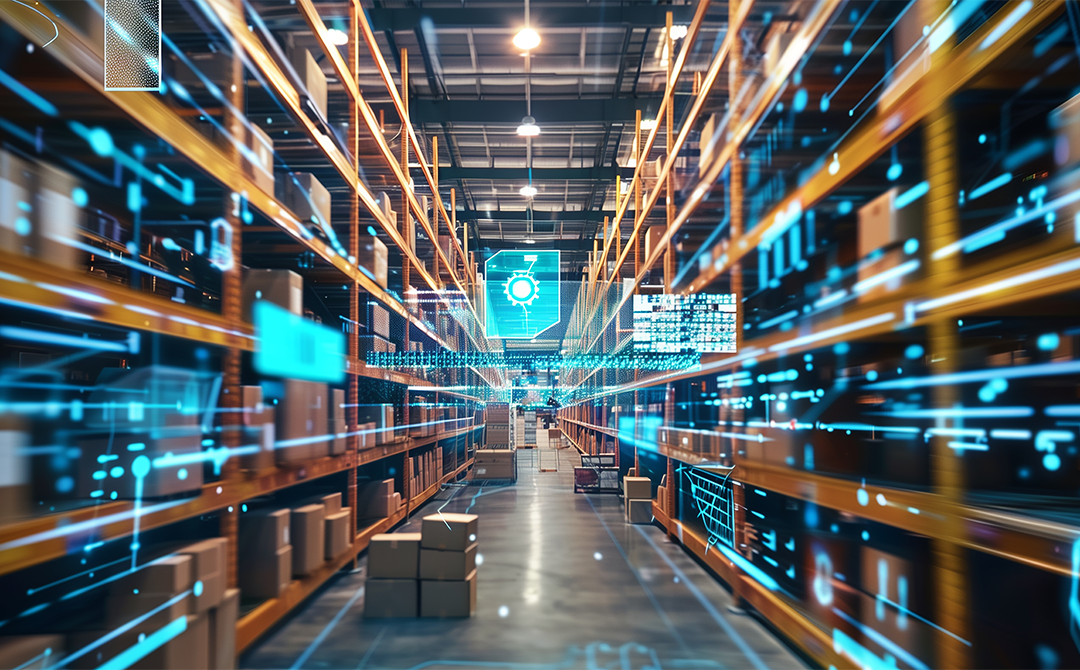
Silos are a problem. Their impact extends far beyond simply hindering information sharing between departments. These communication barriers result in conflicts, mistrust, and impaired decision-making. Consequently, teams often take inadequate or inaccurate actions, potentially alienating customers and external stakeholders. Such issues have inflict substantial financial and reputational damage on businesses.
The issues are equally pronounced for an organization’s supply chain, establishing a clear case for a single supply chain management platform that replaces disparate applications across the chain and harnesses ecosystem-wide data for common organizational understanding and effective decision-making. Otherwise known as a Supply Chain Command Center (SCCC) or Supply Chain Control Tower (SCCT), the unified platform should bring key capabilities to help convert your supply chain into a competitive center that drives market leadership. These include:
- State-of-the-art AI and ML components to deliver a range of predictive and prescriptive analytics in real-time
- Automation of repeatable tasks to reduce errors and enhance efficiency and innovation
- Comprehensive, live visibility that provides a complete picture and a shared understanding to every team member
With this, you will have an AI-powered supply chain that will empower your teams to take targeted and timely action for supply chain success.
According to ABI Research, supply chain control tower revenues will surpass U.S. $10 million in 2025. Manufacturers, logistics service providers (LSPs), and retailers are among the highest adopters currently, with a 17%, 18%, and 15% share of revenues, respectively.
Source: SDCEXEC
This article looks at some key problems enterprises can solve with an AI-powered supply chain underpinned by a supply chain command center.
Collaborating for Outcome Orchestration
In a supply network, decisions made in one department impact others far along the chain. In the presence of data and application silos, most teams don’t get to interact with each other, and each may have a different idea of what tasks or metrics need to be prioritized. This lack of end-to-end orchestration ability can have a significant ripple effect. For instance, a retailer implementing an advanced inventory management system to improve sales may inadvertently cause a supply bottleneck at the distribution center. This could lead to more pressure on logistics operations, empty store shelves, and disappoint customers.
