thereby increasing efficiency and profitability.
As technology advances, retailers must embrace predictive analytics to remain competitive in a fast-paced industry. The future seems promising for those who use these data to create a more responsive and agile retail environment.
Elevate your inventory planning and management with Tredence’s retail data analytics solutions. Explore how our AI-powered analytics support accurate demand forecasting, driving top-line growth and bottom-line savings. Talk to our experts today!
FAQS
How is predictive analytics used in retail?
Predictive analytics in retail helps with demand forecasting, inventory optimization, supply chain management, and predicting future demand patterns. It ensures the right product is available to the right customer at the right time and price, reducing holding costs and improving customer satisfaction.
What is the role of predictive analytics in inventory management?
Predictive analytics uses historical data, machine learning and statistical algorithms to predict future product demand. Retailers can make informed decisions on the right inventory levels, eliminating overstock or out-of-stock issues. They can also reduce holding costs and boost customer satisfaction.
How do predictive analytics enhance supply chain management?
Predictive analytics provides accurate demand forecasting and helps retailers automate inventory orders. It improves procurement cycles, optimizes resource allocation, reduces lead times and delays, and enhances overall supply chain management.
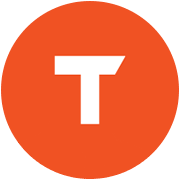
AUTHOR - FOLLOW
Editorial Team
Tredence
Topic Tags