If the retailer had a supply chain control tower, the last-mile team would have known from their unified dashboards that a change in systems at their end would need them to alert other teams in advance. This would have prevented a series of disruptions and ensured all outlets were well stocked during the transition.
Real-Time Visibility and Analysis
In an AI-powered supply chain, you get real-time auto-updates as the raw materials and products move through different cogs in the supply chain. When the supply chain processes and their progress updates are manual, teams are more likely to miss proactively identifying risks and disruptions due to simple human error and because of a lack of time to sift through the delayed and voluminous inputs.
On the other hand, when a supply chain command center replaces manual processes and updates with automated real-time equivalents and couples this with ongoing, cutting-edge analytics of unsiloed data, issues will be flagged before they arise, and corrective actions suggested, ensuring that all responsiveness is data-driven and hits the mark.
Limited AI Capabilities
Imagine a supply chain management solution without AI capabilities. It may unify disparate data sources but will have to overlook unstructured or image data or use up far more time and computing power to ingest such variables.
While it may rapidly run analytics to glean insights from large datasets, the insights delivered, already limited by the data constraints, and basic and static in nature, such as averages and failure rates, are not effective in the current environment of complex volatility.
This makes it essential to embed AI and ML algorithms that provide nuanced prescriptive and predictive intelligence into your supply chain for dynamic optimization. Let us examine a few wins your company will have with such a command center.
Transportation and Distribution Cost Escalation
An area that an AI-powered supply chain effectively optimizes is transportation and distribution costs. It does this by analyzing several variables like fuel costs, demand patterns, delivery routes, vehicle load optimization, traffic patterns, and the latest news reports of extreme weather events. In the absence of AI, the last piece of information would not be tapped into fast enough, leading to suboptimal planning.
Inventory Management Challenges
Consider an online fashion retailer using the AI-powered supply chain to predict inventory based on trends in customer preferences on social media.
In addition, the company will leverage advanced analytics and machine learning to mitigate inventory management challenges and fulfill orders from demanding customers.
- Demand forecasting will use historical sales data, customer behavior, market trends, and other external factors
- Stock-level optimization algorithms analyze supplier performance, seasonal requirements, lead times, and demand patterns
- Automated replenishment predicts when inventory runs low and triggers orders before stockouts.
Bringing together these powerful, highly calibrated techniques that tap into diverse data that would have been impossible in the absence of AI capabilities will help the fashion retailer thrive in a fickle and unpredictable industry.
Inefficient Warehouse Labor Planning
Supply chain disruptions are also caused by improper warehouse labor planning. Warehouses are fast-changing environments with hidden influencing factors and hard-to-discern patterns. Traditional analytics struggles to bring together demand estimation, equipment failure, employee absence, and many more variables. Advanced AI and ML models, however, are made for this very purpose and will equip warehouse managers with optimal suggestions within minutes on hiring and deploying teams or making new schedules for the next few hours or days.
Risk Assessment
Traditionally, evaluating supplier performance, measuring lead times, and ensuring suppliers comply with local and international regulations are done manually. It is time-consuming and prone to errors. This slows down procurement and leaves you unprepared for costly disruptions.
Let’s take the case of a pharmaceutical company where identifying and onboarding suppliers is more rigorous than in other industries. With the help of AI, it tracks geopolitical developments that can affect the supply of raw materials. The AI-powered supply chain will trigger a risk alert if it detects instability in a region with a major supplier. The system will rapidly come up with recommendations for other vetted suppliers, preventing production delays of important, life-saving medication. Such capabilities empower enterprises to de-risk their supply chain via informed data-driven decisions, significantly reducing any related financial impact.
Wrapping Up
In end-to-end supply chain management, introducing an AI-powered supply chain control tower reduces several issues prevalent in traditional methods. It bridges disconnected applications, enhances outcome orchestration, and ensures seamless coordination throughout the entire supply chain. With AI, you can better your operational performance and quickly adapt to fluctuating market conditions.
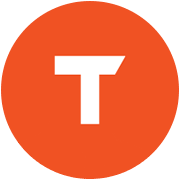
AUTHOR - FOLLOW
Editorial Team
Tredence
Topic Tags